Hierarchical Federated Learning With Momentum Acceleration in Multi-Tier Networks
arxiv(2023)
摘要
In this article, we propose Hierarchical Federated Learning with Momentum Acceleration (HierMo), a three-tier worker-edge-cloud federated learning algorithm that applies momentum for training acceleration. Momentum is calculated and aggregated in the three tiers. We provide convergence analysis for HierMo, showing a convergence rate of O(1/T). In the analysis, we develop a new approach to characterize model aggregation, momentum aggregation, and their interactions. Based on this result, we prove that HierMo achieves a tighter convergence upper bound compared with HierFAVG without momentum. We also propose HierOPT, which optimizes the aggregation periods (worker-edge and edge-cloud aggregation periods) to minimize the loss given a limited training time. By conducting the experiment, we verify that HierMo outperforms existing mainstream benchmarks under a wide range of settings. In addition, HierOPT can achieve a near-optimal performance when we test HierMo under different aggregation periods.
更多查看译文
关键词
Federated learning,momentum,convergence analysis,edge computing
AI 理解论文
溯源树
样例
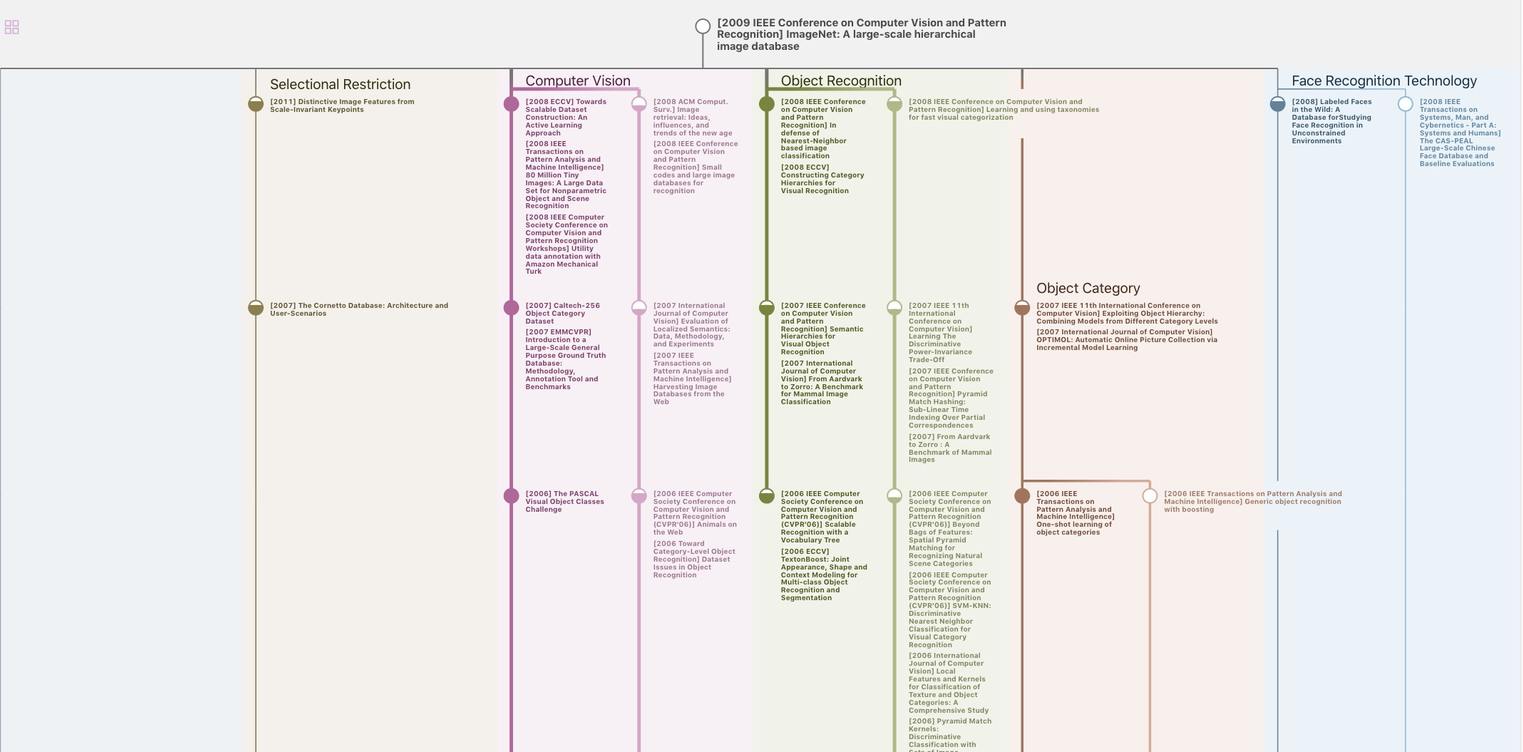
生成溯源树,研究论文发展脉络
Chat Paper
正在生成论文摘要