Pixel-Level and Affinity-Level Knowledge Distillation for Unsupervised Segmentation of Covid-19 Lesions.
IEEE International Conference on Acoustics, Speech, and Signal Processing (ICASSP)(2022)
摘要
Automatic segmentation of COVID-19 lesions is essential for computer-aided diagnosis. However, this task remains challenging because widely-used supervised based methods require large-scale annotated data that is difficult to obtain. Although an unsupervised method based on anomaly detection has shown promising results in [1], its performance is relatively poor. We address this problem by proposing a pixel-level and affinity-level knowledge distillation method. It obtains a pre-trained teacher network with rich semantic knowledge of CT images by constructing and training an auto-encoder at first, and then trains a student network with the same architecture as the teacher by distilling the teacher's knowledge only from normal CT images, and finally localizes COVID-19 lesions using the feature discrepancy between the teacher and the student networks. Besides, except for the traditional pixel-level distillation, we design the affinity-level distillation that takes into account the pairwise relationship of features to fully distill effective knowledge. We evaluate this method by using three different COVID-19 datasets and the experimental results show that the segmentation performance is largely improved when it is compared with the other existing unsupervised anomaly detection methods.
更多查看译文
关键词
Knowledge Distillation,Unsupervised Segmentation,Anomaly Detection,Affinity-Level
AI 理解论文
溯源树
样例
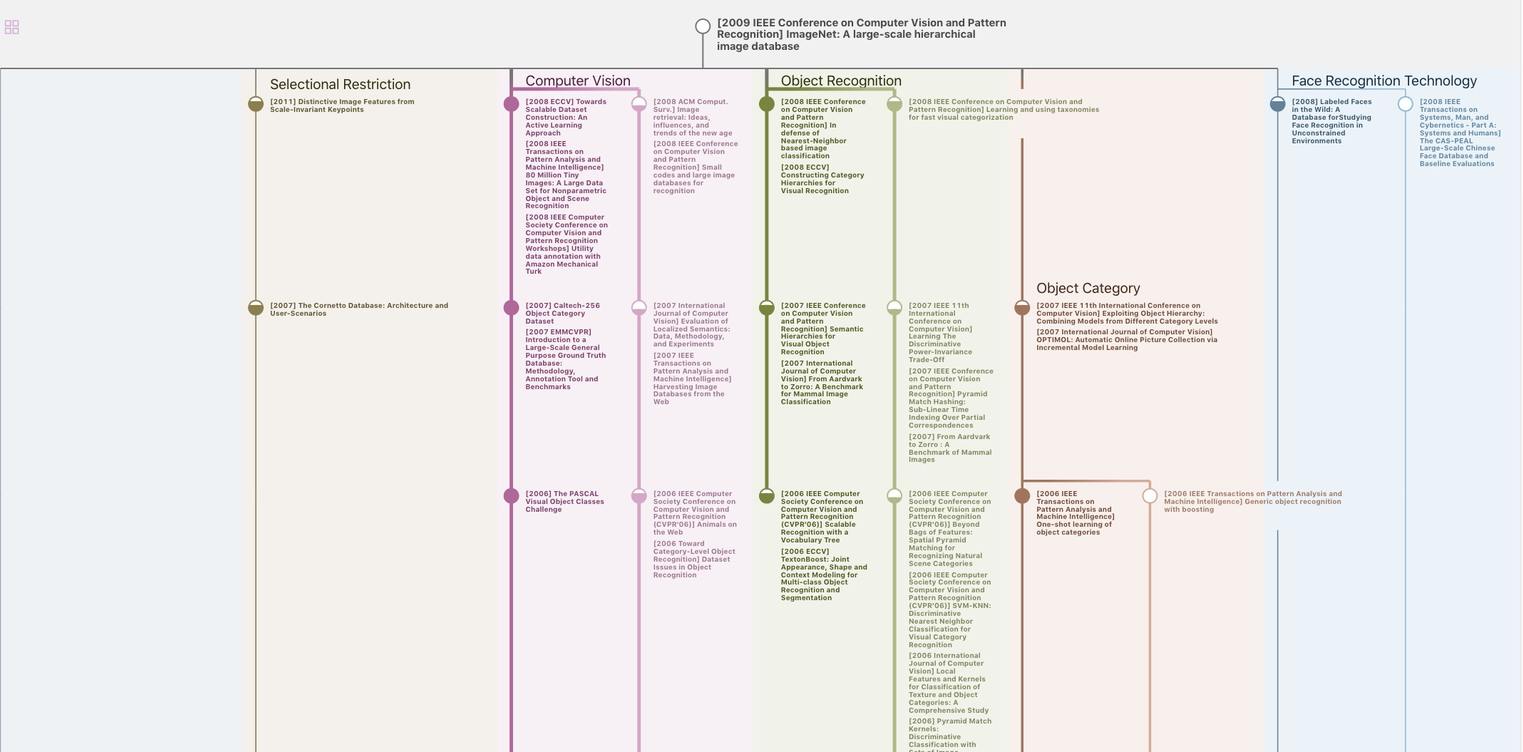
生成溯源树,研究论文发展脉络
Chat Paper
正在生成论文摘要