Motion correction in MRI using deep learning and a novel hybrid loss function
arxiv(2022)
摘要
Purpose To develop and evaluate a deep learning-based method (MC-Net) to suppress motion artifacts in brain magnetic resonance imaging (MRI). Methods MC-Net was derived from a UNet combined with a two-stage multi-loss function. T1-weighted axial brain images contaminated with synthetic motions were used to train the network. Evaluation used simulated T1 and T2-weighted axial, coronal, and sagittal images unseen during training, as well as T1-weighted images with motion artifacts from real scans. Performance indices included the peak signal to noise ratio (PSNR), structural similarity index measure (SSIM), and visual reading scores. Two clinical readers scored the images. Results The MC-Net outperformed other methods implemented in terms of PSNR and SSIM on the T1 axial test set. The MC-Net significantly improved the quality of all T1-weighted images (for all directions and for simulated as well as real motion artifacts), both on quantitative measures and visual scores. However, the MC-Net performed poorly on images of untrained contrast (T2-weighted). Conclusion The proposed two-stage multi-loss MC-Net can effectively suppress motion artifacts in brain MRI without compromising image context. Given the efficiency of the MC-Net (single image processing time ~40ms), it can potentially be used in real clinical settings. To facilitate further research, the code and trained model are available at https://github.com/MRIMoCo/DL_Motion_Correction.
更多查看译文
关键词
mri,motion correction,novel hybrid loss
AI 理解论文
溯源树
样例
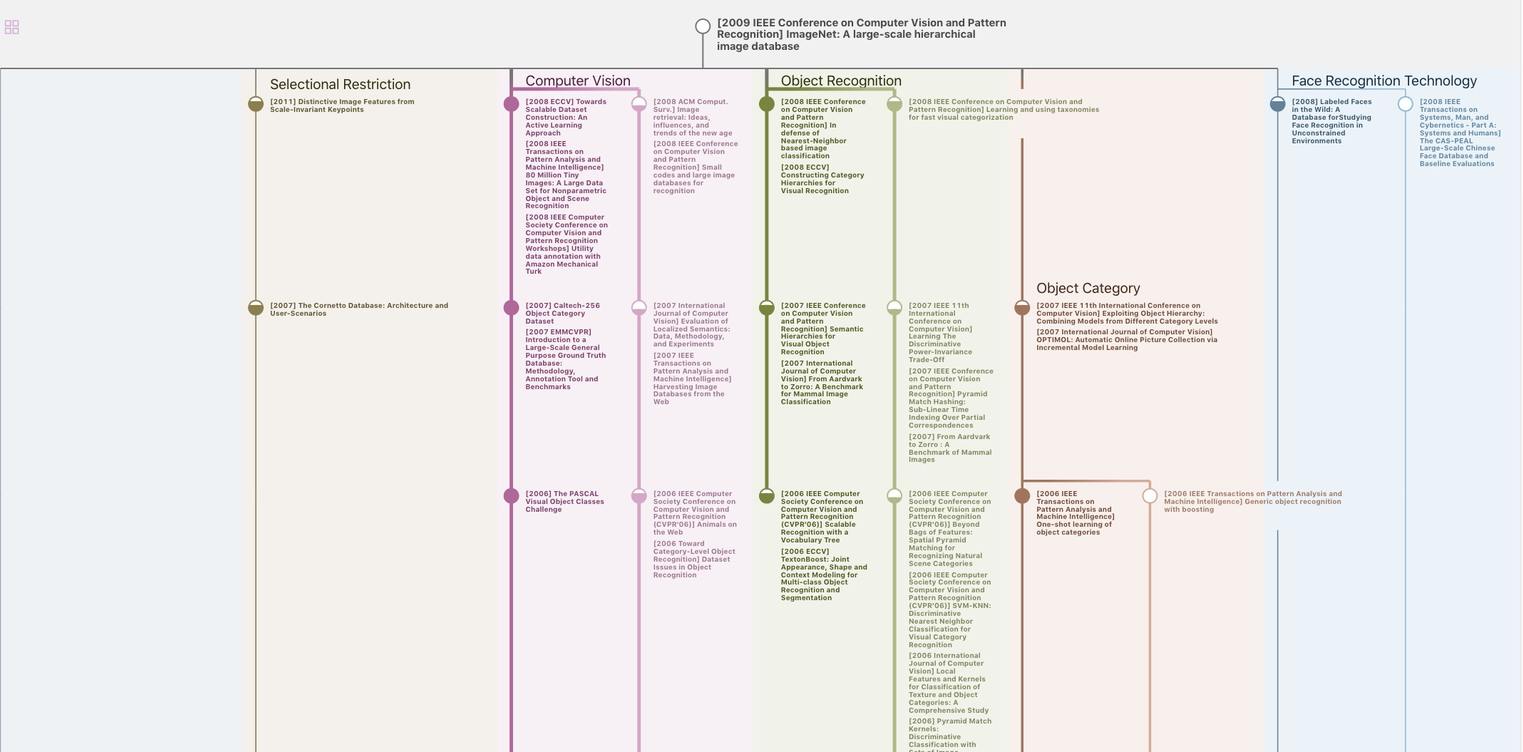
生成溯源树,研究论文发展脉络
Chat Paper
正在生成论文摘要