An Improved RaftStereo Trained with A Mixed Dataset for the Robust Vision Challenge 2022
CoRR(2022)
Abstract
Stereo-matching is a fundamental problem in computer vision. Despite recent progress by deep learning, improving the robustness is ineluctable when deploying stereo-matching models to real-world applications. Different from the common practices, i.e., developing an elaborate model to achieve robustness, we argue that collecting multiple available datasets for training is a cheaper way to increase generalization ability. Specifically, this report presents an improved RaftStereo trained with a mixed dataset of seven public datasets for the robust vision challenge (denoted as iRaftStereo_RVC). When evaluated on the training sets of Middlebury, KITTI-2015, and ETH3D, the model outperforms its counterparts trained with only one dataset, such as the popular Sceneflow. After fine-tuning the pre-trained model on the three datasets of the challenge, it ranks at 2nd place on the stereo leaderboard, demonstrating the benefits of mixed dataset pre-training.
MoreTranslated text
Key words
improved raftstereo,vision challenge,robust
AI Read Science
Must-Reading Tree
Example
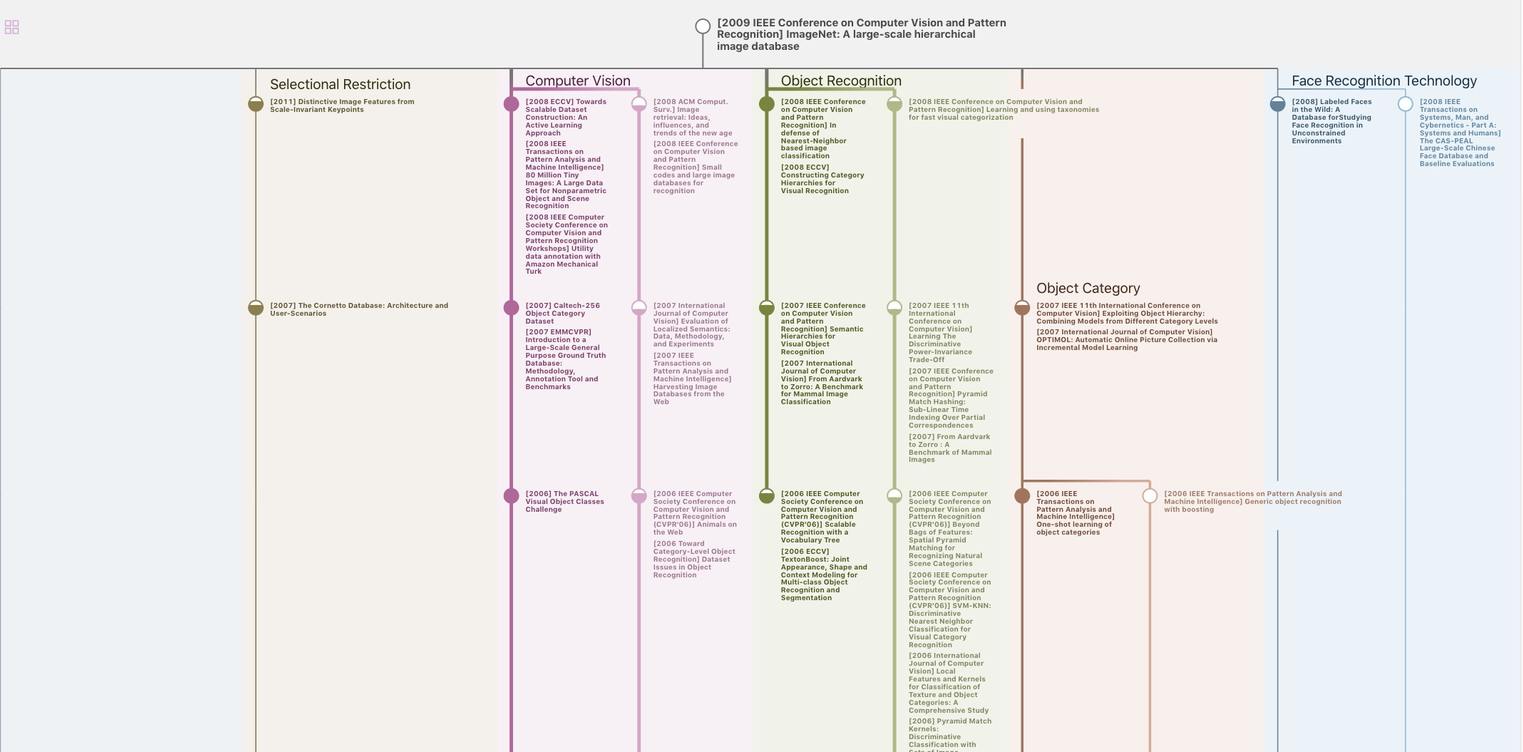
Generate MRT to find the research sequence of this paper
Chat Paper
Summary is being generated by the instructions you defined