Unsupervised feature selection guided by orthogonal representation of feature space.
Neurocomputing(2023)
Abstract
Feature selection has been an outstanding strategy in eliminating redundant and inefficient features in high-dimensional data. This paper introduces a novel unsupervised feature selection based on the matrix factorization, namely Unsupervised Feature Selection Guided by Orthogonal Representation (UFGOR). The orthogonality between a pair of variables refers to a specific case of linear independence such that they are perfectly uncorrelated. Motivated by the benefits of the orthogonality concept, the proposed UFGOR method is established based on the distance between the selected feature set and an orthogonal set corresponding to the whole feature space. Moreover, this orthogonal set is generated via QR-matrix factorization over the whole features and is employed as the compact representation of data matrix. In the next step, an unsupervised feature selection method is performed through the matrix factorization of the generated orthogonal set. Additionally, a dual-correlation model is utilized in the objective func-tion of UFGOR to simultaneously consider both the local correlation in a set of selected features and the global correlation among the samples of a data. A detailed convergence analysis in line with an effec-tive iterative algorithm proposed for the UFGOR method is also given. Numerical experiments on several real-world datasets illustrate the superior efficiency of our approach in comparison with some state-of-the-art unsupervised feature selection methods.(c) 2022 Elsevier B.V. All rights reserved.
MoreTranslated text
Key words
Dimension reduction,Feature selection,Orthogonality,Correlation,Redundancy
AI Read Science
Must-Reading Tree
Example
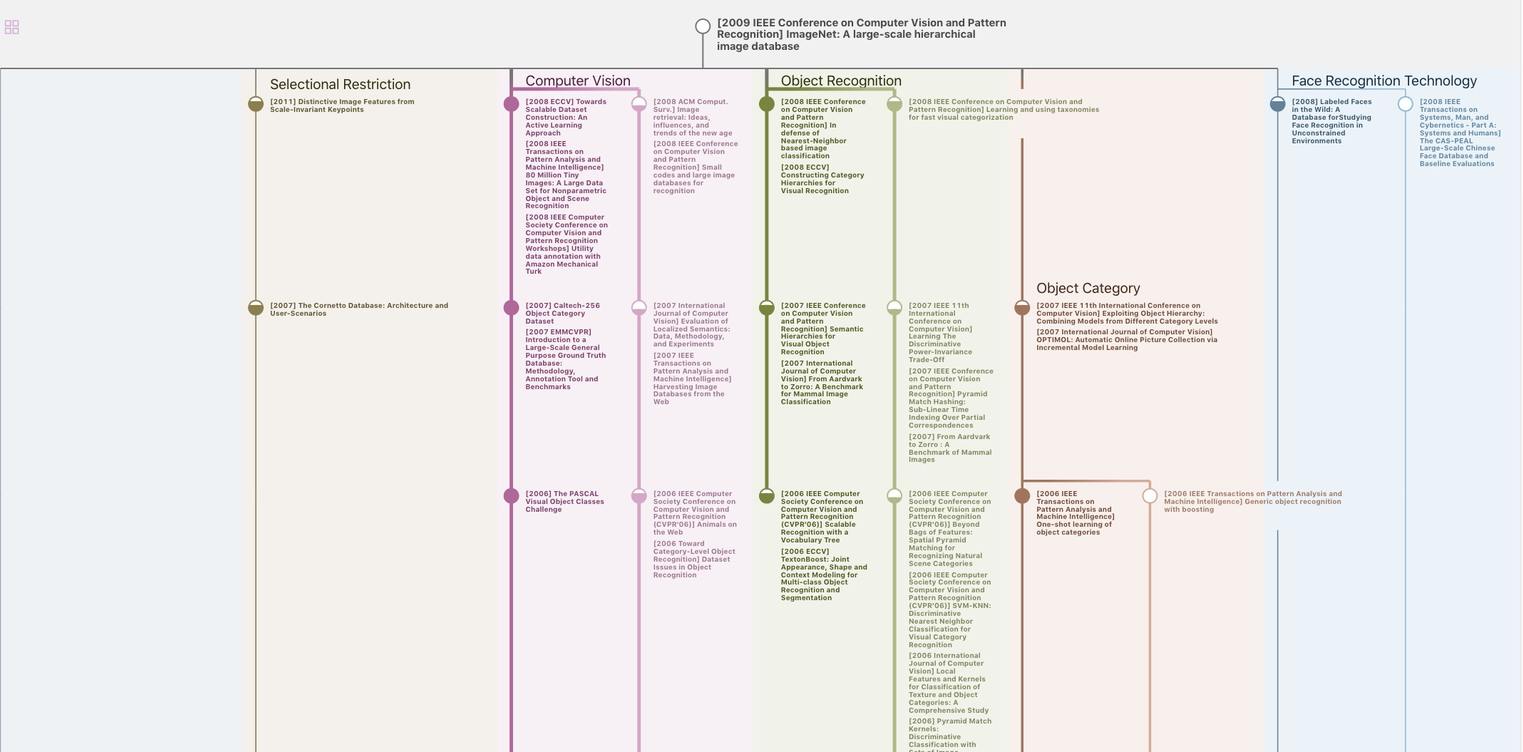
Generate MRT to find the research sequence of this paper
Chat Paper
Summary is being generated by the instructions you defined