Transformer-Based Deep Learning for Sarcasm Detection with Imbalanced Dataset: Resampling Techniques with Downsampling and Augmentation
2022 13th International Conference on Information and Communication Systems (ICICS)(2022)
摘要
Sarcasm is a speech in which the speaker says something positive and means something negative to insult others. Sarcasm detection is a sentiment analysis problem in natural language processing (NLP) with no well-defined borders. Extracting the meaning of sarcastic content can impact the analysis of people’s tendencies in a particular situation. Due to the massive availability of online text data, storage resources, and the evolution of computing powers, deep learning techniques can be deployed in sarcasm detection. Thus, in this paper, different deep learning techniques and mechanisms are used to classify a given text as sarcastic or non-sarcastic in an imbalanced dataset. The transfer learning practice is adopted in this work by training pretrained transformer-based models, the BERT and the RoBERTa. In addition, three RNN-based models are used: the LSTM, BiLSTM, and BiGRU. The models are trained on a dataset from the SemEval2022 competition. The dataset is imbalanced and in the English language. The dataset is processed in two ways: the downsampling and the augmentation to produce a balanced dataset. The models are trained on the two resultant datasets and the original one. The results of the conducted training show that the ensemble of the transformer-based models trained on the original imbalanced dataset outperforms the baseline model in terms of F1-Score with a value of 0.43.
更多查看译文
关键词
NLP,BERT,RoBERTa,Sarcasm,Deep Learning,LSTM,BiLSTM,BiGRU and Transformers
AI 理解论文
溯源树
样例
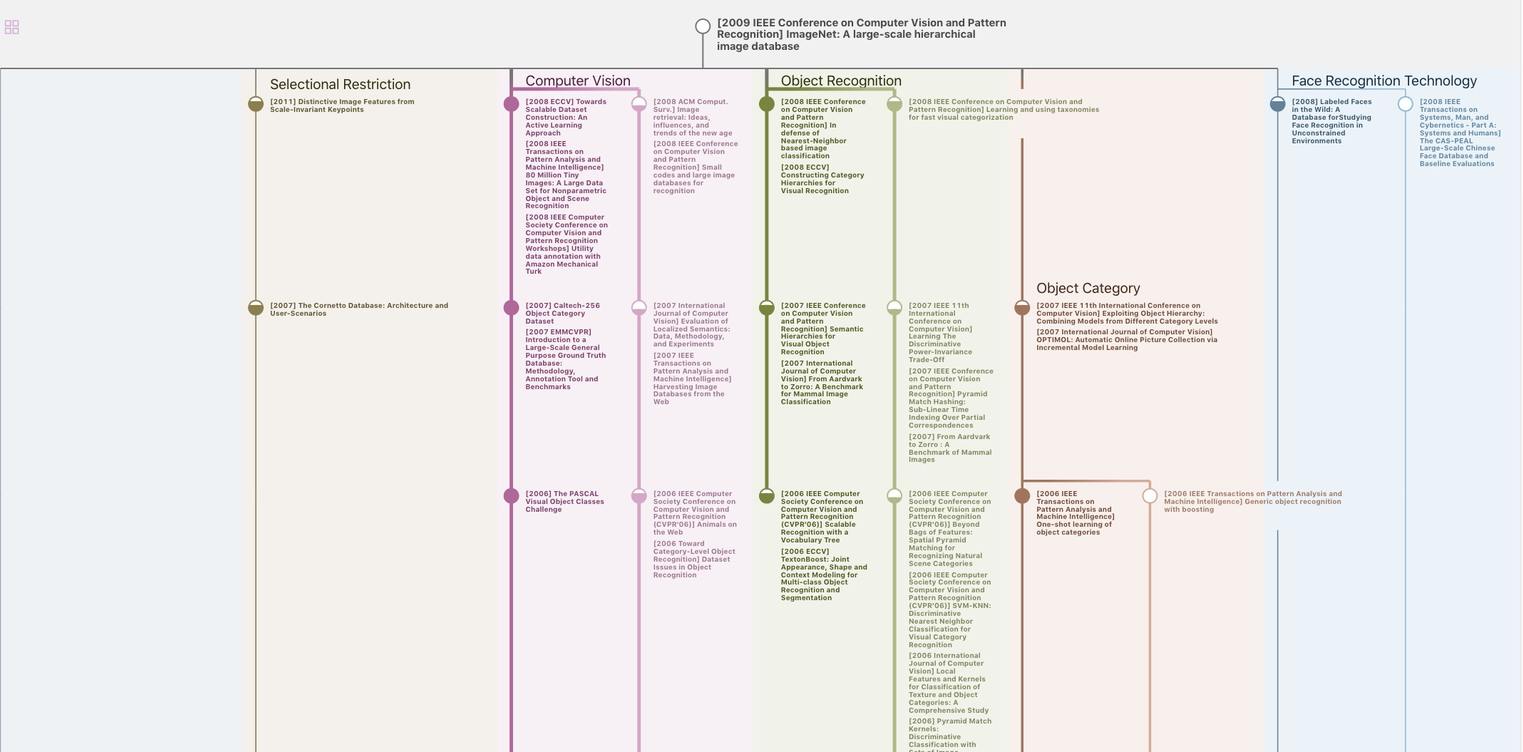
生成溯源树,研究论文发展脉络
Chat Paper
正在生成论文摘要