Brain MRI tumour classification using quantum classical convolutional neural net architecture
Neural Comput. Appl.(2022)
摘要
The use of quantum machines leverages the performance of classical machines in many aspects of solving real-world problems. Classification of a brain MR image for detection of tumour regions is a widely performed diagnostic step when it comes to working with brain images. Classical machine learning methods and/or conventional deep learning architectures including convolutional nets are commonly used for the classification of images. With larger network size, training the model becomes a challenging task to undertake. Quantum algorithms are beneficial in optimizing the performance of classical algorithms by incorporating intrinsic properties of quantum bits. In this paper, a novel Quantum Classical ConvNet architecture (QCCNN) is proposed for a binary class classification of brain MR images for detection of tumour regions in the human brain. The underlying idea is to encode data into quantum states enabling a faster extraction of information followed by using the information to distinguish the class of data. The reliability and the robustness of the proposed architecture are highlighted by the results obtained by the classifier. With technological advancements in quantum computers in the future (more qubits and less noise), the performance of the approach can be further improved. The presented model is tested on various datasets (Brats 2013, Harvard Med School, private dataset) and on quantitative evaluation with standard metrics, and the robustness of the classifier is verified. The proposed QCCNN model achieves accuracies in the range of 97.5–98.72% on different datasets which defend the ability of the presented architecture in detecting and classifying brain tumours.
更多查看译文
关键词
Hybrid quantum algorithm,Quantum net,Brain tumour classification,Convolutional neural net
AI 理解论文
溯源树
样例
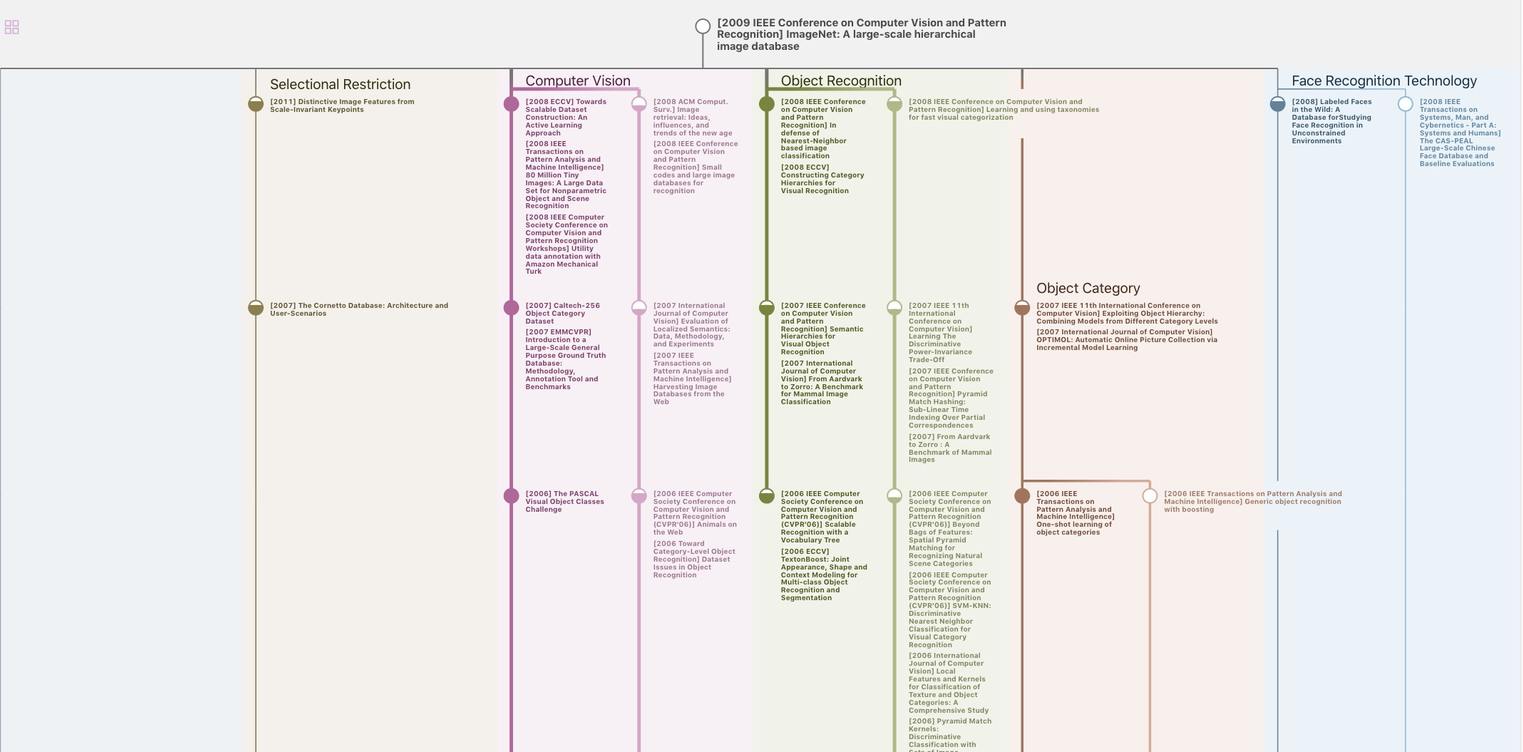
生成溯源树,研究论文发展脉络
Chat Paper
正在生成论文摘要