SoftSpeech: Unsupervised Duration Model in FastSpeech 2.
Conference of the International Speech Communication Association (INTERSPEECH)(2022)
摘要
In this paper, we propose a neural Text-To-Speech (TTS) system SoftSpeech, which employs a novel soft length regulated duration attention based decoder. It learns the encoder output mapping to decoder output simultaneously from an unsupervised duration model (Soft-LengthRegulator) without the requirement of external duration information. The Soft-LengthRegulator consists of a Feed-Forward Transformer (FFT) block with Conditional Layer Normalization (CLN), following a learned upsampling layer with multi-head attention and guided multi-head attention constraint, and it is integrated in each decoder layer and achieves accelerated training convergence and better naturalness within FastSpeech 2 framework. Soft Dynamic Time Warping (Soft-DTW) is adopted to align the mismatch spectrogram loss. Moreover, a Fine-Grained style Variational AutoEncoder (VAE) is designed to further improve the naturalness of synthesized speech. The experiments show SoftSpeech outperforms FastSpeech 2 in subjective tests, and can be successfully applied to minority languages with low resources.
更多查看译文
关键词
TTS, Soft-DTW, Attention, FastSpeech, VAE
AI 理解论文
溯源树
样例
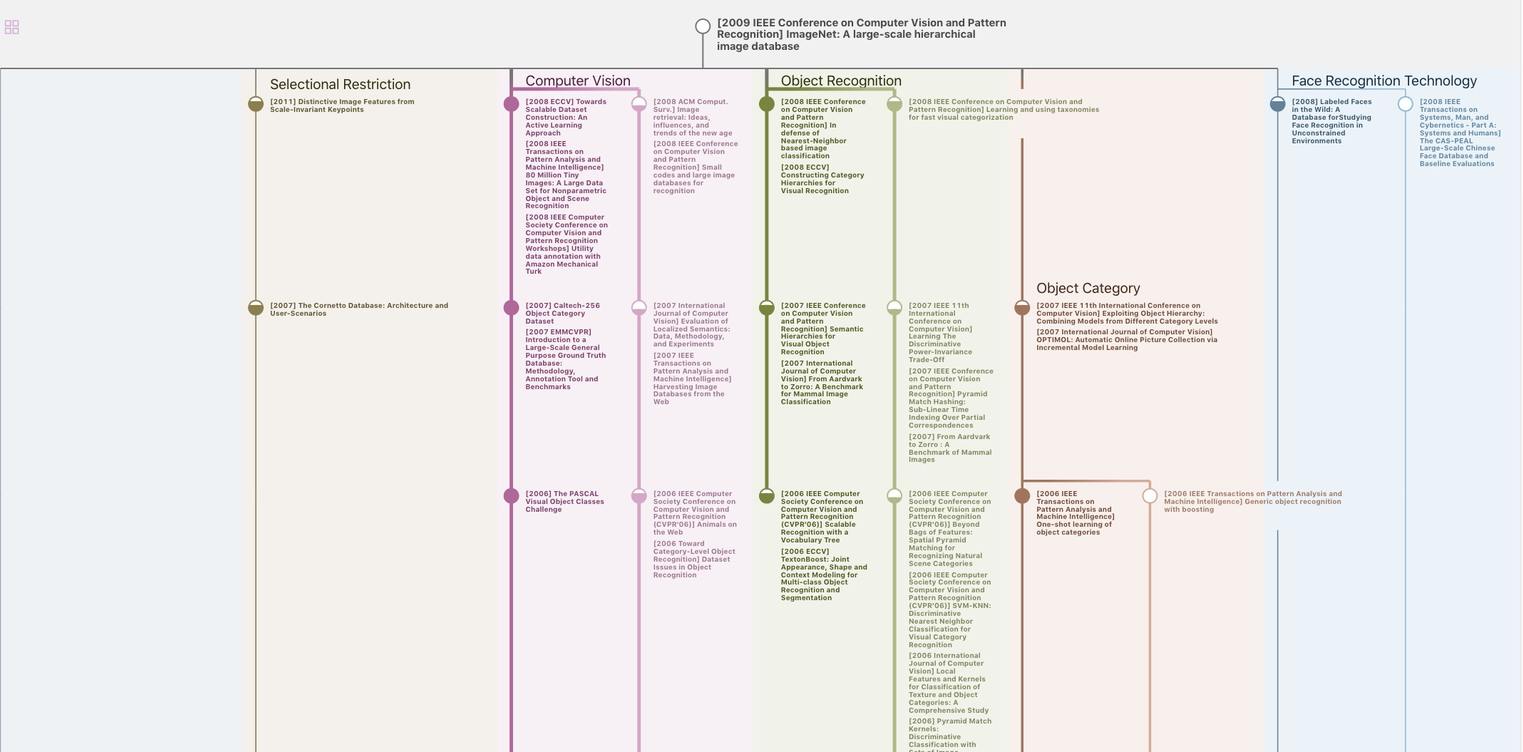
生成溯源树,研究论文发展脉络
Chat Paper
正在生成论文摘要