Evaluation of Neural Network Verification Methods for Air-to-Air Collision Avoidance
Journal of Air Transportation(2022)
摘要
Neural network approximations have become attractive to compress data for automation and autonomy algorithms for use on storage-limited and processing-limited aerospace hardware. However, unless these neural network approximations can be exhaustively verified to be safe, they cannot be certified for use on aircraft. An example of such systems is the unmanned Airborne Collision Avoidance System (ACAS) Xu, which is a very popular benchmark for open-loop neural network control system verification tools. This paper proposes a new closed-loop extension of this benchmark, which consists of a set of 10 closed-loop properties selected to evaluate the safety of an ownship aircraft in the presence of a co-altitude intruder aircraft. These closed-loop safety properties are used to evaluate five of the 45 neural networks that comprise the ACAS Xu benchmark (corresponding to co-altitude cases) as well as the switching logic between the five neural networks. The combination of nonlinear dynamics and switching between five neural networks is a challenging verification task accomplished with star-set reachability methods in two verification tools. The safety of the ownship aircraft under initial position uncertainty is guaranteed in every scenario proposed.
更多查看译文
关键词
neural network verification methods,air-to-air
AI 理解论文
溯源树
样例
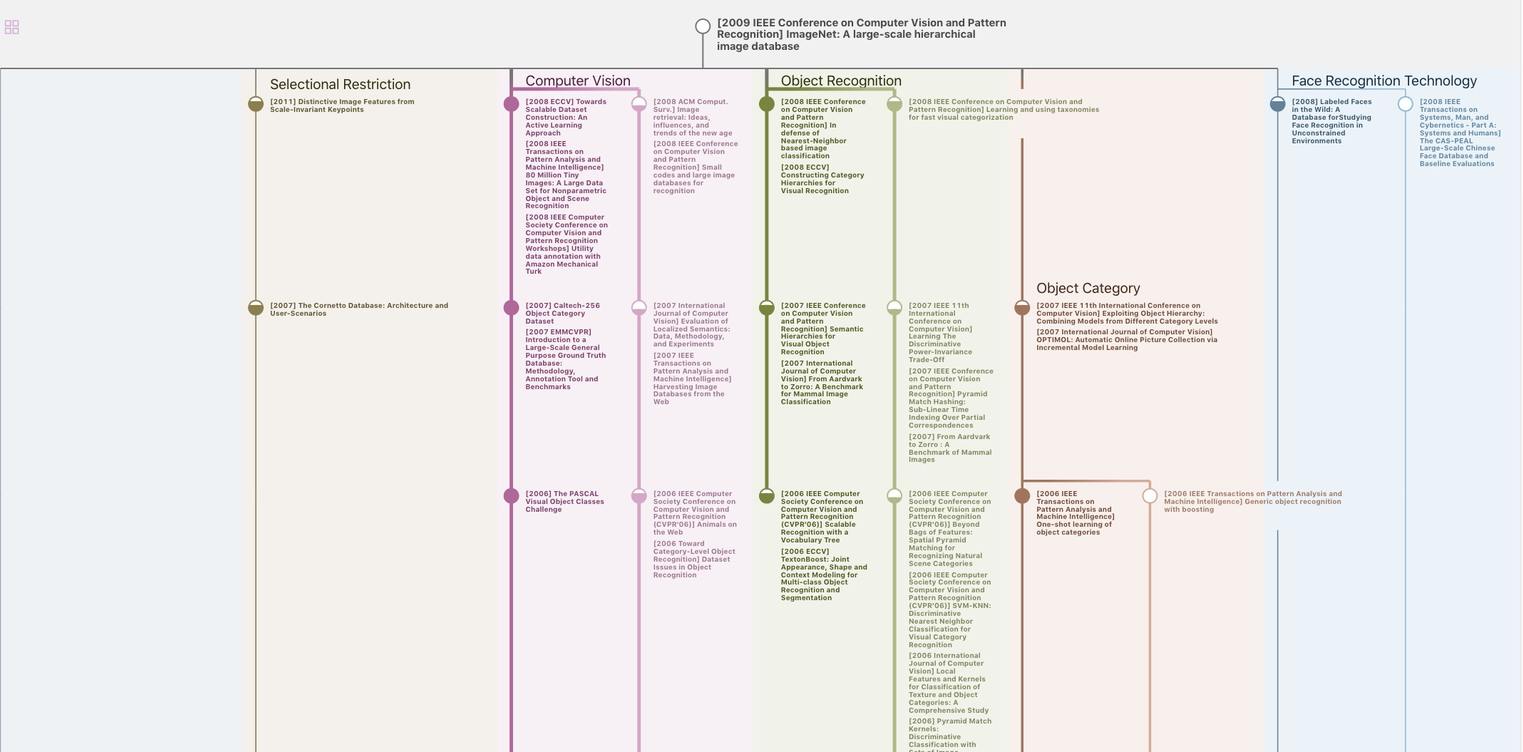
生成溯源树,研究论文发展脉络
Chat Paper
正在生成论文摘要