EP-Pred: A Machine Learning Tool for Bioprospecting Promiscuous Ester Hydrolases
BIOMOLECULES(2022)
摘要
When bioprospecting for novel industrial enzymes, substrate promiscuity is a desirable property that increases the reusability of the enzyme. Among industrial enzymes, ester hydrolases have great relevance for which the demand has not ceased to increase. However, the search for new substrate promiscuous ester hydrolases is not trivial since the mechanism behind this property is greatly influenced by the active site's structural and physicochemical characteristics. These characteristics must be computed from the 3D structure, which is rarely available and expensive to measure, hence the need for a method that can predict promiscuity from sequence alone. Here we report such a method called EP-pred, an ensemble binary classifier, that combines three machine learning algorithms: SVM, KNN, and a Linear model. EP-pred has been evaluated against the Lipase Engineering Database together with a hidden Markov approach leading to a final set of ten sequences predicted to encode promiscuous esterases. Experimental results confirmed the validity of our method since all ten proteins were found to exhibit a broad substrate ambiguity.
更多查看译文
关键词
biocatalysts,bioprospecting,esterases,lipases,hydrolases,machine learning,supervised learning
AI 理解论文
溯源树
样例
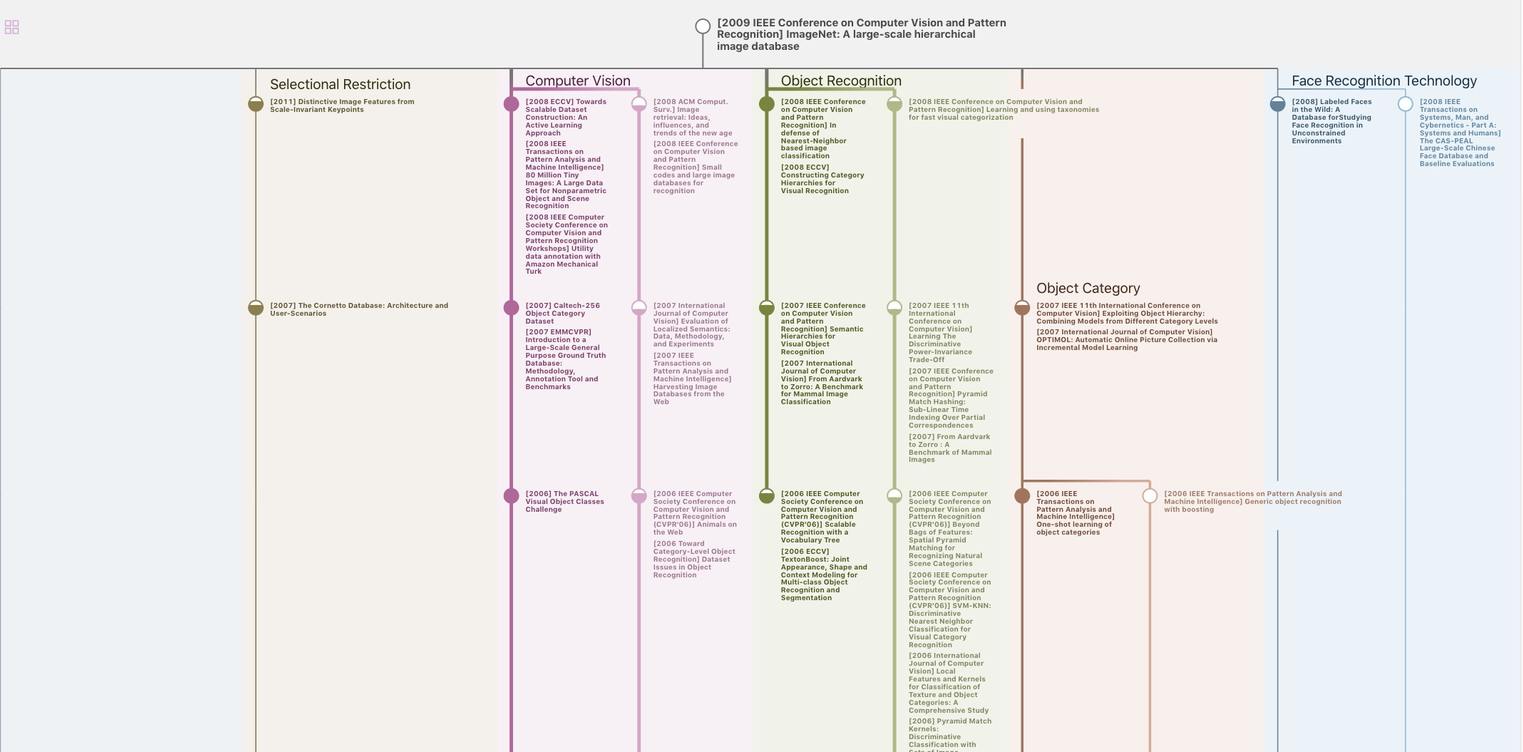
生成溯源树,研究论文发展脉络
Chat Paper
正在生成论文摘要