Ada-JSR: Sample Efficient Adaptive Joint Support Recovery From Extremely Compressed Measurement Vectors.
IEEE International Conference on Acoustics, Speech, and Signal Processing (ICASSP)(2022)
Abstract
This paper considers the problem of recovering the joint support (of size K) of a set of unknown sparse vectors in R-d, each of which can be sensed using a different measurement matrix. Such models have wide applicability ranging from communication to multi-task learning. We develop an adaptive strategy called Adaptive Joint Support Recovery (AdaJSR) that enables exact support recovery in the extreme compression regime with only m = 1 measurement per unknown vector while requiring a total complexity of no more than K left perpendicular log(2) p(d) right perpendicular measurements. Unlike existing support recovery techniques which require suitable assumptions on the correlation structure or distribution of the unknown signals in order to operate in the regime m < K, we show that the flexibility of adaptive measurement design alone allows us to operate in this extreme compression regime, without the need for imposing any correlation or sub-Gaussian priors.
MoreTranslated text
Key words
Joint Support Recovery,Multiple Measurement Vector,Adaptive Sensing,mmWave Channel Subspace Estimation,Multi-Task Learning
AI Read Science
Must-Reading Tree
Example
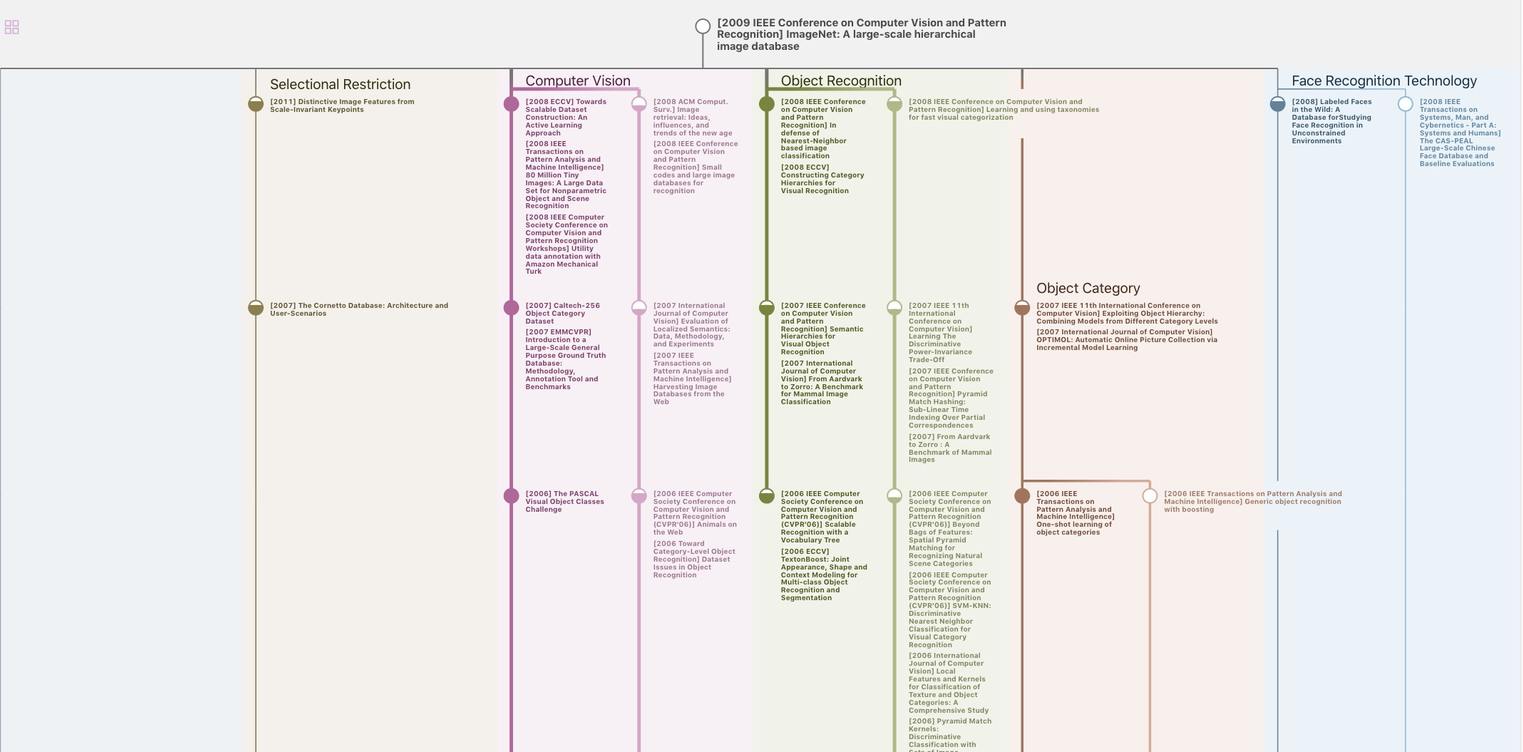
Generate MRT to find the research sequence of this paper
Chat Paper
Summary is being generated by the instructions you defined