Augmentation by Counterfactual Explanation Fixing an Overconfident Classifier
WACV(2023)
Abstract
A highly accurate but overconfident model is ill-suited for deployment in critical applications such as healthcare and autonomous driving. The classification outcome should reflect a high uncertainty on ambiguous in-distribution samples that lie close to the decision boundary. The model should also refrain from making overconfident decisions on samples that lie far outside its training distribution, far-out-of-distribution (far-OOD), or on unseen samples from novel classes that lie near its training distribution (near-OOD). This paper proposes an application of counterfactual explanations in fixing an over-confident classifier. Specifically, we propose to fine-tune a given pre-trained classifier using augmentations from a counterfactual explainer (ACE) to fix its uncertainty characteristics while retaining its predictive performance. We perform extensive experiments with detecting far-OOD, near-OOD, and ambiguous samples. Our empirical results show that the revised model have improved uncertainty measures, and its performance is competitive to the state-of-the-art methods.
MoreTranslated text
Key words
Algorithms: Explainable,fair,accountable,privacy-preserving,ethical computer vision,Adversarial learning,adversarial attack and defense methods
AI Read Science
Must-Reading Tree
Example
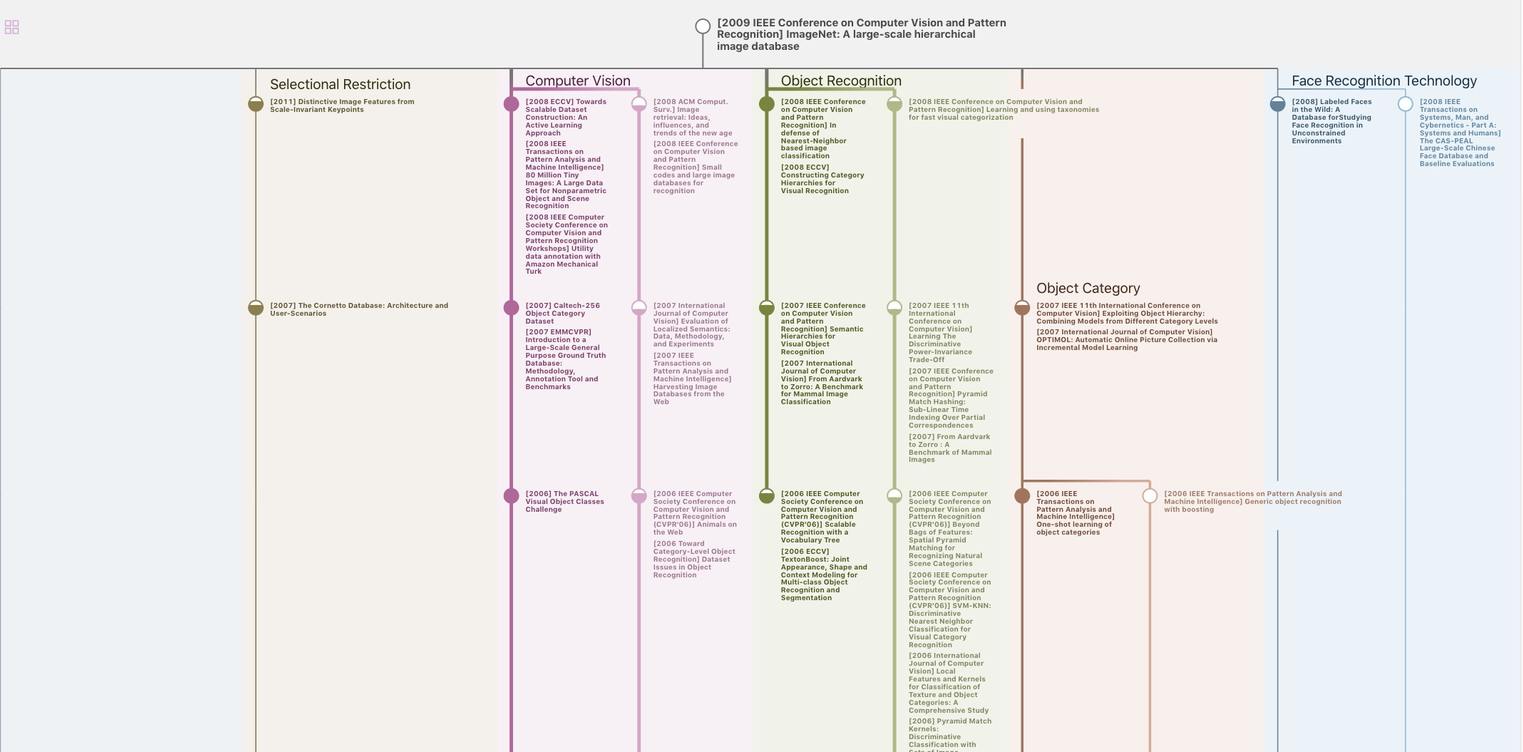
Generate MRT to find the research sequence of this paper
Chat Paper
Summary is being generated by the instructions you defined