Uncertainty-based Meta-Reinforcement Learning for Robust Radar Tracking
arxiv(2022)
摘要
Nowadays, Deep Learning (DL) methods often overcome the limitations of traditional signal processing approaches. Nevertheless, DL methods are barely applied in real-life applications. This is mainly due to limited robustness and distributional shift between training and test data. To this end, recent work has proposed uncertainty mechanisms to increase their reliability. Besides, meta-learning aims at improving the generalization capability of DL models. By taking advantage of that, this paper proposes an uncertainty-based Meta-Reinforcement Learning (Meta-RL) approach with Out-of-Distribution (OOD) detection. The presented method performs a given task in unseen environments and provides information about its complexity. This is done by determining first and second-order statistics on the estimated reward. Using information about its complexity, the proposed algorithm is able to point out when tracking is reliable. To evaluate the proposed method, we benchmark it on a radar-tracking dataset. There, we show that our method outperforms related Meta-RL approaches on unseen tracking scenarios in peak performance by 16% and the baseline by 35% while detecting OOD data with an F1-Score of 72%. This shows that our method is robust to environmental changes and reliably detects OOD scenarios.
更多查看译文
关键词
Radar Sensors,Reinforcement Learning,Meta Learning,Out-of Distribution Detection
AI 理解论文
溯源树
样例
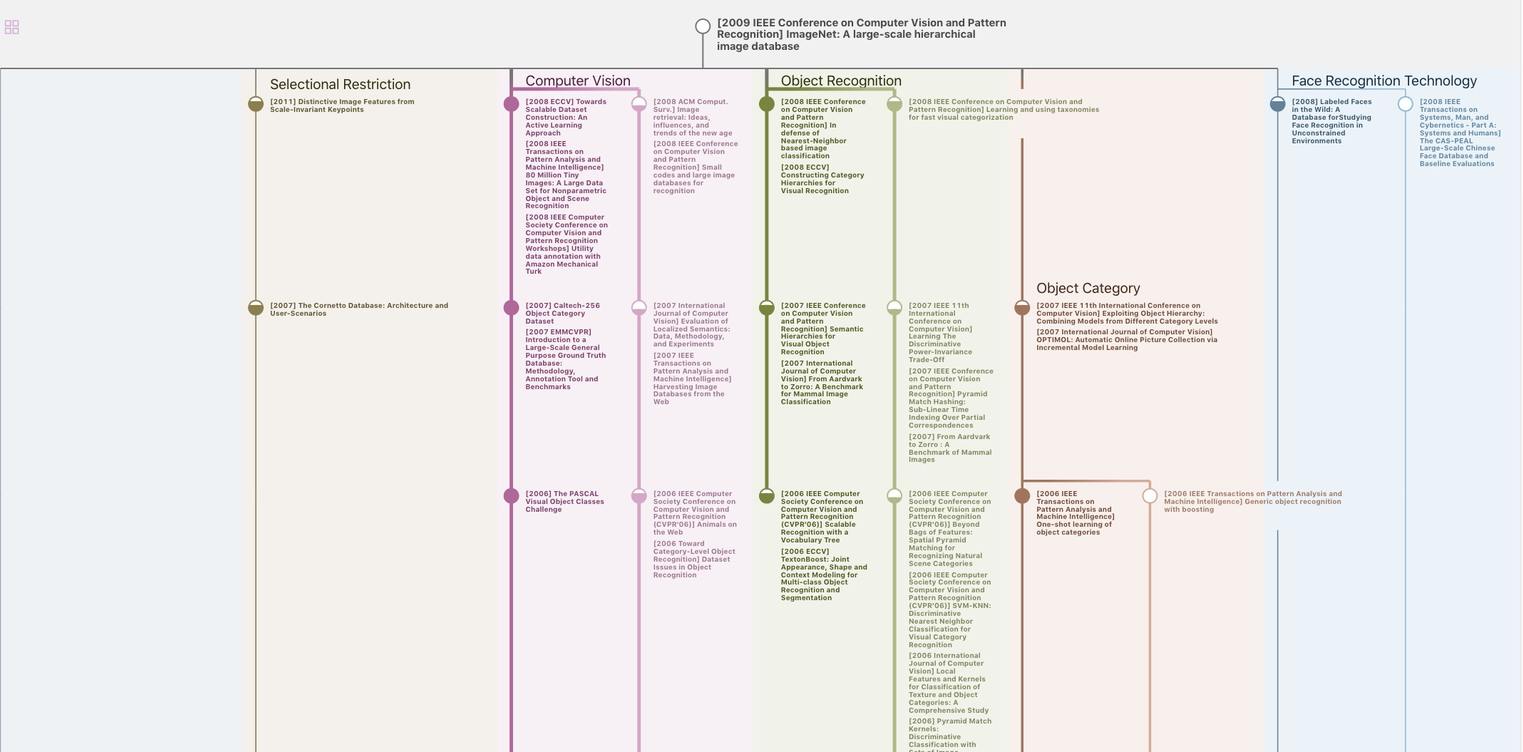
生成溯源树,研究论文发展脉络
Chat Paper
正在生成论文摘要