Click-Through Rate Prediction Network Based on User Behavior Sequences and Feature Interactions
Journal of Donghua University(English Edition)(2022)
Abstract
In recent years, deep learning has been widely applied in the fields of recommendation systems and click-through rate(CTR) prediction, and thus recommendation models incorporating deep learning have emerged. In addition, the design and implementation of recommendation models using information related to user behavior sequences is an important direction of current research in recommendation systems, and models calculate the likelihood of users clicking on target items based on their behavior sequence information. In order to explore the relationship between features, this paper improves and optimizes on the basis of deep interest network (DIN) proposed by Ali's team. Based on the user behavioral sequences information, the attentional factorization machine (AFM) is integrated to obtain richer and more accurate behavioral sequence information. In addition, this paper designs a new way of calculating attention weights, which uses the relationship between the cosine similarity of any two vectors and the absolute value of their modal length difference to measure their relevance degree. Thus, a novel deep learning CTR prediction mode is proposed, that is, the CTR prediction network based on user behavior sequence and feature interactions deep interest and machines network (DIMN). We conduct extensive comparison experiments on three public datasets and one private music dataset, which are more recognized in the industry, and the results show that the DIMN obtains a better performance compared with the classical CTR prediction model.
MoreTranslated text
Key words
click-through rate (CTR) prediction,behavior sequence,feature interaction,attention
AI Read Science
Must-Reading Tree
Example
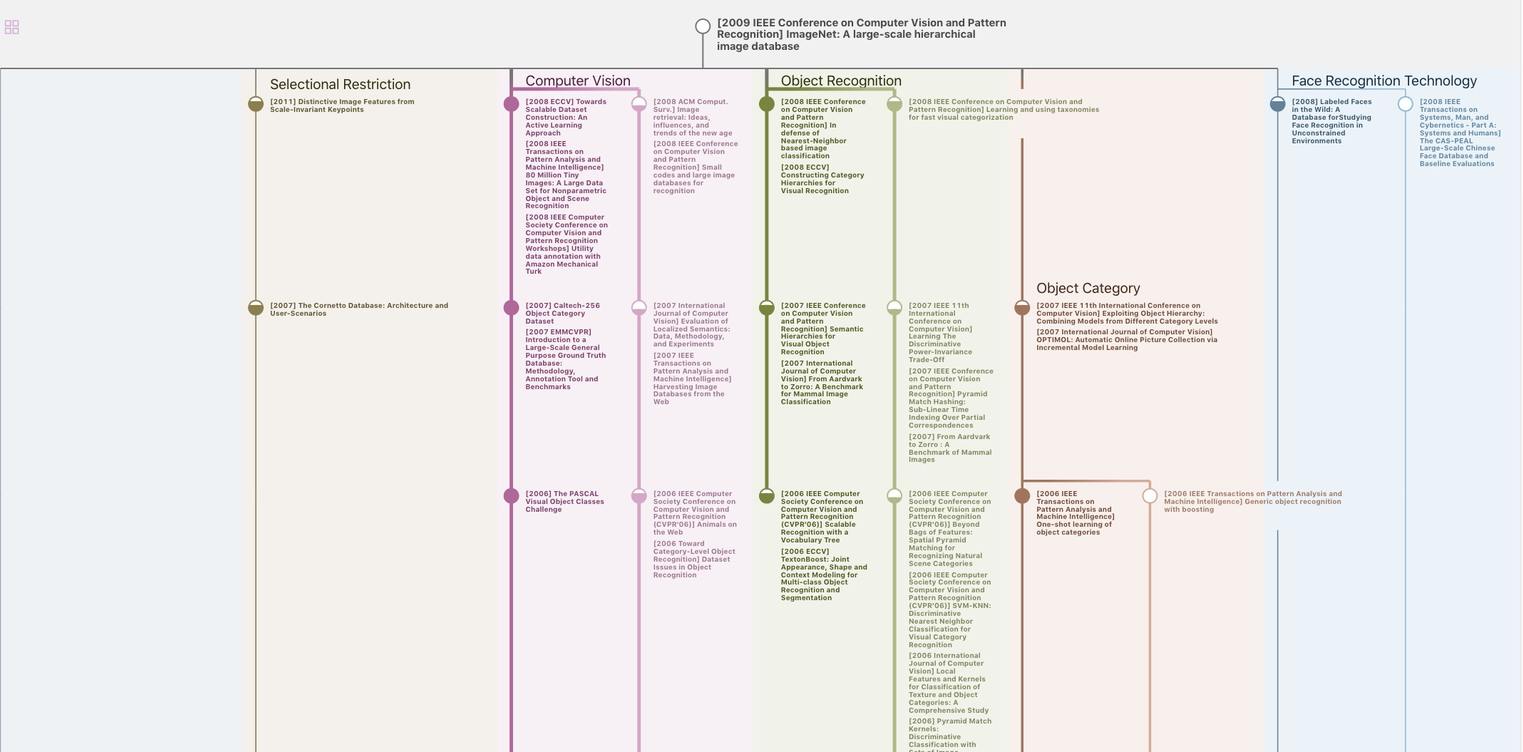
Generate MRT to find the research sequence of this paper
Chat Paper
Summary is being generated by the instructions you defined