Auto-Learning Correlation-Filter-Based Target State Estimation for Real-Time UAV Tracking
REMOTE SENSING(2022)
摘要
Most existing tracking methods based on discriminative correlation filters (DCFs) update the tracker every frame with a fixed learning rate. However, constantly adjusting the tracker can hardly handle the fickle target appearance in UAV tracking (e.g., undergoing partial occlusion, illumination variation, or deformation). To mitigate this, we propose a novel auto-learning correlation filter for UAV tracking, which fully exploits valuable information behind response maps for adaptive feedback updating. Concretely, we first introduce a principled target state estimation (TSE) criterion to reveal the confidence level of the tracking results. We suggest an auto-learning strategy with the TSE metric to update the tracker with adaptive learning rates. Based on the target state estimation, we further developed an innovative lost-and-found strategy to recognize and handle temporal target missing. Finally, we incorporated the TSE regularization term into the DCF objective function, which by alternating optimization iterations can efficiently solve without much computational cost. Extensive experiments on four widely-used UAV benchmarks have demonstrated the superiority of the proposed method compared to both DCF and deep-based trackers. Notably, ALCF achieved state-of-the-art performance on several benchmarks while running over 50 FPS on a single CPU. Code will be released soon.
更多查看译文
关键词
single object tracking,UAV tracking,correlation filters,target state estimation,auto-learning
AI 理解论文
溯源树
样例
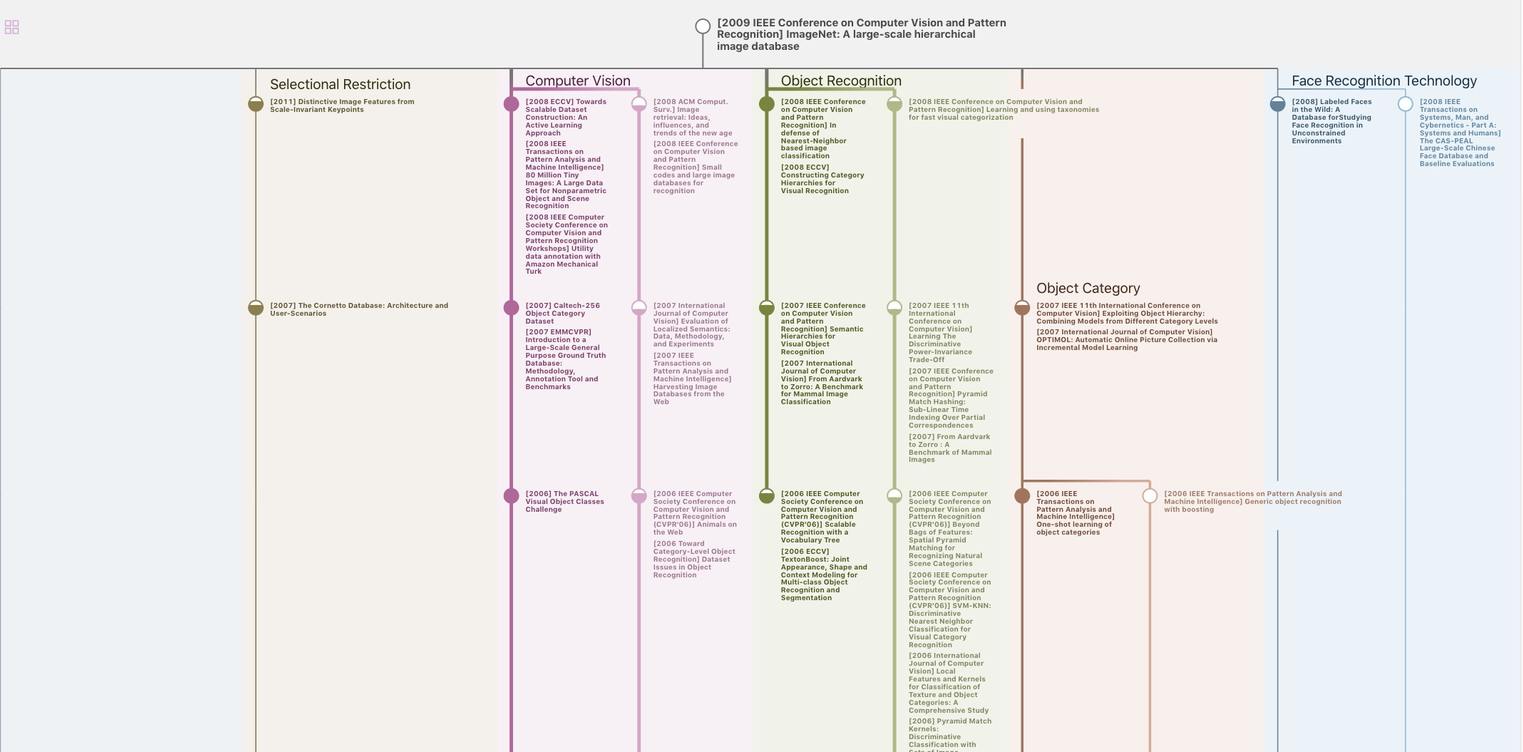
生成溯源树,研究论文发展脉络
Chat Paper
正在生成论文摘要