Time-Balanced Focal Loss for Audio Event Detection
ICASSP 2022 - 2022 IEEE International Conference on Acoustics, Speech and Signal Processing (ICASSP)(2022)
摘要
Sound Event Detection (SED) tackles the challenge of identifying sound events in an audio recording by delimiting both their temporal boundaries as well as sound category. With recent advances in deep learning, current systems are able to leverage availability of large datasets to train sophisticated and highly effective SED models. Nonetheless, sound sources and acoustic characteristics of different classes vary greatly in their prevalence as well as representation in labeled datasets. The challenge with data imbalance in the case of SED stems not only from the representation (number of samples) across classes but also the natural asymmetry in time duration across different events varying from short transient events such as the clacking of dishes to more sustained events such as vacuuming. This variability results in an inherent disproportional representation of effective training samples. To address this compounded imbalance issue, this work proposes a balanced focal learning function that introduces a novel time-sensitive classwise weight. The proposed loss is applied to SED in the context of DCASE2021 challenge, and reports a notable improvement over the baseline, particularly in the case of shorter sound events.
更多查看译文
关键词
Imbalanced data,focal loss,weighted loss,sound event detection,DCASE challenge
AI 理解论文
溯源树
样例
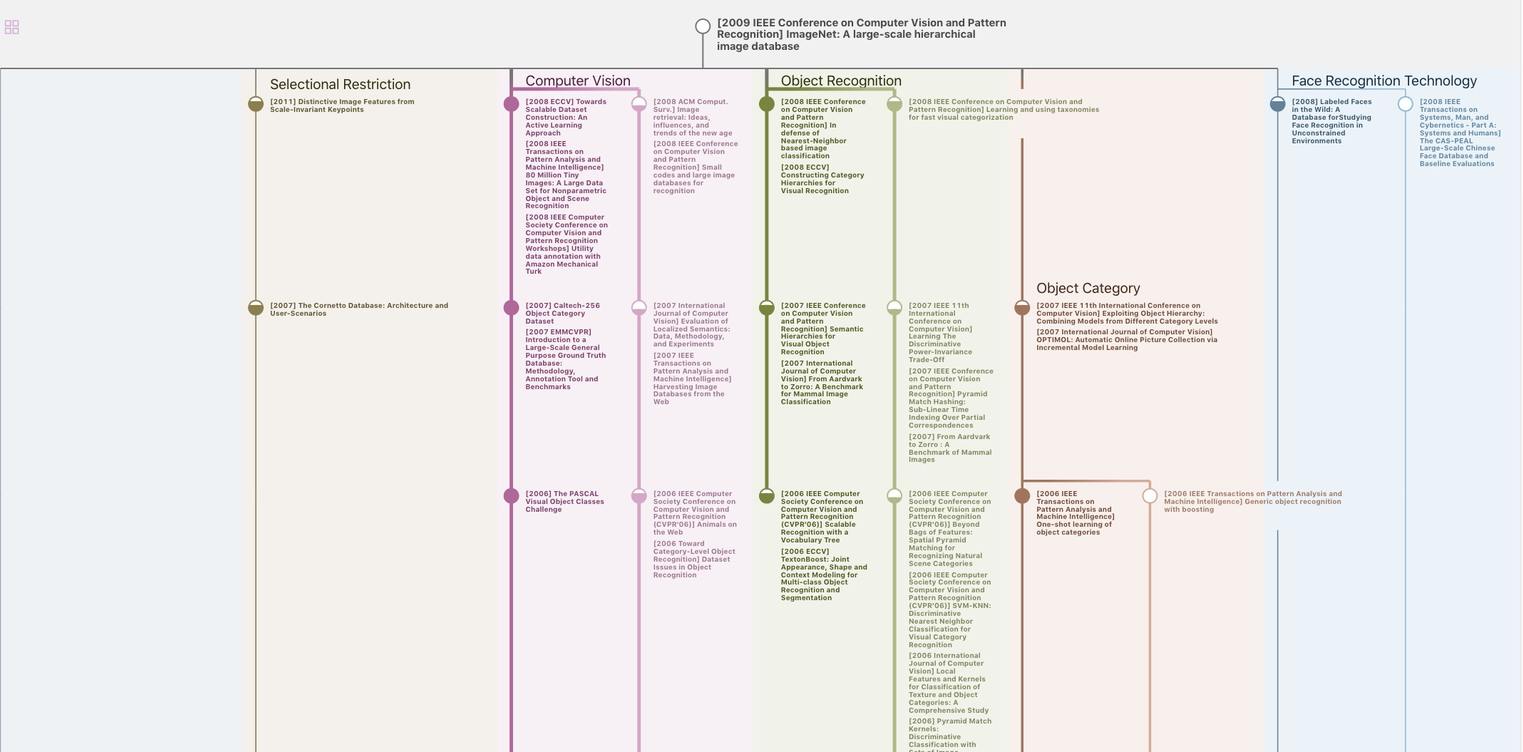
生成溯源树,研究论文发展脉络
Chat Paper
正在生成论文摘要