Local Context-aware Self-attention for Continuous Sign Language Recognition.
Conference of the International Speech Communication Association (INTERSPEECH)(2022)
摘要
Transformer-based architectures are adopted in many continuous sign language recognition (CSLR) works for sequence modeling due to their strong capability of extracting global contexts. However, since vanilla self-attention (SA), the core module of Transformer, computes a weighted average over all time steps, the local temporal semantics of sign videos may not be fully exploited. In this work, we propose local context-aware self-attention (LCSA) to enhance the vanilla SA to leverage both local and global contexts. We introduce the local contexts at two different levels of model computation: score and query levels. At the score level, we modulate the attention scores explicitly with an additional Gaussian bias. At the query level, local contexts are modeled implicitly using depth-wise temporal convolutional networks (DTCNs). However, the vanilla Gaussian bias has two major shortcomings: first, its window size is fixed and needs to be fine-tuned laboriously; second, the fixed window size is common among all time steps. In this work, a dynamic Gaussian bias is further proposed to address the above issues. Experimental results on two benchmarks, PHOENIX-2014 and CSL, validate the effectiveness and superiority of our method.
更多查看译文
关键词
continuous sign language recognition, self-attention, local contexts, sequence modeling
AI 理解论文
溯源树
样例
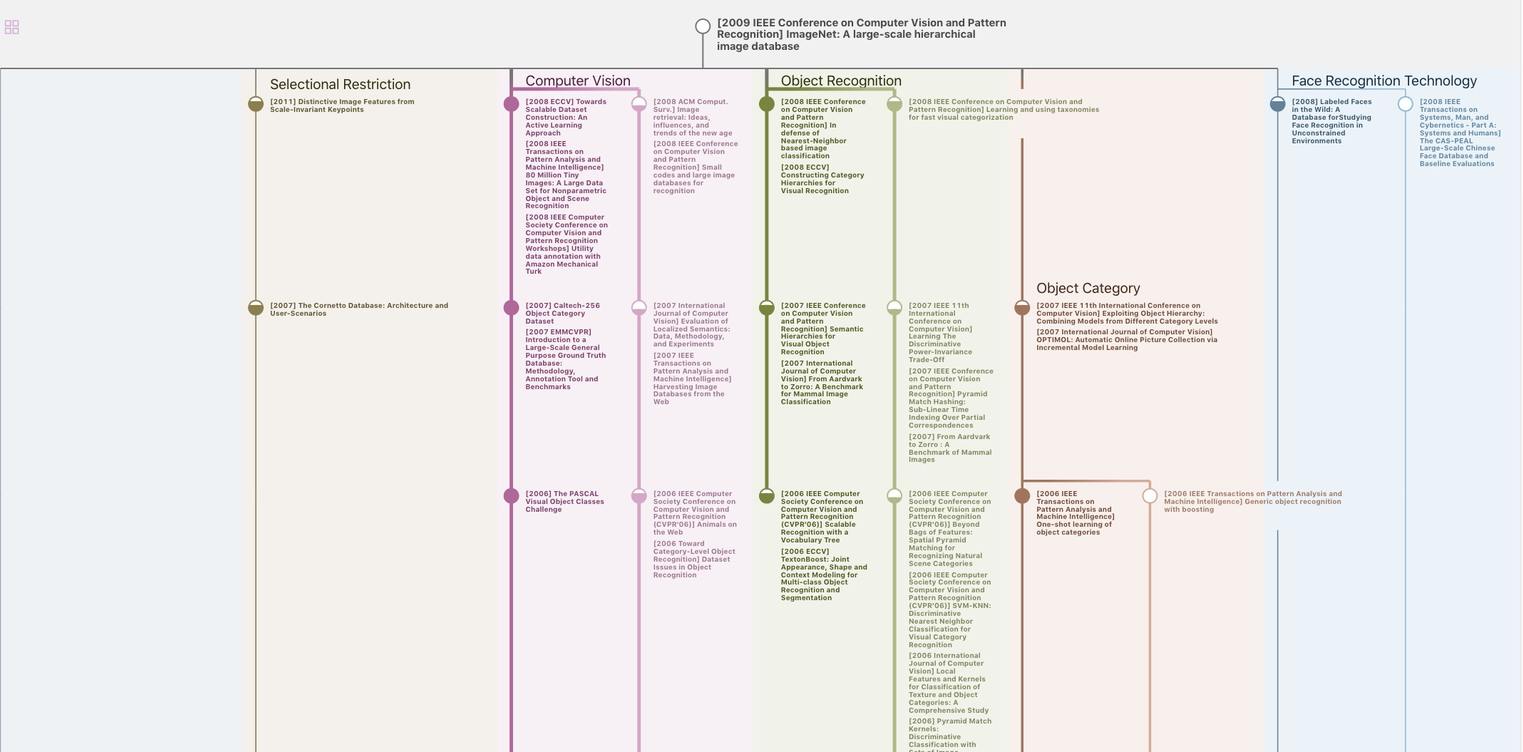
生成溯源树,研究论文发展脉络
Chat Paper
正在生成论文摘要