Superstabilizing Control of Discrete-Time ARX Models under Error in Variables
arxiv(2023)
摘要
This paper applies a polynomial optimization-based framework towards the superstabilizing control of an Autoregressive with Exogenous Input (ARX) model given noisy data observations. The recorded input and output values are corrupted with L-infinity-bounded noise where the bounds are known. This is an instance of Error in Variables (EIV) in which true internal state of the ARX system remains unknown. The consistency set of ARX models compatible with noisy data has a bilinearity between unknown plant parameters and unknown noise terms. The requirement for a dynamic compensator to superstabilize all consistent plants is expressed using polynomial nonnegativity constraints, and solved using sum-of-squares (SOS) methods in a converging hierarchy of semidefinite programs in increasing size. The computational complexity of this method may be reduced by applying a Theorem of Alternatives to eliminate the noise terms. The effectiveness of this method is demonstrated on control of example ARX models.
更多查看译文
关键词
Data-based control,Linear systems,Sum-of-squares,Robust controller synthesis,Convex optimization,Uncertain systems
AI 理解论文
溯源树
样例
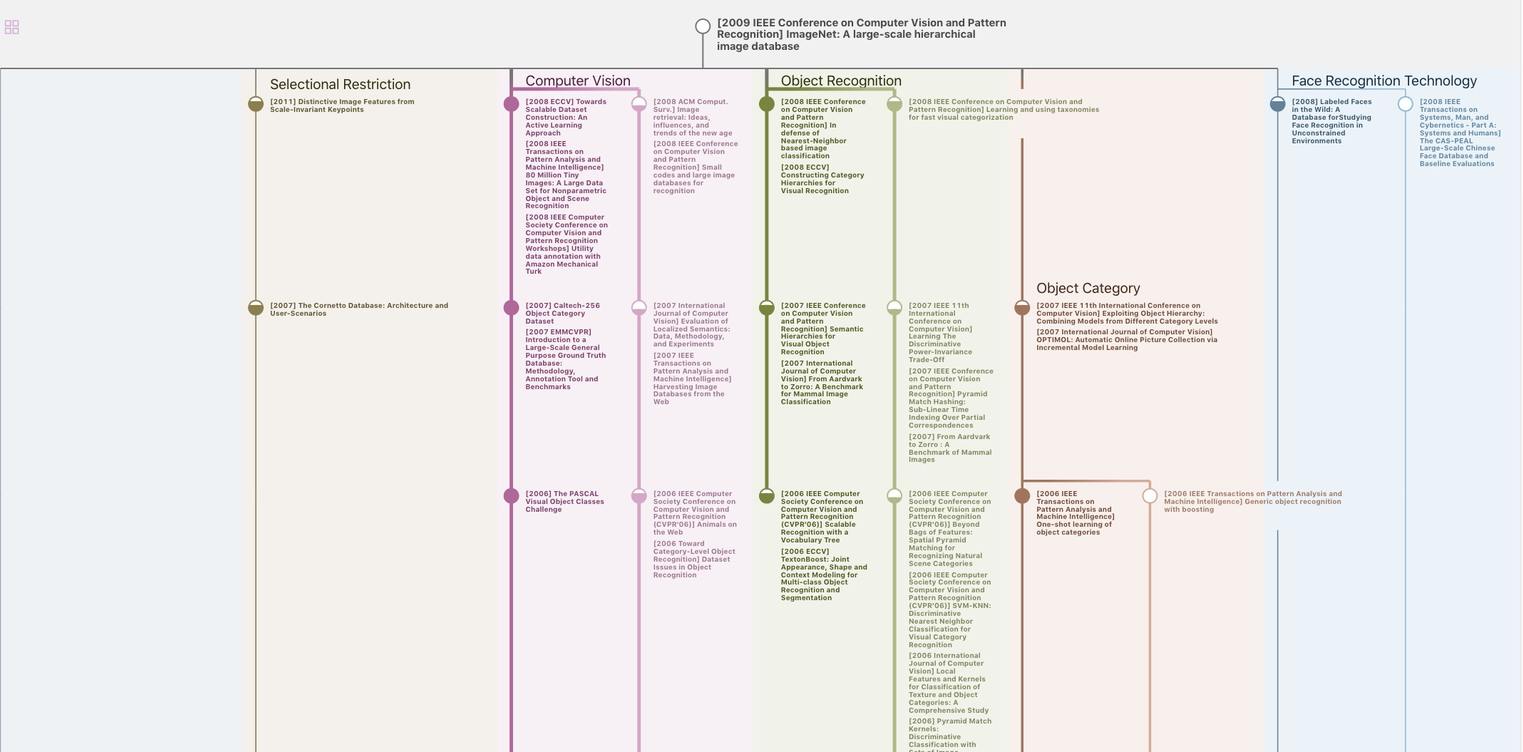
生成溯源树,研究论文发展脉络
Chat Paper
正在生成论文摘要