Adversarial Attacks on Protein Language Models
bioRxiv (Cold Spring Harbor Laboratory)(2022)
摘要
Deep Learning models for protein structure prediction, such as AlphaFold2, leverage Transformer architectures and their attention mechanism to capture structural and functional properties of amino acid sequences. Despite the high accuracy of predictions, biologically insignificant perturbations of the input sequences, or even single point mutations, can lead to substantially different 3d structures. On the other hand, protein language models are often insensitive to biologically relevant mutations that induce misfolding or dysfunction (e.g. missense mutations). Precisely, predictions of the 3d coordinates do not reveal the structure-disruptive effect of these mutations. Therefore, there is an evident inconsistency between the biological importance of mutations and the resulting change in structural prediction. Inspired by this problem, we introduce the concept of adversarial perturbation of protein sequences in continuous embedding spaces of protein language models. Our method relies on attention scores to detect the most vulnerable amino acid positions in the input sequences. Adversarial mutations are biologically diverse from their references and are able to significantly alter the resulting 3d structures.
### Competing Interest Statement
The authors acknowledge AREA Science Park supercomputing platform ORFEO made available for conducting the research reported in this paper, and the technical support of the staff of the Laboratory of Data Engineering. F.C. was supported by the grant PNR ``FAIR-by-design". A.C. was supported by the ARGO funding program.
更多查看译文
关键词
protein,language,models
AI 理解论文
溯源树
样例
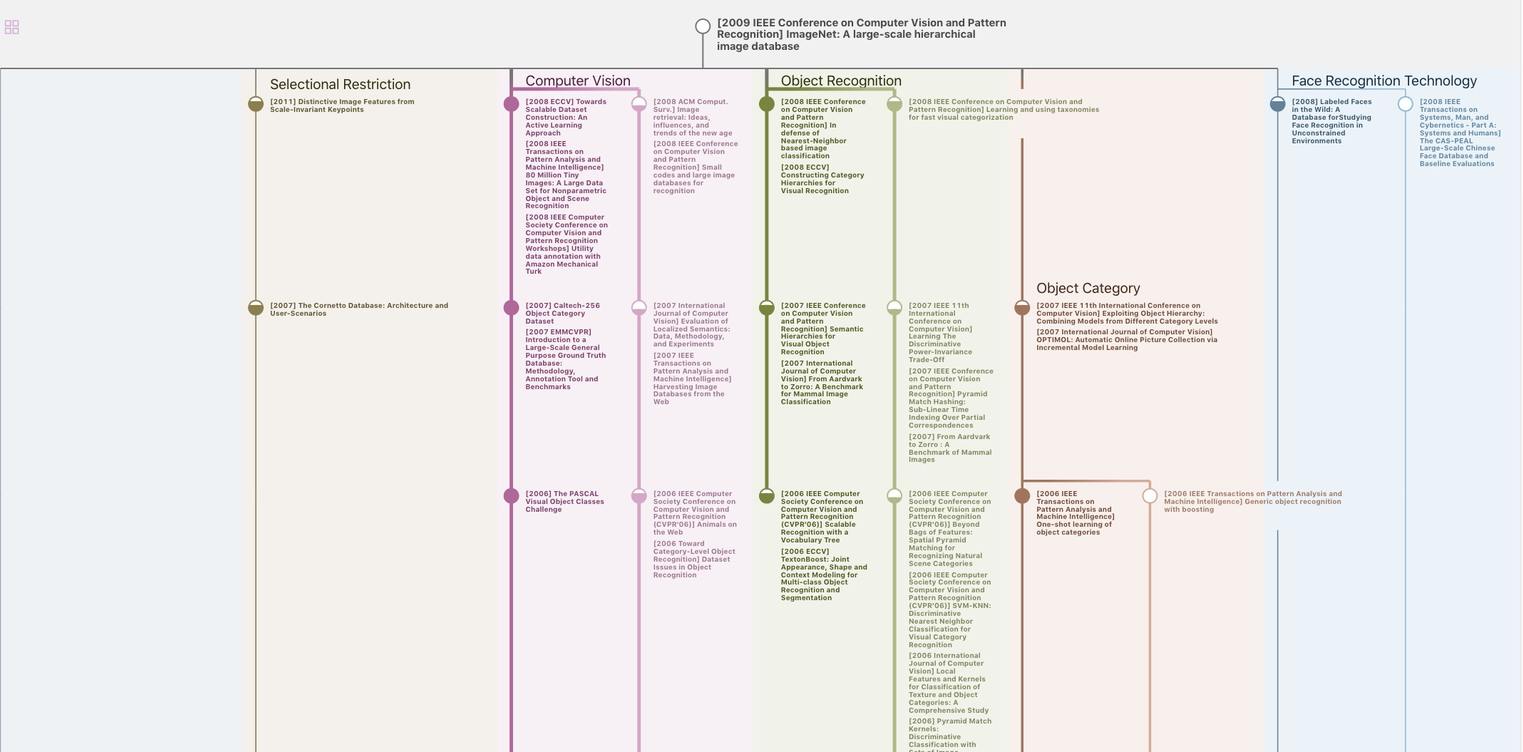
生成溯源树,研究论文发展脉络
Chat Paper
正在生成论文摘要