Whitening Convergence Rate of Coupling-based Normalizing Flows
NeurIPS 2022(2022)
摘要
Coupling-based normalizing flows (e.g. RealNVP) are a popular family of normalizing flow architectures that work surprisingly well in practice. This calls for theoretical understanding. Existing work shows that such flows weakly converge to arbitrary data distributions. However, they make no statement about the stricter convergence criterion used in practice, the maximum likelihood loss. For the first time, we make a quantitative statement about this kind of convergence: We prove that all coupling-based normalizing flows perform whitening of the data distribution (i.e. diagonalize the covariance matrix) and derive corresponding convergence bounds that show a linear convergence rate in the depth of the flow. Numerical experiments demonstrate the implications of our theory and point at open questions.
更多查看译文
关键词
normalizing flows,generative modeling,RealNVP,theory,maximum likelihood,kullback leibler divergence,invertible neural network,information theory,convergence,coupling block
AI 理解论文
溯源树
样例
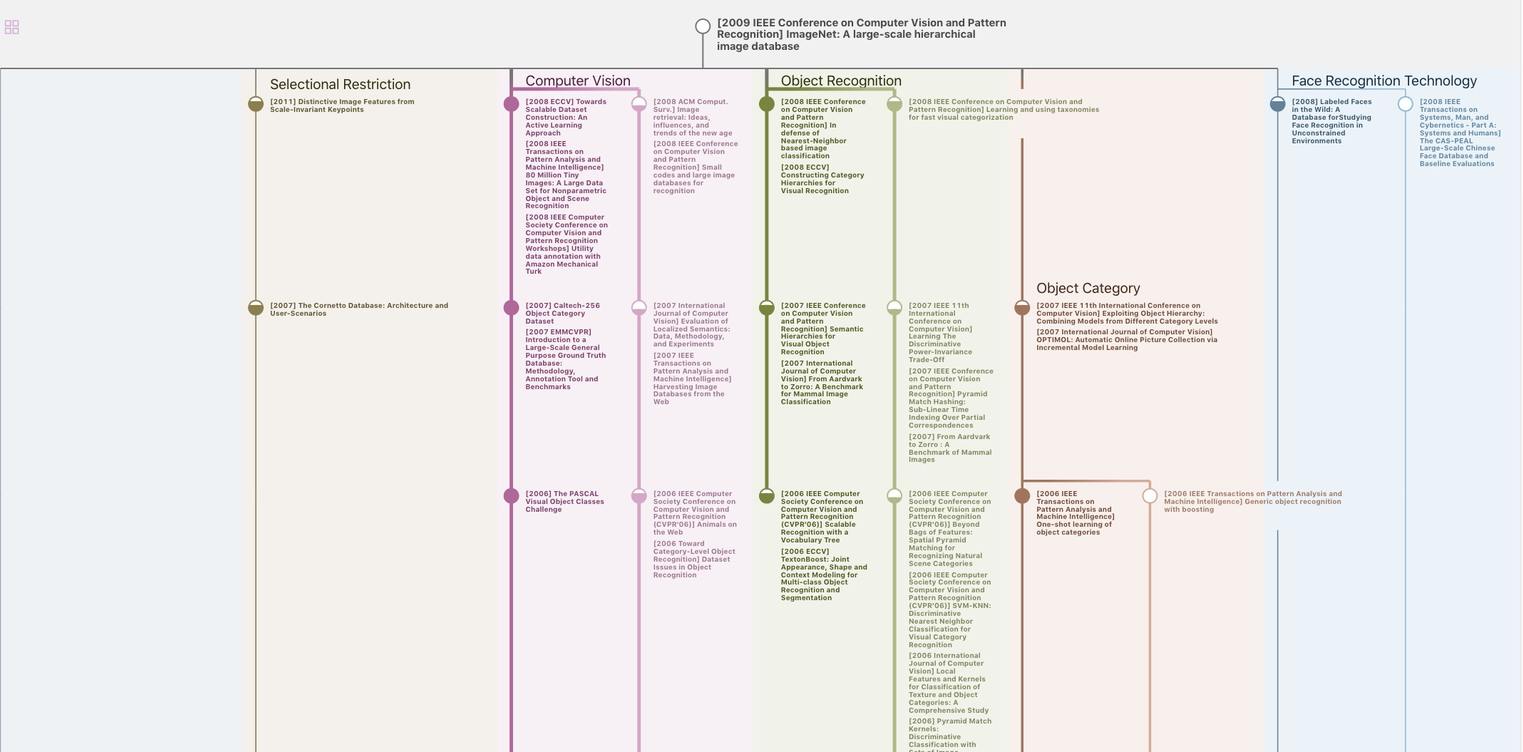
生成溯源树,研究论文发展脉络
Chat Paper
正在生成论文摘要