Accumulated Trivial Attention Matters in Vision Transformers on Small Datasets
WACV(2023)
Abstract
Vision Transformers has demonstrated competitive performance on computer vision tasks benefiting from their ability to capture long-range dependencies with multi-head self-attention modules and multi-layer perceptron. However, calculating global attention brings another disadvantage compared with convolutional neural networks, i.e. requiring much more data and computations to converge, which makes it difficult to generalize well on small datasets, which is common in practical applications. Previous works are either focusing on transferring knowledge from large datasets or adjusting the structure for small datasets. After carefully examining the self-attention modules, we discover that the number of trivial attention weights is far greater than the important ones and the accumulated trivial weights are dominating the attention in Vision Transformers due to their large quantity, which is not handled by the attention itself. This will cover useful non-trivial attention and harm the performance when trivial attention includes more noise, e.g. in shallow layers for some backbones. To solve this issue, we proposed to divide attention weights into trivial and non-trivial ones by thresholds, then Suppressing Accumulated Trivial Attention (SATA) weights by proposed Trivial WeIghts Suppression Transformation (TWIST) to reduce attention noise. Extensive experiments on CIFAR-100 and Tiny-ImageNet datasets show that our suppressing method boosts the accuracy of Vision Transformers by up to 2.3%. Code is available at https://github.com/xiangyu8/SATA.
MoreTranslated text
Key words
Algorithms: Machine learning architectures,formulations,and algorithms (including transfer)
AI Read Science
Must-Reading Tree
Example
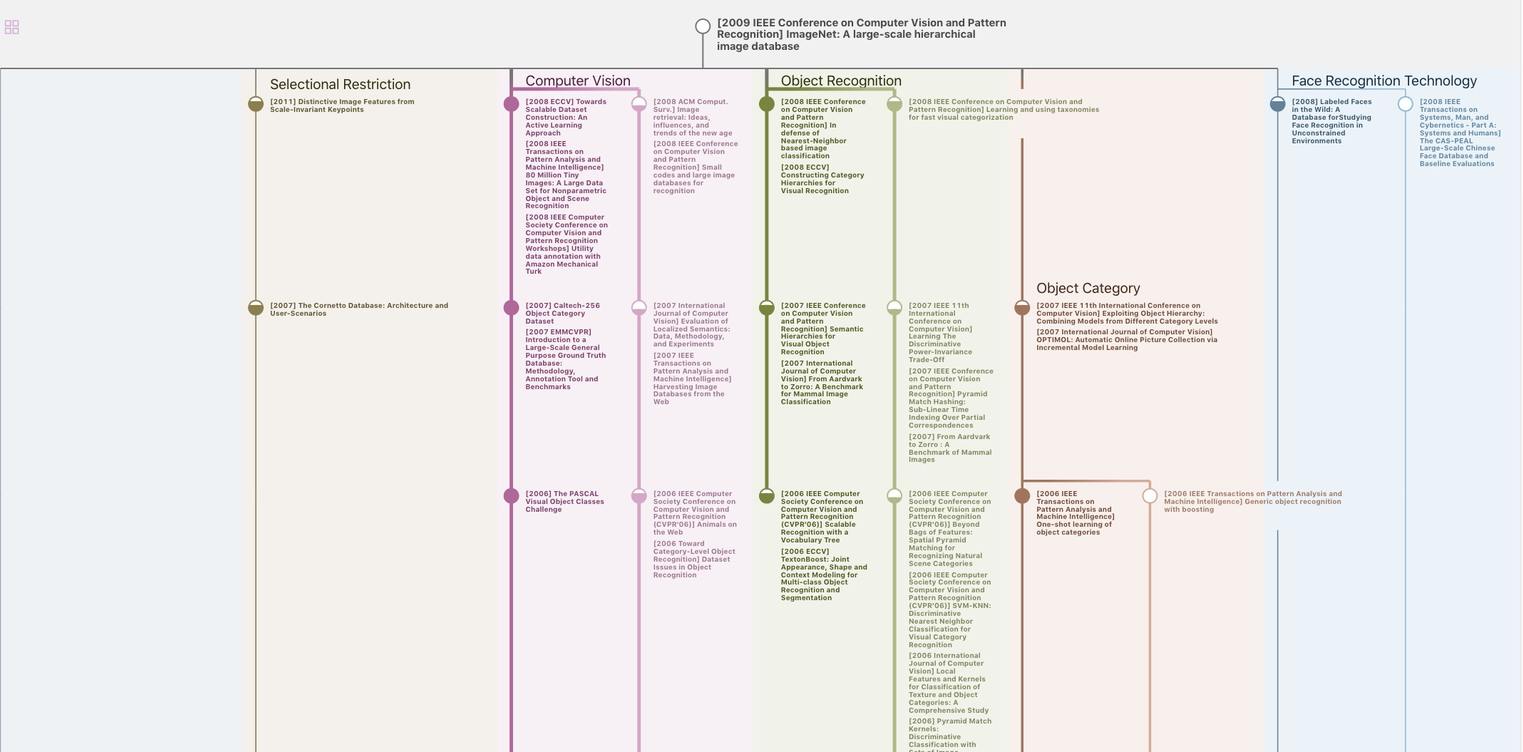
Generate MRT to find the research sequence of this paper
Chat Paper
Summary is being generated by the instructions you defined