Multiple Instance Learning Based on Mol2vec Molecular Substructure Embeddings for Discovery of NDM-1 Inhibitors
Practical Applications of Computational Biology and Bioinformatics, 16th International Conference (PACBB 2022)(2022)
摘要
In this paper, we first present a new dataset of NDM-1 biological activities that is compiled by a cleaned version of the NMDI database. A literature review enriched the former database by 741 new compounds, comprising activities against NDM-1 classified in three classes (inactive, weakly and strongly active compounds) by specifying a unifying procedure for the labeling, which covers a range of different activity properties. Second, we restate the classification problem in the Multiple Instance Learning (MIL) setting by representing the compounds as a collection of Mol2vec vectors, each of them corresponding to a specific substructure (either atom or atom including their firsts neighbors). We observe an amelioration up to 45.7% and 38.47% in respect to balanced accuracy and F1-score, respectively, for the strongly active class in the MIL approach when compared to the classical Machine Learning paradigm. Finally, we present a classification and ranking framework based on classifiers learned by a k-fold CV procedure, which possess different hyper-parameters per fold, learnt by a Bayes optimization procedure. We observe that the top-3 and top-5 ranked accuracies of the strongly active classified compounds yield 100% for the MIL setting.
更多查看译文
关键词
Machine leaning, Multiple instance learning, Drug discovery, NDM-1 inhibitors
AI 理解论文
溯源树
样例
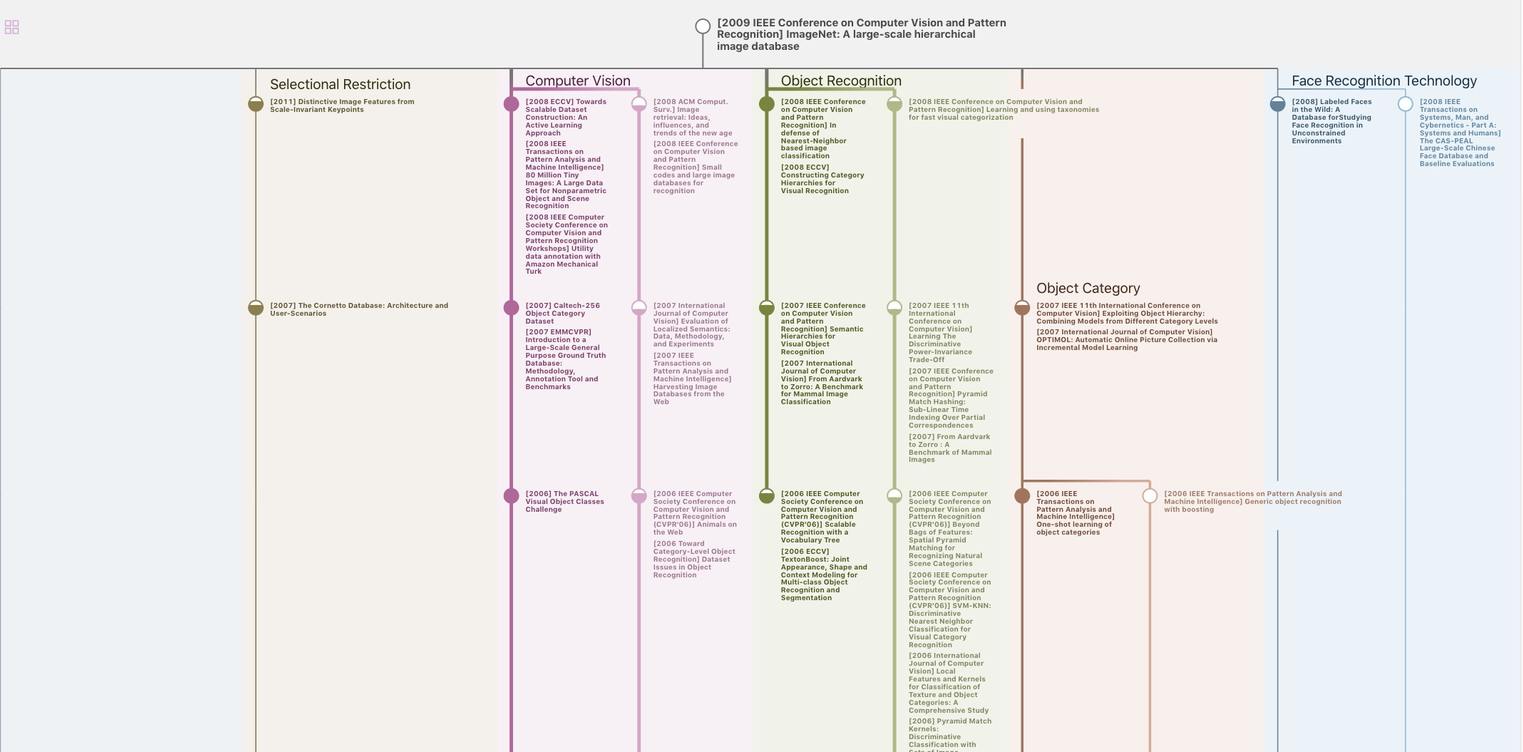
生成溯源树,研究论文发展脉络
Chat Paper
正在生成论文摘要