MM-Align: Learning Optimal Transport-based Alignment Dynamics for Fast and Accurate Inference on Missing Modality Sequences
emnlp 2022(2022)
Abstract
Existing multimodal tasks mostly target at the complete input modality setting, i.e., each modality is either complete or completely missing in both training and test sets. However, the randomly missing situations have still been underexplored. In this paper, we present a novel approach named MM-Align to address the missing-modality inference problem. Concretely, we propose 1) an alignment dynamics learning module based on the theory of optimal transport (OT) for indirect missing data imputation; 2) a denoising training algorithm to simultaneously enhance the imputation results and backbone network performance. Compared with previous methods which devote to reconstructing the missing inputs, MM-Align learns to capture and imitate the alignment dynamics between modality sequences. Results of comprehensive experiments on three datasets covering two multimodal tasks empirically demonstrate that our method can perform more accurate and faster inference and relieve overfitting under various missing conditions.
MoreTranslated text
AI Read Science
Must-Reading Tree
Example
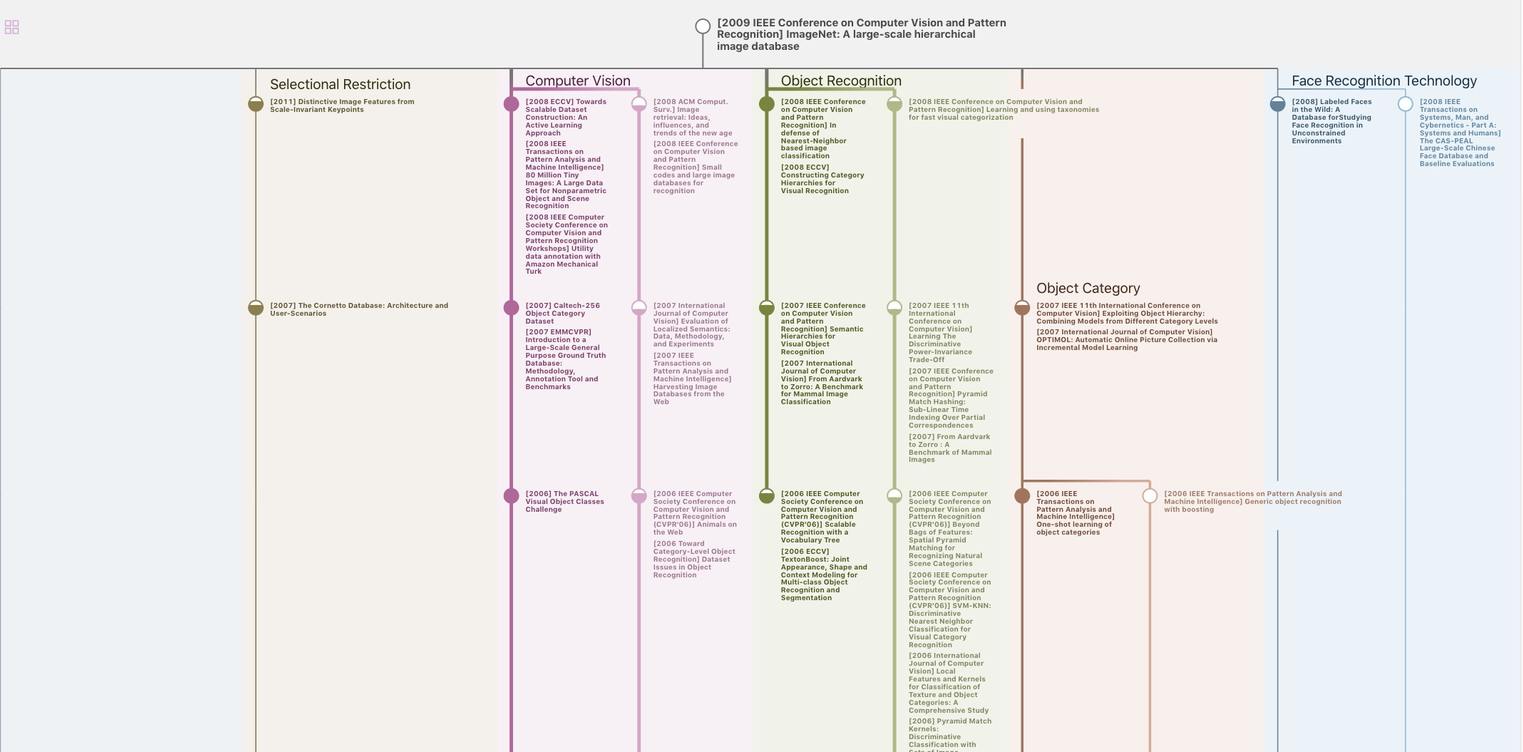
Generate MRT to find the research sequence of this paper
Chat Paper
Summary is being generated by the instructions you defined