Leveraging Demonstrations with Latent Space Priors
Trans Mach Learn Res(2023)
摘要
Demonstrations provide insight into relevant state or action space regions, bearing great potential to boost the efficiency and practicality of reinforcement learning agents. In this work, we propose to leverage demonstration datasets by combining skill learning and sequence modeling. Starting with a learned joint latent space, we separately train a generative model of demonstration sequences and an accompanying low-level policy. The sequence model forms a latent space prior over plausible demonstration behaviors to accelerate learning of high-level policies. We show how to acquire such priors from state-only motion capture demonstrations and explore several methods for integrating them into policy learning on transfer tasks. Our experimental results confirm that latent space priors provide significant gains in learning speed and final performance. We benchmark our approach on a set of challenging sparse-reward environments with a complex, simulated humanoid, and on offline RL benchmarks for navigation and object manipulation. Videos, source code and pre-trained models are available at the corresponding project website at https://facebookresearch.github.io/latent-space-priors .
更多查看译文
关键词
latent space priors,demonstrations
AI 理解论文
溯源树
样例
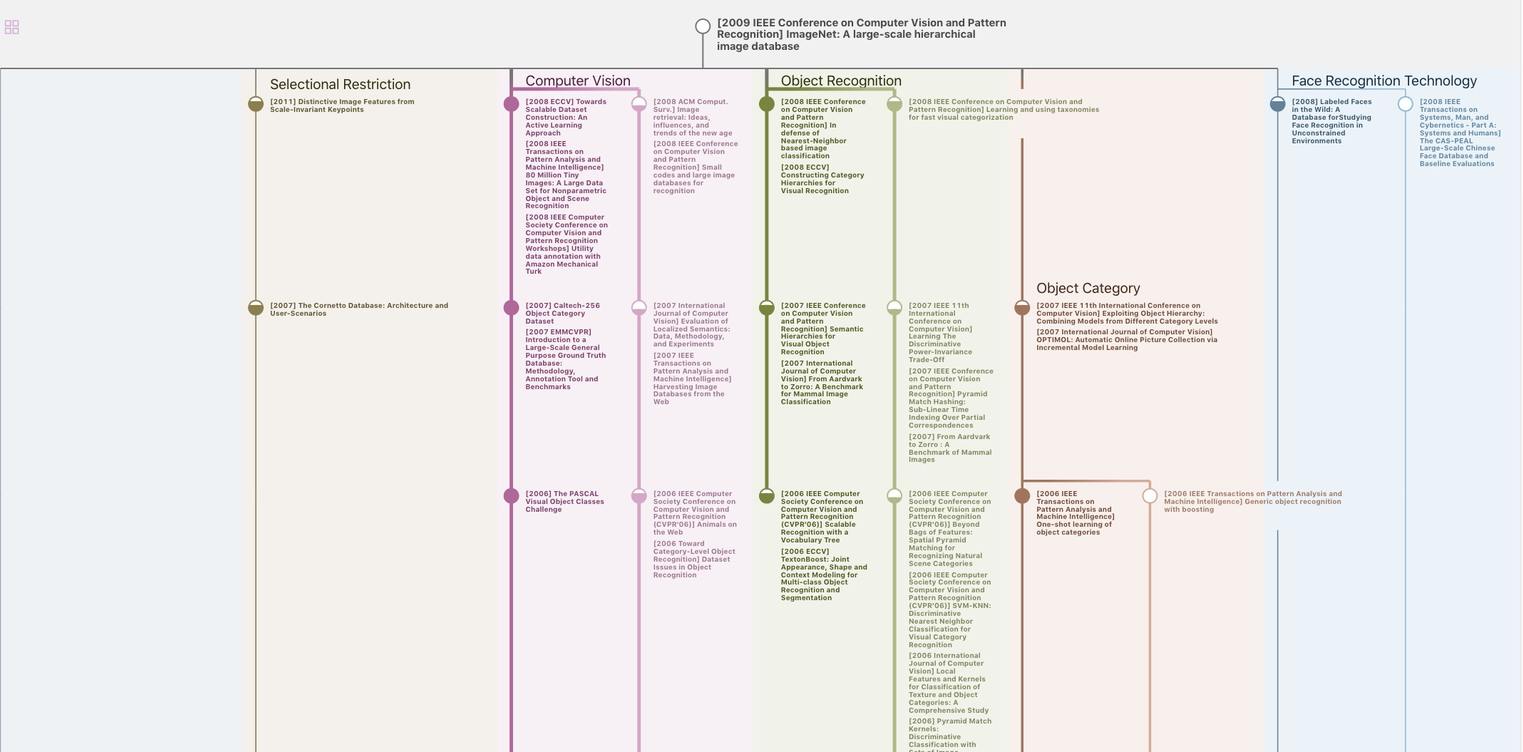
生成溯源树,研究论文发展脉络
Chat Paper
正在生成论文摘要