Improving Group Lasso for high-dimensional categorical data
arxiv(2022)
Abstract
Sparse modelling or model selection with categorical data is challenging even for a moderate number of variables, because one parameter is roughly needed to encode one category or level. The Group Lasso is a well known efficient algorithm for selection continuous or categorical variables, but all estimates related to a selected factor usually differ. Therefore, a fitted model may not be sparse, which makes the model interpretation difficult. To obtain a sparse solution of the Group Lasso we propose the following two-step procedure: first, we reduce data dimensionality using the Group Lasso; then to choose the final model we use an information criterion on a small family of models prepared by clustering levels of individual factors. We investigate selection correctness of the algorithm in a sparse high-dimensional scenario. We also test our method on synthetic as well as real datasets and show that it performs better than the state of the art algorithms with respect to the prediction accuracy or model dimension.
MoreTranslated text
Key words
group lasso,high-dimensional
AI Read Science
Must-Reading Tree
Example
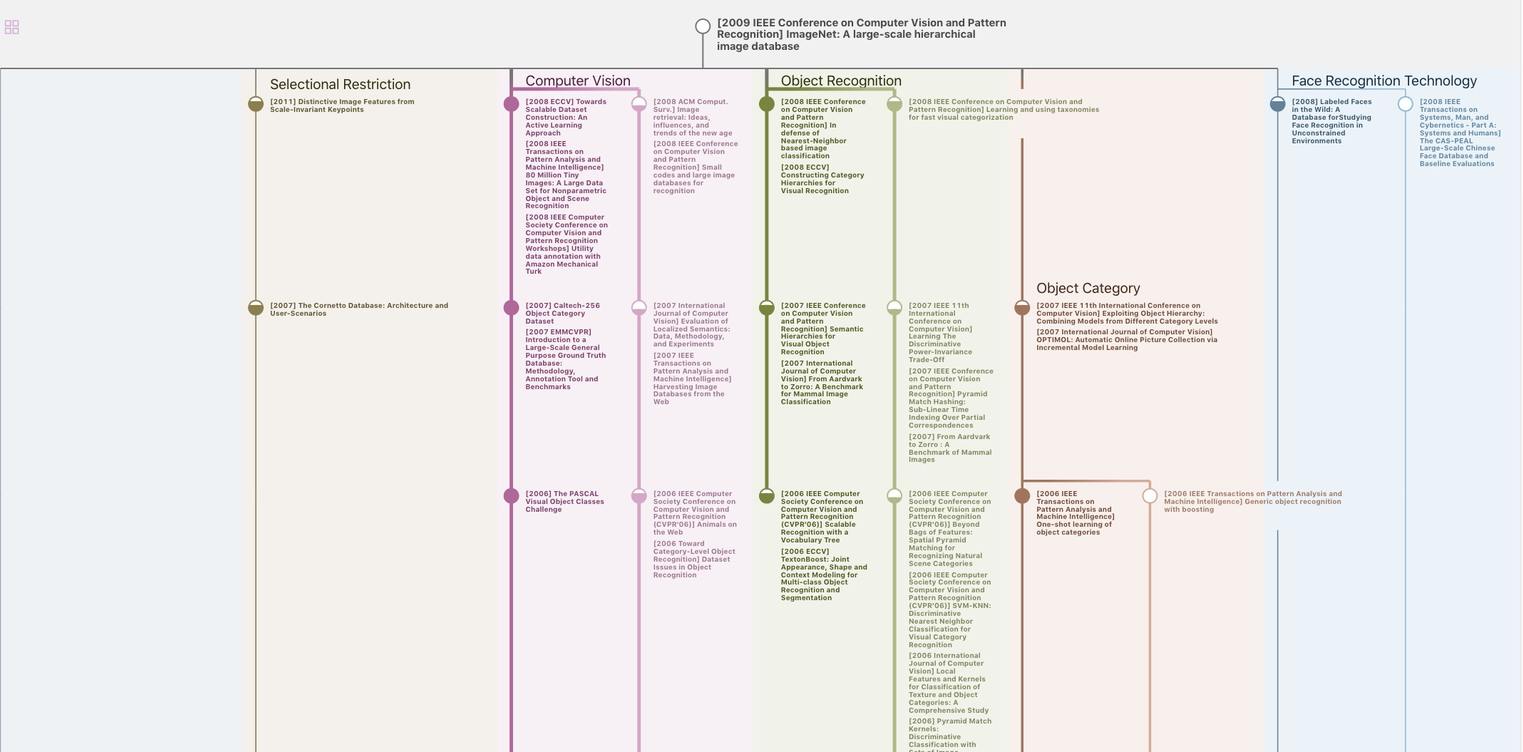
Generate MRT to find the research sequence of this paper
Chat Paper
Summary is being generated by the instructions you defined