Drought stress impact on the performance of deep convolutional neural networks for weed detection in Bahiagrass
Grass and Forage Science(2023)
Abstract
Machine vision-based weed detection relies on features such as plant colour, leaf texture, shape, and patterns. Drought stress in plants can alter leaf colour and morphological features, which may in turn affect the reliability of machine vision-based weed detection. The objective of this research was to evaluate the feasibility of using deep convolutional neural networks for the detection of Florida pusley (Richardia scabra L.) growing in drought stressed and unstressed bahiagrass (Paspalum natatum Flugge). The object detection neural networks you only look once (YOLO)v3, faster region-based convolutional network (Faster R-CNN), and variable filter net (VFNet) failed to effectively detect Florida pusley growing in drought stressed or unstressed bahiagrass, with F1 scores <= 0.54 in the testing dataset. Nevertheless, the use of the image classification neural networks AlexNet, GoogLeNet, and Visual Geometry Group-Network (VGGNet) was highly effective and achieved high (>= 0.97) F1 scores and recall values (>= 0.98) in detecting images containing Florida pusley growing in drought stressed or unstressed bahiagrass. Overall, these results demonstrated the effectiveness of using an image classification convolutional neural network for detecting Florida pusley in drought stressed or unstressed bahiagrass. These findings illustrate the broad applicability of these neural networks for weed detection.
MoreTranslated text
Key words
artificial intelligence,computer vision,deep learning,digital agriculture,weed detection
AI Read Science
Must-Reading Tree
Example
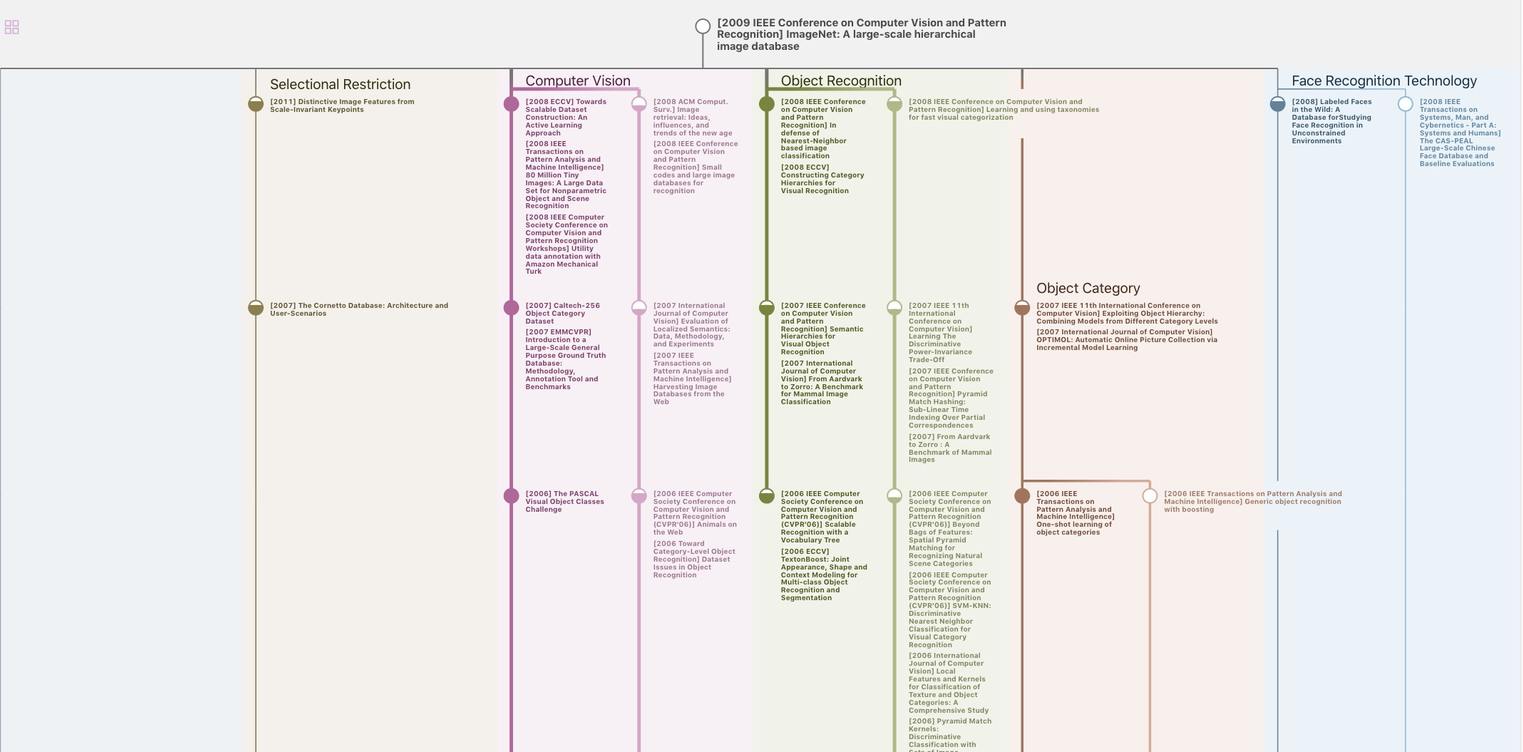
Generate MRT to find the research sequence of this paper
Chat Paper
Summary is being generated by the instructions you defined