A distributed sparse logistic regression with L_1/2 regularization for microarray biomarker discovery in cancer classification
Soft Comput.(2022)
摘要
Microarray is a high-throughput sequencing technology, which can be used to classify cancer types and select the highly relevant cancer biomarkers (i.e., genes). To improve the availability of ever-increasing microarray data, data-integrative analysis becomes a hot research direction. However, the complexity of gene expression data still brings many challenges to the data integration methods: (1) the relevant biomarker selection in multiple high-dimensional datasets; (2) the batch effects between datasets; (3) the high noise in features and samples; (4) the large-scale data analysis with high computational cost. To overcome these challenges, we propose a novel Distribute-based Biological data-Integrative Analysis model—DBIA. DBIA is based on the L_1/2 regularized logistic regression ( L_1/2 LR) model and the alternating direction multiplication algorithm (ADMM) for data integration. The regularization model is an effective method for selecting latent cancer-relevant genes and improving the accuracy of cancer classification. Moreover, we adopt the L_1/2 LR model to reduce the noise and dimensionality of the data. ADMM is employed to reduce the batch effects between datasets, analyze multiple datasets in parallel, and save the computational cost of large-scale data analysis. Experimental results on the simulation and real-world datasets demonstrate that DBIA achieves the good prediction performance with a shorter time, lower hardware requirements, and strong robustness. The genes selected by DBIA have a certain biological significance.
更多查看译文
关键词
Microarray data integration,L_1/2 regularized logistic regression,ADMM algorithm,Cancer classification,Gene selection
AI 理解论文
溯源树
样例
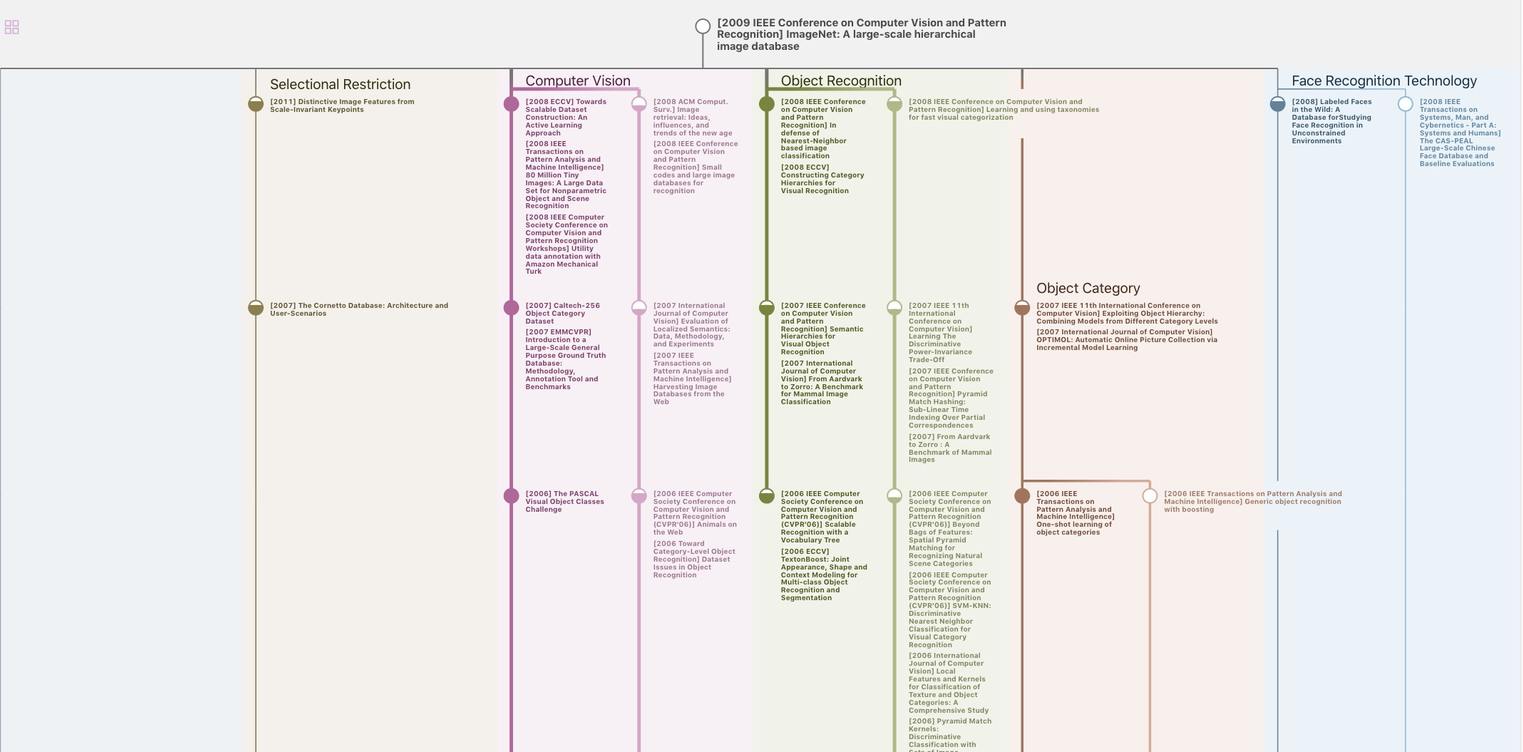
生成溯源树,研究论文发展脉络
Chat Paper
正在生成论文摘要