STDE: A Single-Senior-Teacher Knowledge Distillation Model for High-Dimensional Knowledge Graph Embeddings
2022 IEEE 2nd International Conference on Information Communication and Software Engineering (ICICSE)(2022)
摘要
An important role of Knowledge Graph Embedding (KGE) is to automatically complete the missing fact in a knowledge base. It is well-known that human society is constantly developing and the knowledge generated by human society will always being increasing. The increasing scale of the knowledge base is a great challenge to the storage and computing resources of downstream applications. At present, the dimensions of most mainstream knowledge graph embedding models are between 200-1000. For a large-scale knowledge base with millions of entities, these embeddings with hundreds of dimensional values are not conducive to rapid and frequent deployment on many kinds of artificial intelligent applications with limited storage and computing resources. To solve this problem, we propose a single-senior-teacher knowledge distillation model for high-dimensional knowledge graph embeddings named STDE, which constructs a low-dimensional student from a trained high-dimensional teacher. In STDE, the senior teacher can help the student learn key knowledge from correct knowledge and indistinguishable wrong knowledge with use of high-quality negative samples of triplets. We apply STDE to four typical KGE models on two famous data sets. Experimental results show that STDE can compress the embedding parameters of high-dimensional KGE models to 1/8 or 1/16 of their original scales. We further verify the effectiveness of "senior teacher" through ablation experiments.
更多查看译文
关键词
knowledge graph embedding,knowledge distillation,link prediction
AI 理解论文
溯源树
样例
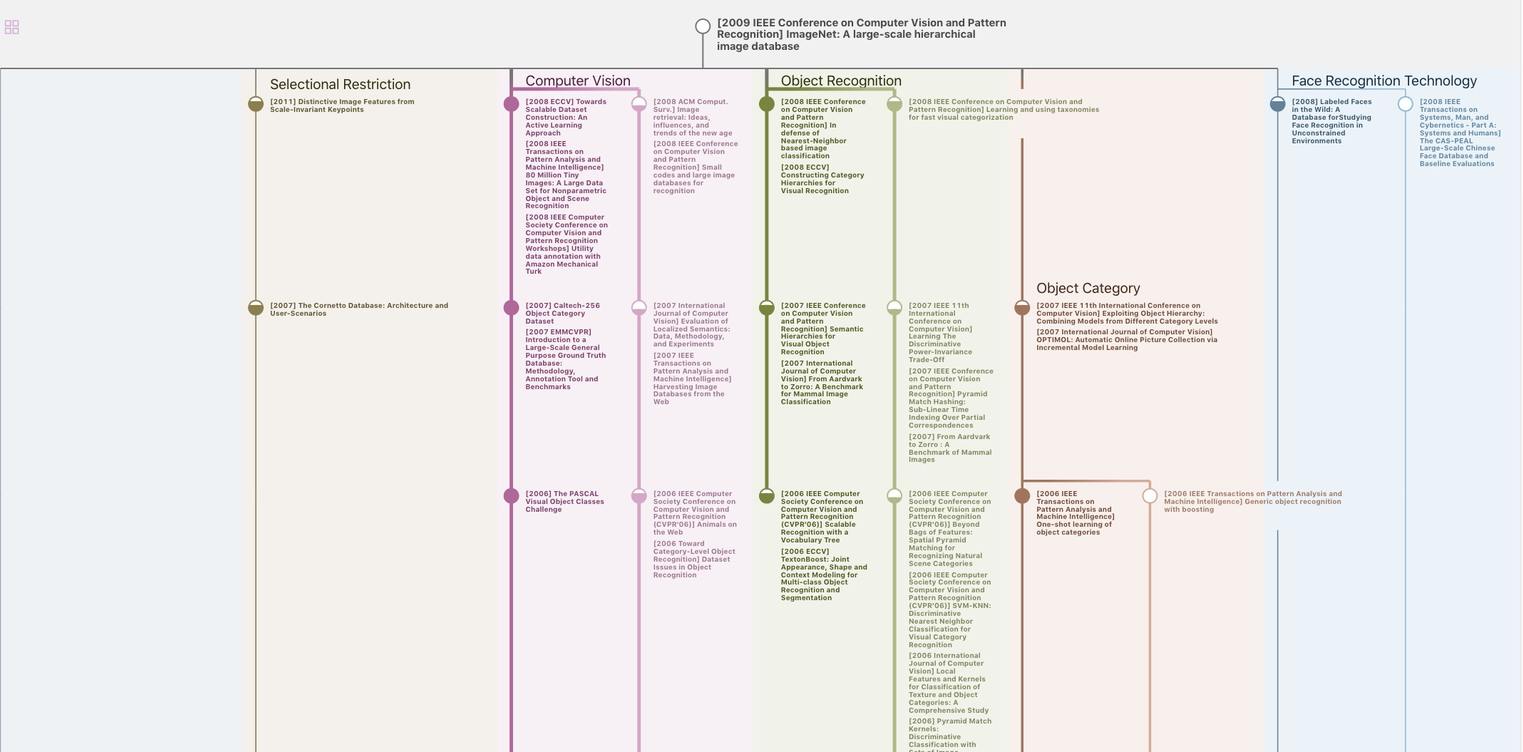
生成溯源树,研究论文发展脉络
Chat Paper
正在生成论文摘要