Principal component analysis of infinite variance functional data
Journal of Multivariate Analysis(2023)
摘要
Principal Components Analysis is a widely used approach of multivariate analysis. Over the past 30 years, it has gained renewed attention in the context of functional data, chiefly as a commonly used tool for dimension reduction or feature extraction. Consequently, a large body of statistical theory has been developed to justify its application in various contexts. This theory focuses on the convergence of sample PCs to their population counterparts in a multitude of statistical models and under diverse data collection assumptions. What such results have in common is the assumption that the population covariance operator exists.This paper is concerned with multivariate and functional data that have infinite variance and, consequently, for which the population covariance operator is not defined. However, the sample covariance operator and its eigenfunctions are always defined. It has been unknown what the asymptotic behavior of these important statistics is in the context of infinite variance multivariate or functional data. We derive suitable large sample theory. In particular, we specify normalizing sequences and conditions for suitably defined consistency. We study multivariate models in which explicit limits can be derived. These examples show that definitions, results and intuition developed for multivariate and functional data with finite variance need not apply in the setting we consider.
更多查看译文
关键词
primary,secondary
AI 理解论文
溯源树
样例
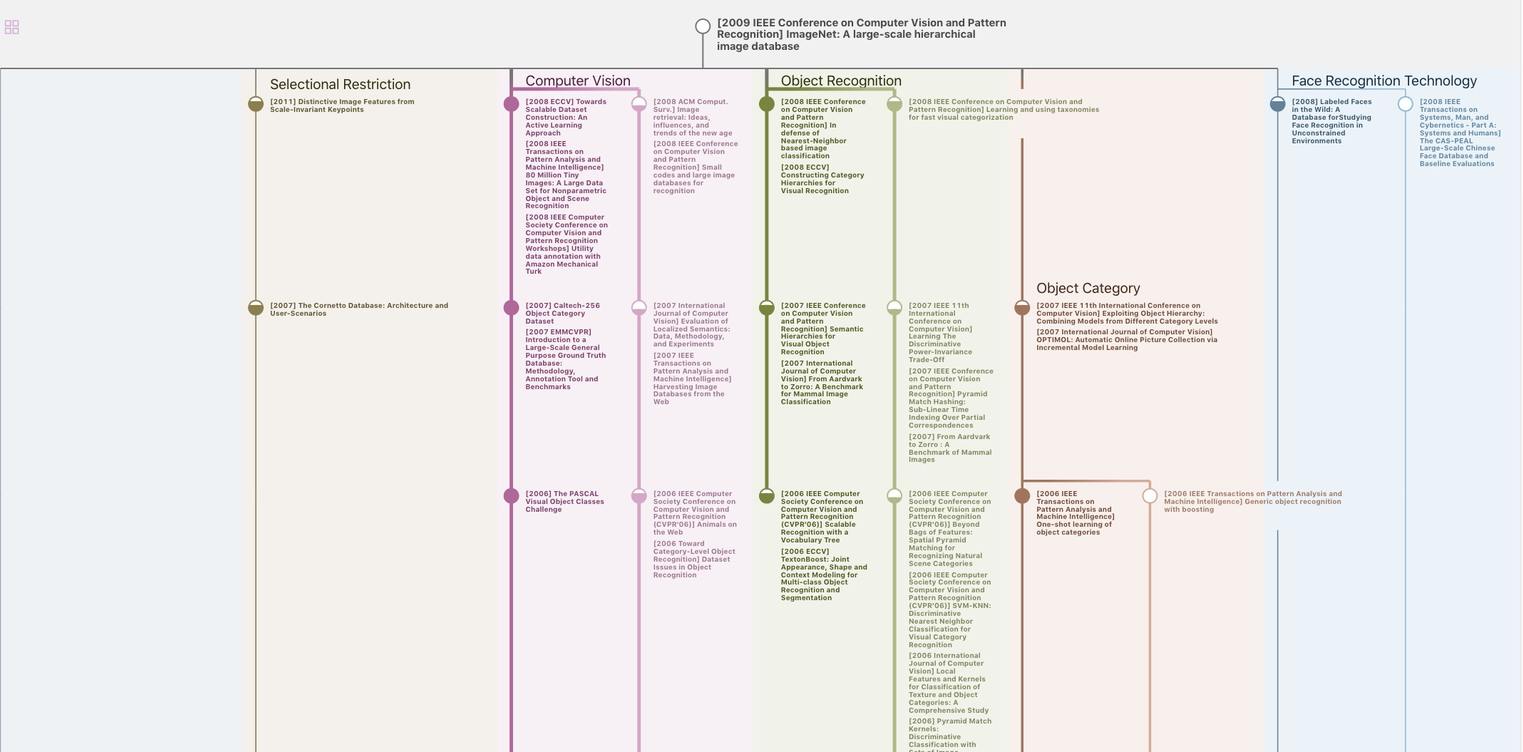
生成溯源树,研究论文发展脉络
Chat Paper
正在生成论文摘要