Modelling Multi-relations for Convolutional-based Knowledge Graph Embedding
Procedia Computer Science(2022)
摘要
Representation learning of knowledge graphs aims to embed entities and relations into low-dimensional vectors. Most existing works only consider the direct relations or paths between an entity pair. It is considered that such approaches disconnect the semantic connection of multi-relations between an entity pair, and we propose a convolutional and multi-relational representation learning model, ConvMR. The proposed ConvMR model addresses the multi-relation issue in two aspects: (1) Encoding the multi-relations between an entity pair into a unified vector that maintains the semantic connection. (2) Since not all relations are necessary while joining multi-relations, we propose an attention-based relation encoder to automatically assign weights to different relations based on semantic hierarchy. Experimental results on two popular datasets, FB15k-237 and WN18RR, achieved consistent improvements on the mean rank. We also found that ConvMR is efficient to deal with less frequent entities.
更多查看译文
关键词
Knowledge Graph Embedding,Multi-relation,Convolution,Representation learning
AI 理解论文
溯源树
样例
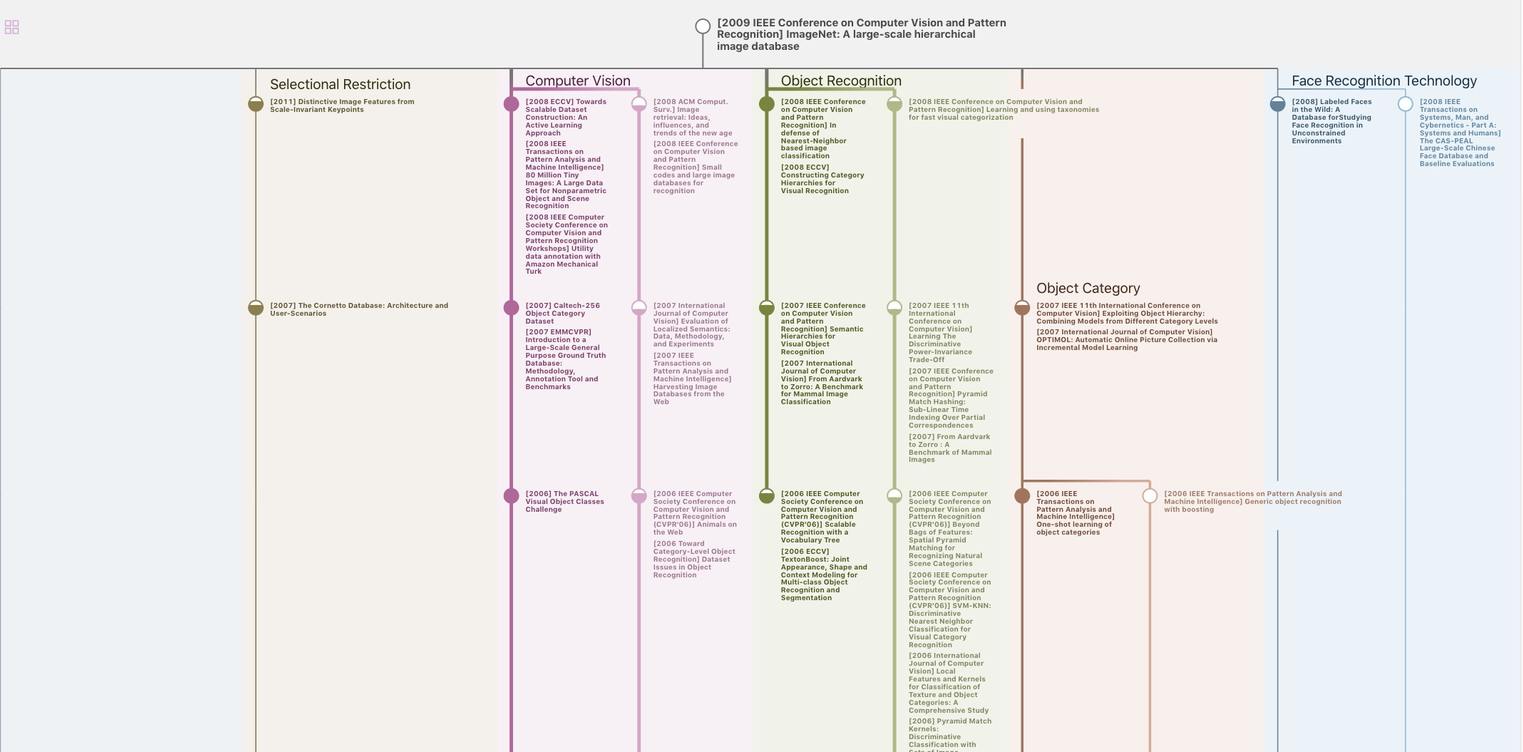
生成溯源树,研究论文发展脉络
Chat Paper
正在生成论文摘要