Moment Estimation for Nonparametric Mixture Models through Implicit Tensor Decomposition
SIAM JOURNAL ON MATHEMATICS OF DATA SCIENCE(2023)
摘要
We present an alternating least squares (ALS) type numerical optimization scheme to estimate conditionally independent mixture models in R-n, without parametrizing the distributions. Following the method of moments, we tackle an incomplete tensor decomposition problem to learn the mixing weights and componentwise means. Then we compute the cumulative distribution functions, higher moments, and other statistics of the component distributions through linear solves. Crucially for computations in high dimensions, the steep costs associated with high-order tensors are avoided, via the development of efficient tensor-free operations. Numerical experiments demonstrate the competitive performance of the algorithm and its applicability to many models and applications. Furthermore we provide theoretical analyses, establishing identifiability from low-order moments of the mixture and guaranteeing local linear convergence of the ALS algorithm.
更多查看译文
关键词
nonparametric mixture,moments,tensor decomposition,numerical optimization
AI 理解论文
溯源树
样例
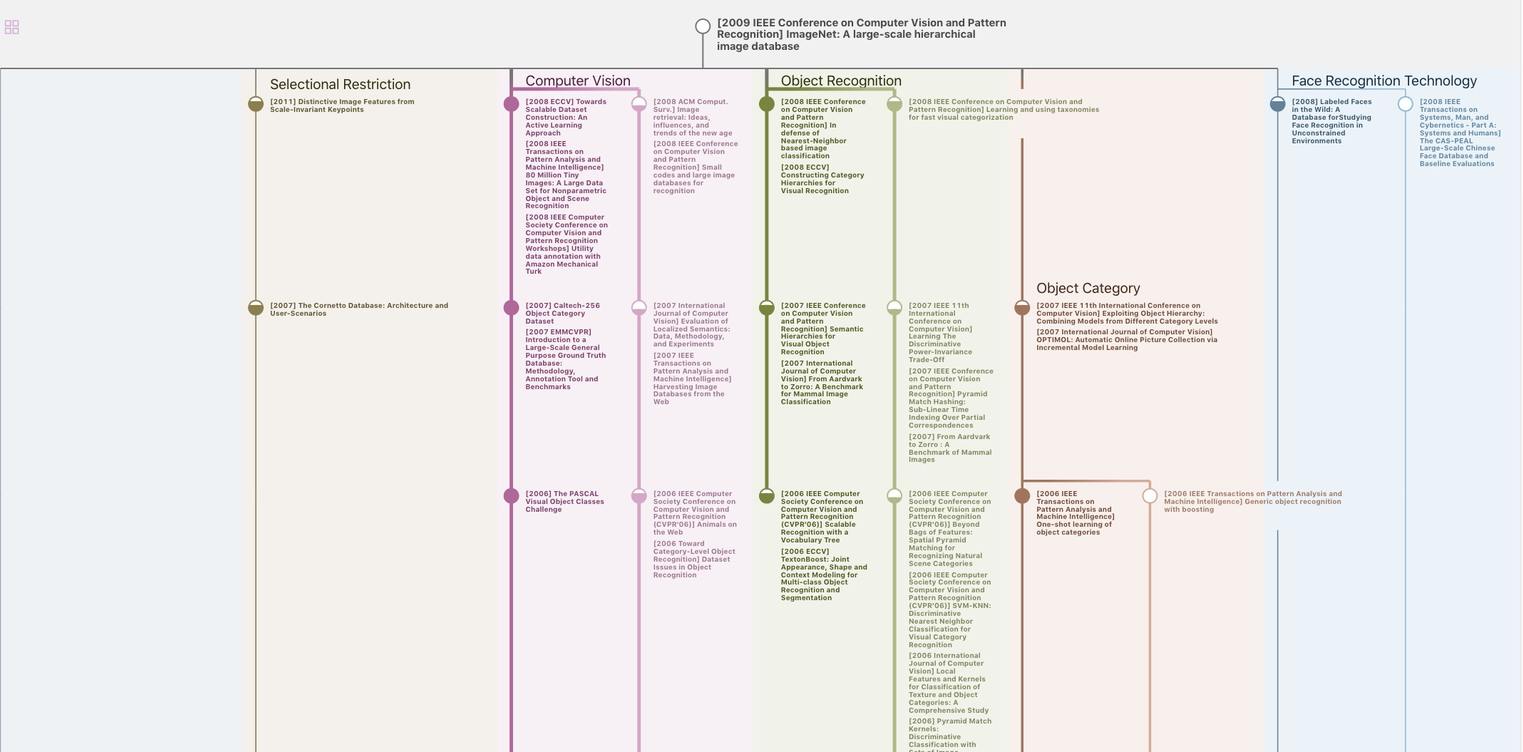
生成溯源树,研究论文发展脉络
Chat Paper
正在生成论文摘要