Using time series analysis and dual-stage attention-based recurrent neural network to predict landslide displacement
Environmental Earth Sciences(2022)
Abstract
Accurately estimating and predicting landslide deformation is critical to the assessment of landslide hazards. This research proposes a landslide displacement prediction model based on Variational Mode Decomposition (VMD) and Dual-stage Attention-based Recurrent Neural Network (DA-RNN). Using the VMD algorithm, the proposed model first decomposes the cumulative displacement into trend displacement, periodic displacement, and random displacement, and classifies all trigger factor sequences into low-frequency and high-frequency components. Then, the feature sequences selected by grey relational analysis are fed into the DA-RNN model to predict the periodic and random displacements in strict mode. The final predicted displacement is the sum of the trend displacement, periodic displacement, and random displacement. The proposed model was verified and evaluated by the case of the Baishuihe landslide in the Three Gorges reservoir area of China. The root-mean-square error of the prediction results of the proposed model at monitoring stations ZG118 and XD01 are 5.964 and 13.033, respectively. The proposed model outperforms the baseline models of long short-term memory network, back propagation neural network, and least square support vector regression in terms of prediction accuracy and feasibility.
MoreTranslated text
Key words
Landslide displacement prediction,Dual-stage attention-based recurrent neural network,Variational mode decomposition,Deep learning
AI Read Science
Must-Reading Tree
Example
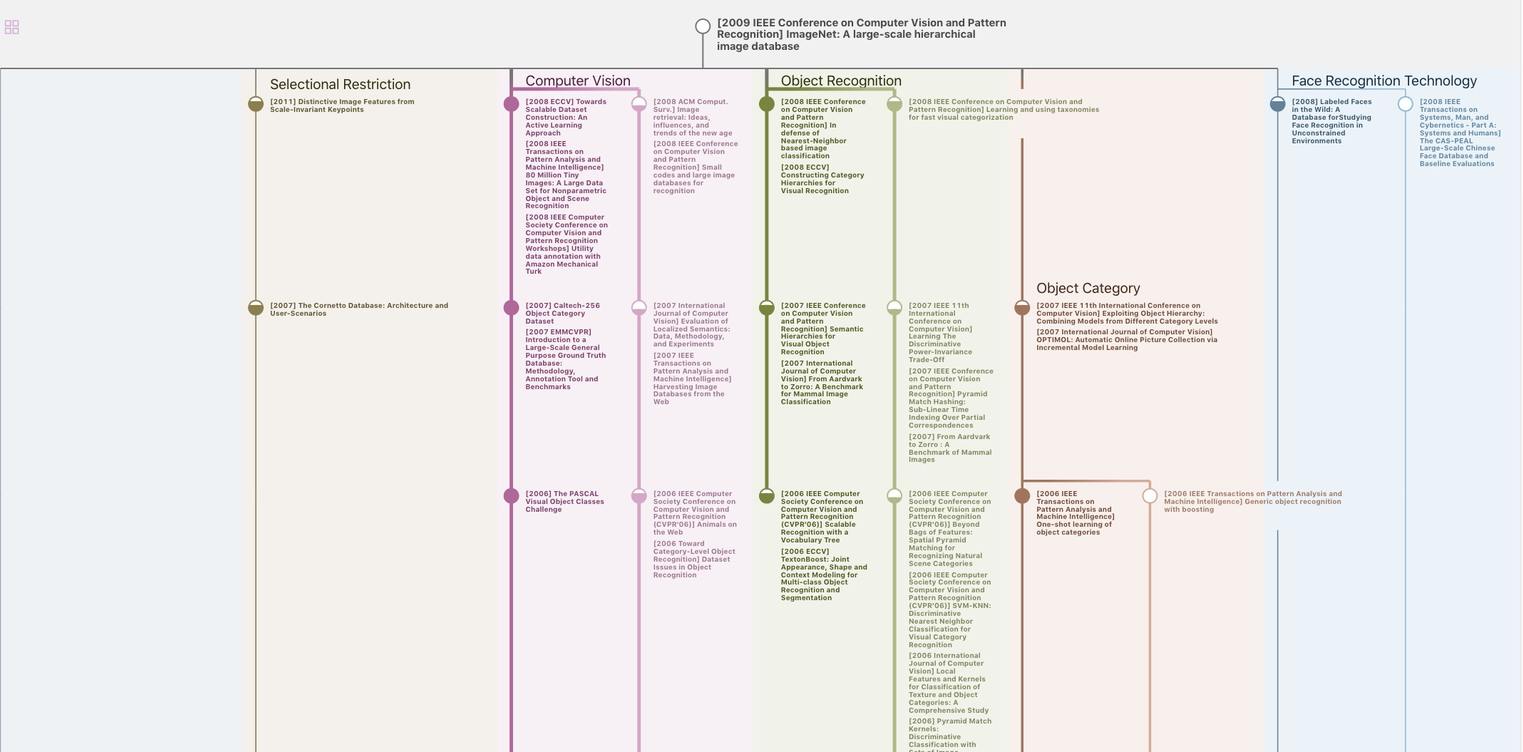
Generate MRT to find the research sequence of this paper
Chat Paper
Summary is being generated by the instructions you defined