Heterogeneous Graph Node Classification With Multi-Hops Relation Features.
IEEE International Conference on Acoustics, Speech, and Signal Processing (ICASSP)(2022)
摘要
In recent years, knowledge graph (KG) has obtained many achievements in both research and industrial fields. However, most KG algorithms consider node embedding with only structure and node features, but not relation features. In this paper, we propose a novel Heterogeneous Attention (HAT) algorithm and use both node-based and path-based attention mechanisms to learn various types of nodes and edges on the KG. To better capture representations, multi-hop relation features are involved to generate edge embeddings and help the model obtain more semantic information. To capture a more complex representation, we design different encoder parameters for different types of nodes and edges in HAT. Extensive experiments validate that our HAT significantly outperforms the state-of-the-art methods on both the public datasets and a large-scale real-world fintech dataset(1).
更多查看译文
关键词
Graph Neural Networks,Heterogeneous graph,Node classification
AI 理解论文
溯源树
样例
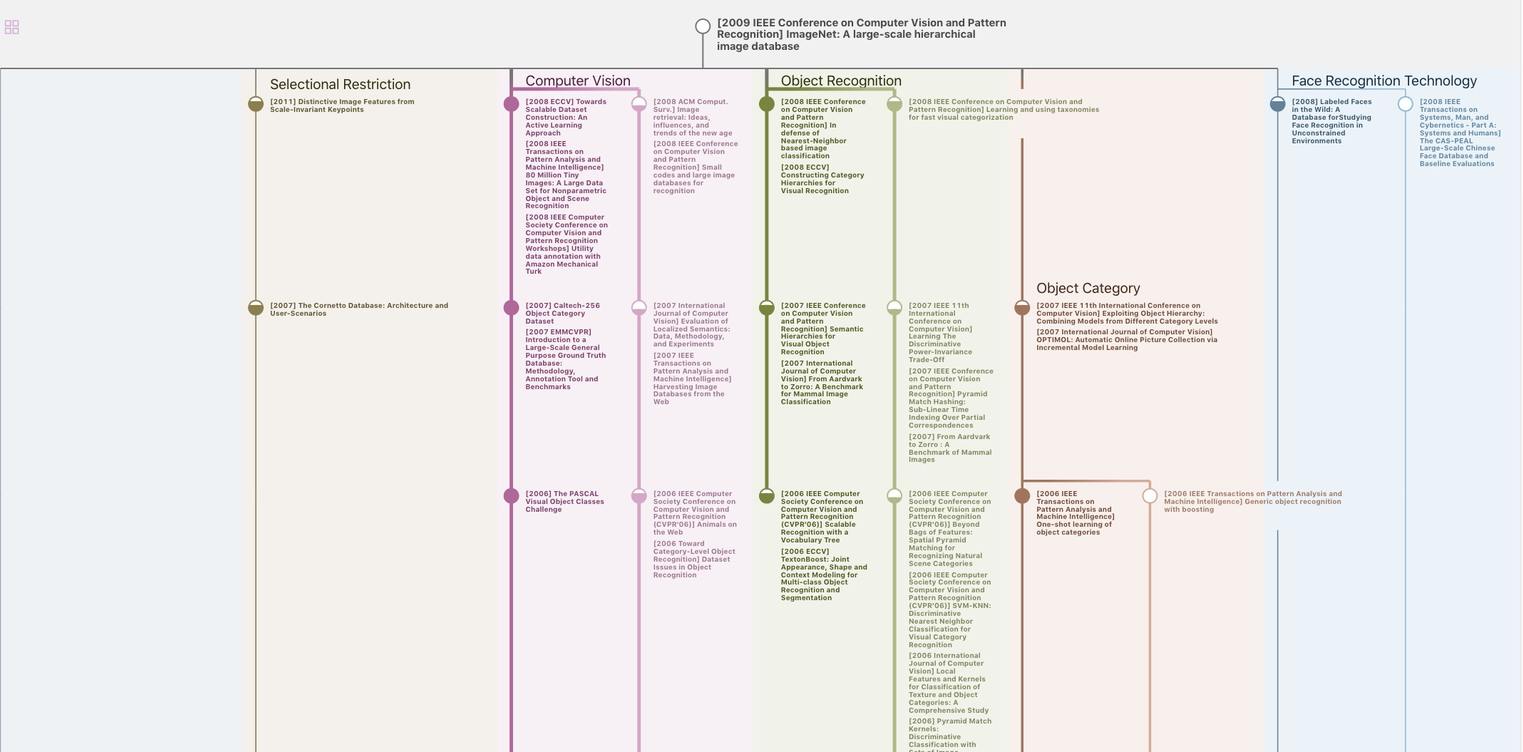
生成溯源树,研究论文发展脉络
Chat Paper
正在生成论文摘要