High-Resolution 3D Magnetic Resonance Fingerprinting With a Graph Convolutional Network
IEEE Transactions on Medical Imaging(2023)
摘要
Magnetic resonance fingerprinting (MRF) is a novel quantitative imaging framework for rapid and simultaneous quantification of multiple tissue properties. 3D MRF allows higher through-plane resolution, but the acquisition process is slow when whole-brain coverage is needed. Existing methods for acceleration mainly rely on GRAPPA for k-space interpolation in the partition-encoding direction, limiting the acceleration factor to 2 or 3. In this work, we replace GRAPPA with a deep learning approach for accurate tissue quantification with greater acceleration. Specifically, a graph convolution network (GCN) is developed to cater to the non-Cartesian spiral sampling trajectories typical in MRF acquisition. The GCN maintains high quantification accuracy with up to 6-fold acceleration and allows 1mm isotropic resolution whole-brain 3D MRF data to be acquired in 3min and submillimeter 3D MRF (0.8mm) in 5min, greatly improving the feasibility of MRF in clinical settings.
更多查看译文
关键词
3D magnetic resonance fingerprinting (MRF),graph convolution,GRAPPA,k-space interpolation
AI 理解论文
溯源树
样例
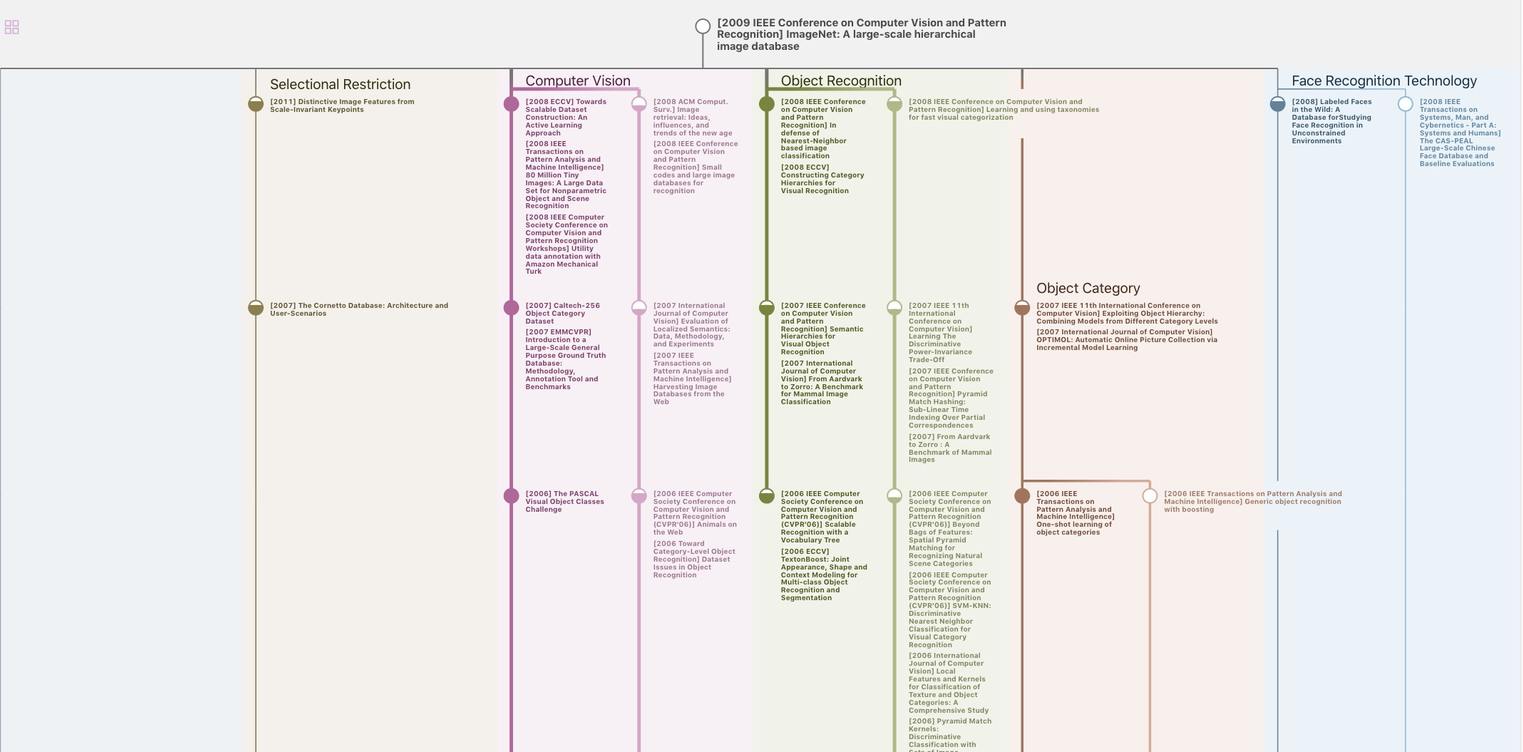
生成溯源树,研究论文发展脉络
Chat Paper
正在生成论文摘要