On Zero-Dynamics Stealthy Attacks with Learned State Space Models
2024 4th International Conference on Smart Grid and Renewable Energy (SGRE)(2024)
Abstract
Zero-dynamics stealthy attacks are a subset of false data injection attacks (FDIAs) that can be catastrophic as they are designed to be undetectable by traditional residual based anomaly detectors. The attacker’s ability to successfully attack a system relies heavily on their capacity to learn an accurate state space model. Utilizing a grey box approach to system identification, we show that even when the attacker is able to learn a state space model close enough to have a high probability of a successful attack, making small improvements to the system’s anomaly detector causes the probability of a successful attack to drop drastically. Finally, we study the trade-offs between making the system less susceptible to zero-dynamics attacks and maintaining its controllability, by increasing the sampling time of the system, thus providing the attacker fewer samples to learn a state space model.
MoreTranslated text
Key words
State Space,State-space Model,Stealthy Attacks,Sampling Time,System Identification,Anomaly Detection,Attack Success,Injection Attacks,Probability Of Attack,False Data Injection Attacks,Normal Conditions,Control System,Learning Models,System State,Water Level,Catastrophic Events,System In Order,Equation Of State,Parameter Uncertainty,System Output,False Alarm Rate,Cyber Attacks,Learning Output,Learning Spaces,Tank Level,Types Of Attacks,Impact Of Attacks,Actual Output,Representation Learning,Internet Of Things Devices
AI Read Science
Must-Reading Tree
Example
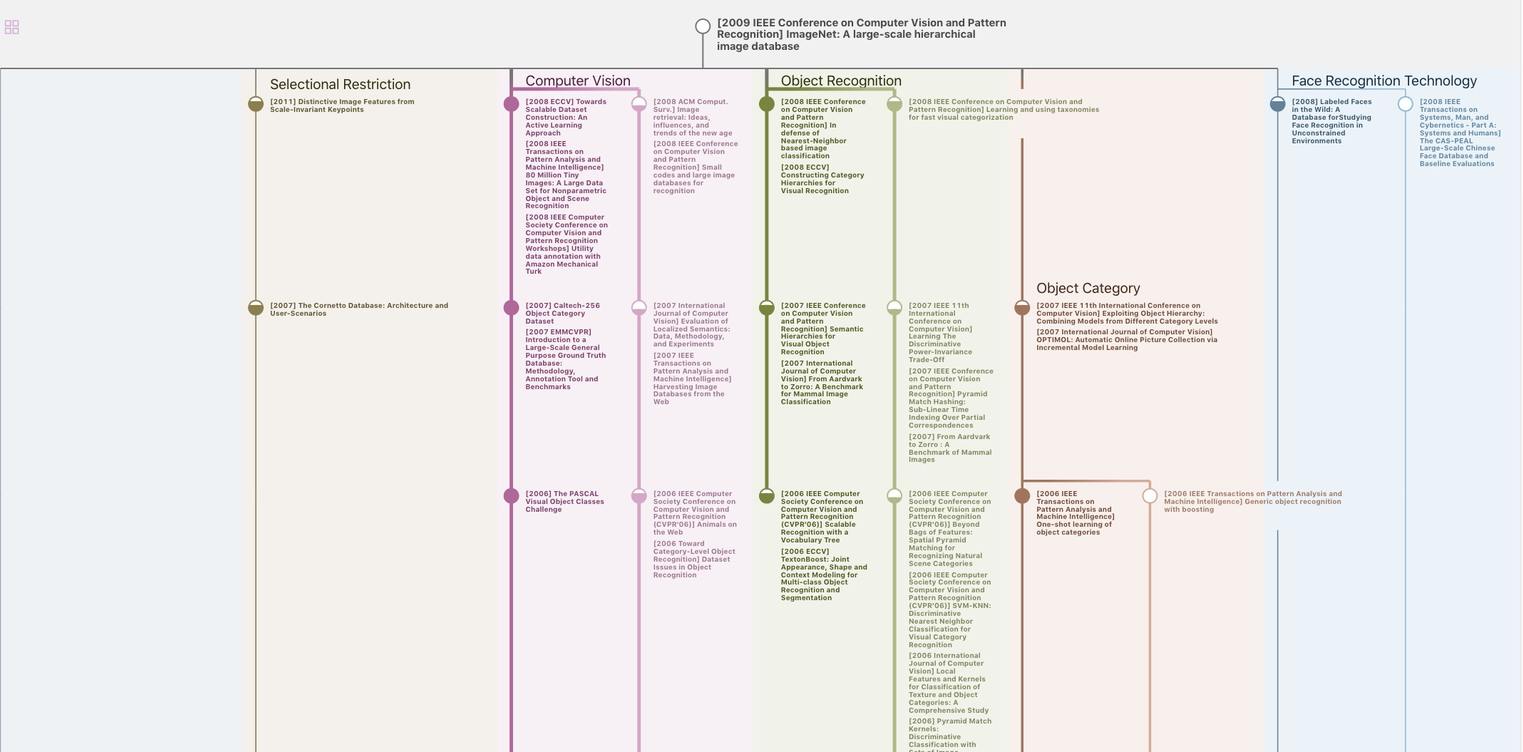
Generate MRT to find the research sequence of this paper
Chat Paper
Summary is being generated by the instructions you defined