Graph Learning Information Criterion.
IEEE International Conference on Acoustics, Speech, and Signal Processing (ICASSP)(2022)
摘要
In this paper, we propose a parameter selection method for graph learning. Graph learning, a technique of learning graphs from observations, is required in many applications, e.g., classification, prediction, and clustering. However, there is no established method to determine hyperparameters that control the strength of the regularization reflecting prior knowledge. To resolve the problem, we consider a model selection criterion for the graph learning problem based on Laplacian constrained Gaussian Markov random field. The proposed criterion is the value based on model evidence, which is used for model selection in Bayesian statistics. It can be estimated by averaging the negative log-likelihood over the posterior distribution of a graph learning model. To compute this criterion, we present an efficient sampler of the posterior distribution. In the experiment with random graphs, we demonstrate that the proposed method can select hyperparameters having a good trade-off between F-measure and relative error.
更多查看译文
关键词
Graph learning,network topology inference,model evidence,information criterion
AI 理解论文
溯源树
样例
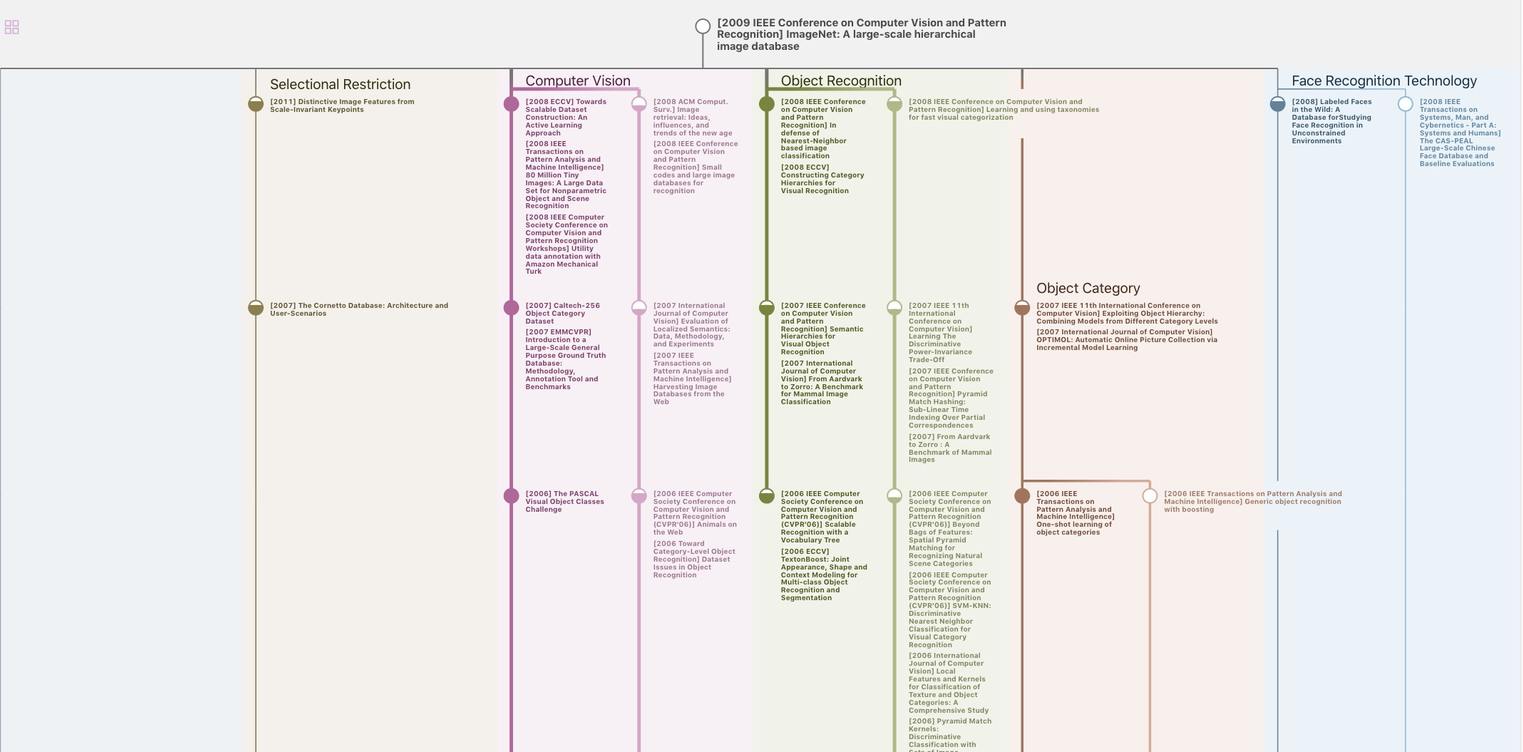
生成溯源树,研究论文发展脉络
Chat Paper
正在生成论文摘要