A Deep Fourier Residual method for solving PDEs using Neural Networks
Computer Methods in Applied Mechanics and Engineering(2023)
摘要
When using Neural Networks as trial functions to numerically solve PDEs, a key choice to be made is the loss function to be minimised, which should ideally correspond to a norm of the error. In multiple problems, this error norm coincides with – or is equivalent to – the H−1-norm of the residual; however, it is often difficult to accurately compute it. This work assumes rectangular domains and proposes the use of a Discrete Sine/Cosine Transform to accurately and efficiently compute the H−1 norm. The resulting Deep Fourier-based Residual (DFR) method efficiently and accurately approximate solutions to PDEs. This is particularly useful when solutions lack H2 regularity and methods involving strong formulations of the PDE fail. We observe that the H1-error is highly correlated with the discretised loss during training, which permits accurate error estimation via the loss.
更多查看译文
关键词
Deep learning,Neural Networks,Numerical PDEs,Fourier methods
AI 理解论文
溯源树
样例
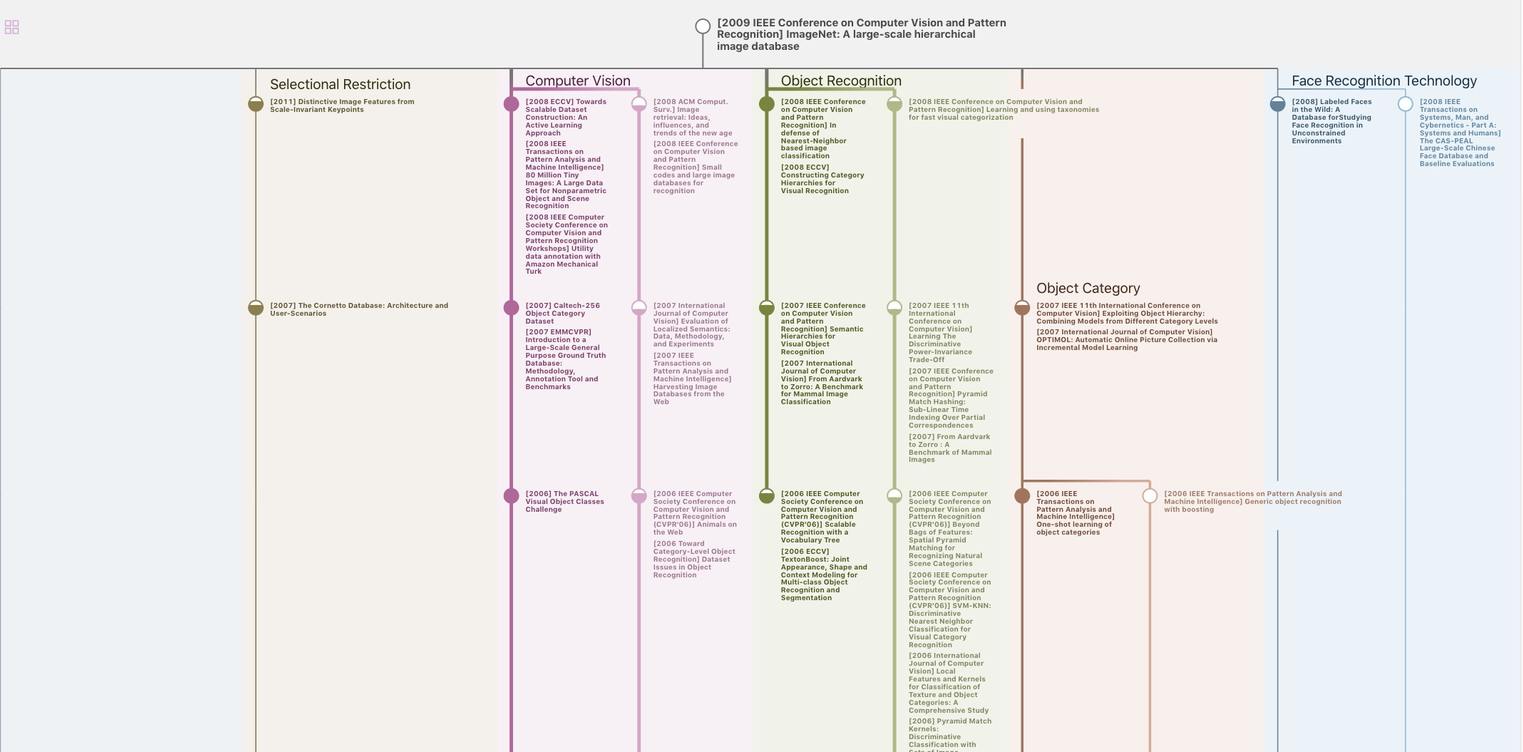
生成溯源树,研究论文发展脉络
Chat Paper
正在生成论文摘要