Cascading Biases: Investigating the Effect of Heuristic Annotation Strategies on Data and Models
emnlp 2022(2022)
摘要
Cognitive psychologists have documented that humans use cognitive heuristics, or mental shortcuts, to make quick decisions while expending less effort. While performing annotation work on crowdsourcing platforms, we hypothesize that such heuristic use among annotators cascades on to data quality and model robustness. In this work, we study cognitive heuristic use in the context of annotating multiple-choice reading comprehension datasets. We propose tracking annotator heuristic traces, where we tangibly measure low-effort annotation strategies that could indicate usage of various cognitive heuristics. We find evidence that annotators might be using multiple such heuristics, based on correlations with a battery of psychological tests. Importantly, heuristic use among annotators determines data quality along several dimensions: (1) known biased models, such as partial input models, more easily solve examples authored by annotators that rate highly on heuristic use, (2) models trained on annotators scoring highly on heuristic use don't generalize as well, and (3) heuristic-seeking annotators tend to create qualitatively less challenging examples. Our findings suggest that tracking heuristic usage among annotators can potentially help with collecting challenging datasets and diagnosing model biases.
更多查看译文
关键词
heuristic annotation
AI 理解论文
溯源树
样例
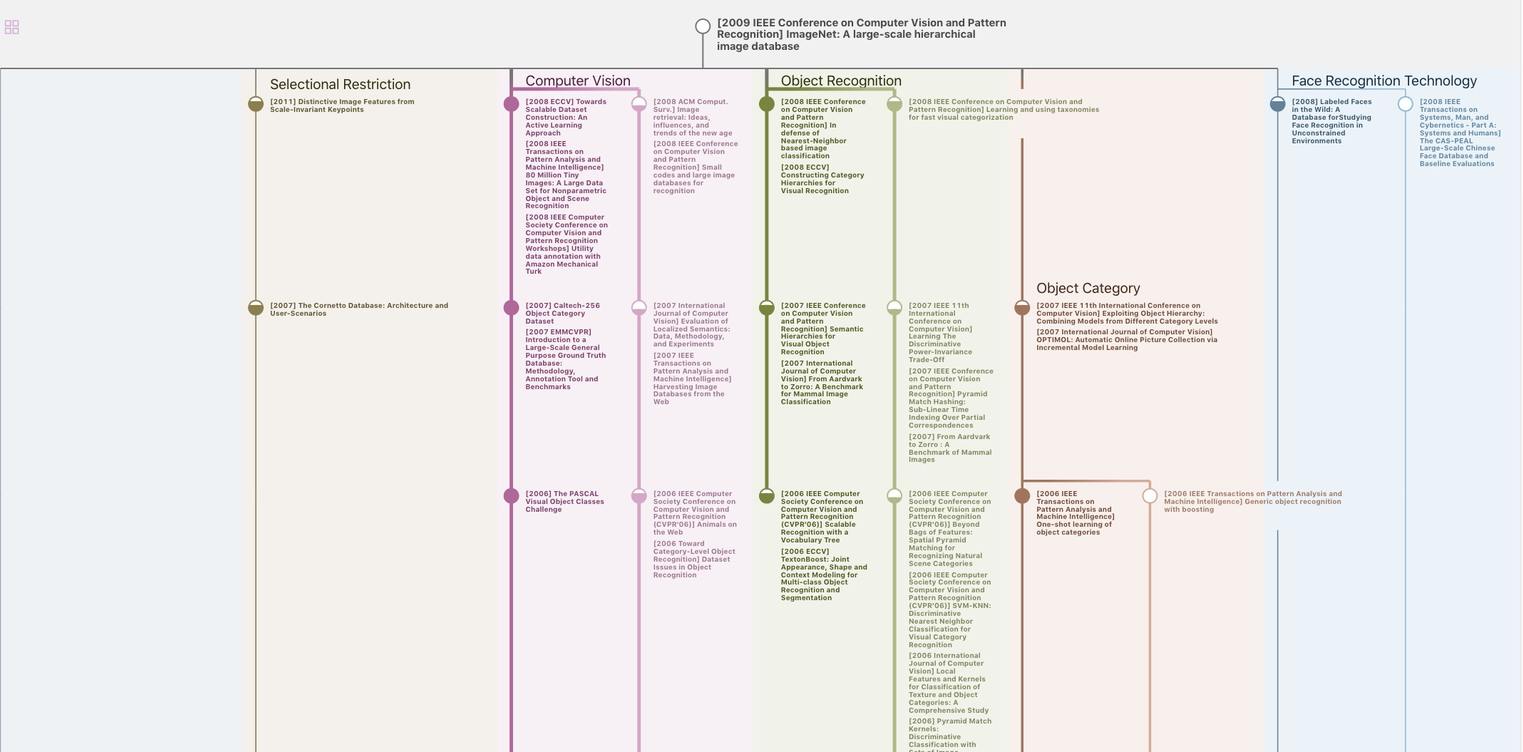
生成溯源树,研究论文发展脉络
Chat Paper
正在生成论文摘要