Deep denoising autoencoder-assisted continuous scoring of peak quality in high-resolution LC−MS data
Chemometrics and Intelligent Laboratory Systems(2022)
摘要
Accurate peak picking is a challenging but fundamental problem in LC-MS-based omics analysis. Previous efforts mainly focused on continuous wavelet transform (CWT) based peak detection, which is highly sensitive while suffers from unsatisfactory precision. Recently proposed deep learning (DL) based peak classifiers improve the performance significantly. However, their classification strategy loses the continuous criterion for controlling the false positive rate flexibly. Here we put forward AutoMS, which employs a deep learning-based denoising autoencoder to grasp the common characteristics of chromatographic peaks, and predict noise-deducted peaks from the original peak profiles. By comparing the difference before and after processed, it scores the peak quality continuously and precisely. From the evaluating result, AutoMS improved the accuracy for peak picking. AutoMS integrates HPIC for ROI extraction in order to accept raw data directly and output quantitative results. It also supports peak lists obtained from other tools with little adjustment. AutoMS is open source and available at https://github.com/hcji/AutoMS.
更多查看译文
关键词
peak quality,continuous scoring,lc−ms,autoencoder-assisted,high-resolution
AI 理解论文
溯源树
样例
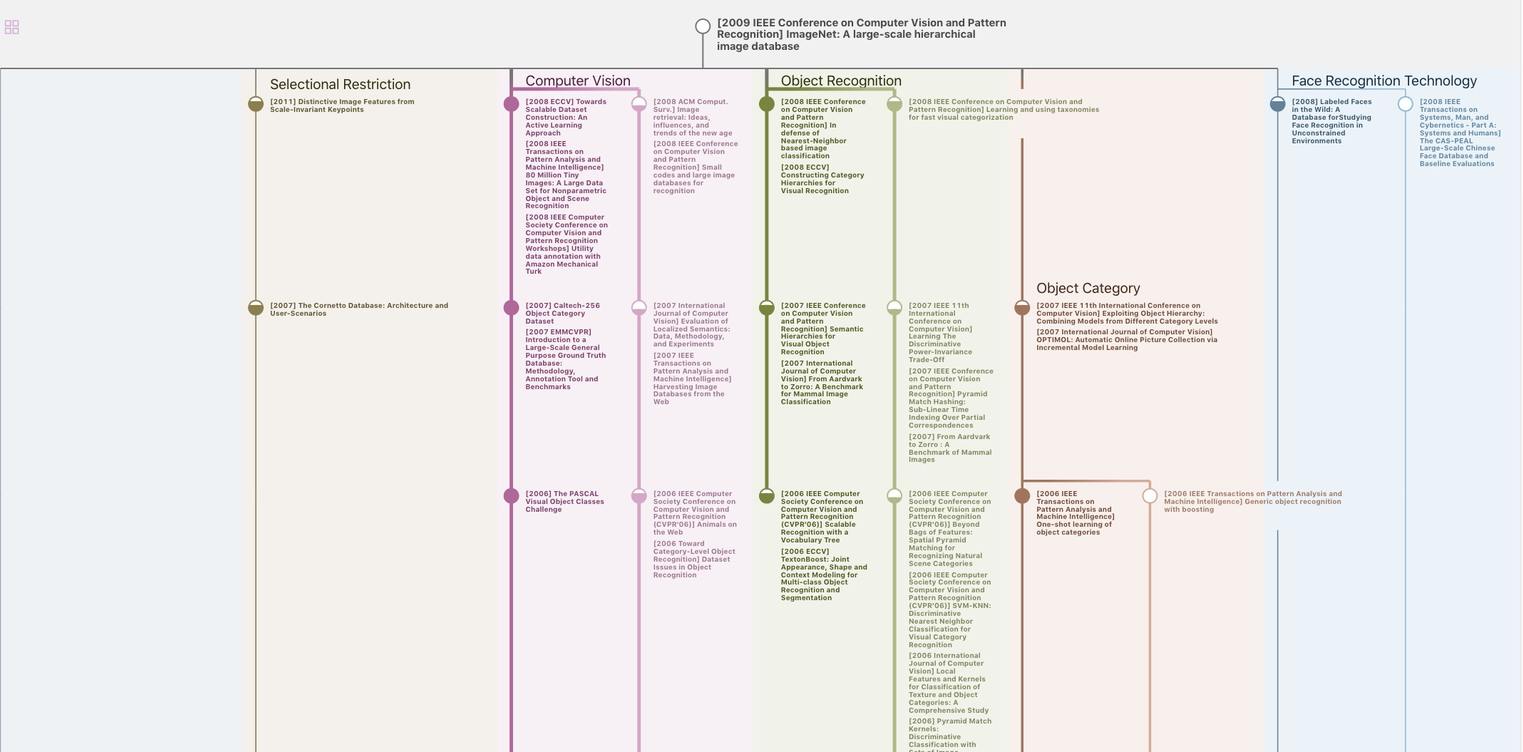
生成溯源树,研究论文发展脉络
Chat Paper
正在生成论文摘要