Convolutional networks and transformers for intelligent road tunnel investigations
Computers & Structures(2023)
摘要
Visual inspections do not provide a reliable and objective assessment of the conservation state of road tunnels. Although direct tests might represent a valid survey approach, they would often lead to pro-hibitive costs if performed extensively. Therefore, indirect techniques, such as ground-penetrating radar (GPR), have become fundamental to supporting limited direct tests. The analysis of the GPR tunnel linings profiles is mainly hand-operated. It permits the detection of various tunnel linings defects, characterizing a tunnel's global health state. In the present work, the authors developed an artificial intelligence (AI) based automatic road tunnel defects hierarchical classification framework to improve the efficiency of this powerful indirect surveying method. Adopting the most recent tools in image processing provided by the deep learning (DL) community, the authors proposed a convolutional neural network (CNN) with the acknowledged ResNet-50 architecture, initialized through the transfer learning method. For the sake of comparisons, the authors also adopted the state-of-art convolutional EfficientNet architecture. To fur-ther improve the proposed framework, the authors investigated how the bidimensional Fourier transform applied as a preprocessing procedure could affect the classification performances of the ResNet-50 model. Finally, to further enhance the classification performance, the state-of-art neural vision transformer (ViT) architecture has been adopted with the transfer learning approach to the currently proposed defects clas-sification framework. (c) 2022 Elsevier Ltd. All rights reserved.
更多查看译文
关键词
Deep learning,Vision Transformers,Road tunnels,Fourier transform,Convolutional Neural Network,Structural Health Monitoring,Ground Penetrating Radar
AI 理解论文
溯源树
样例
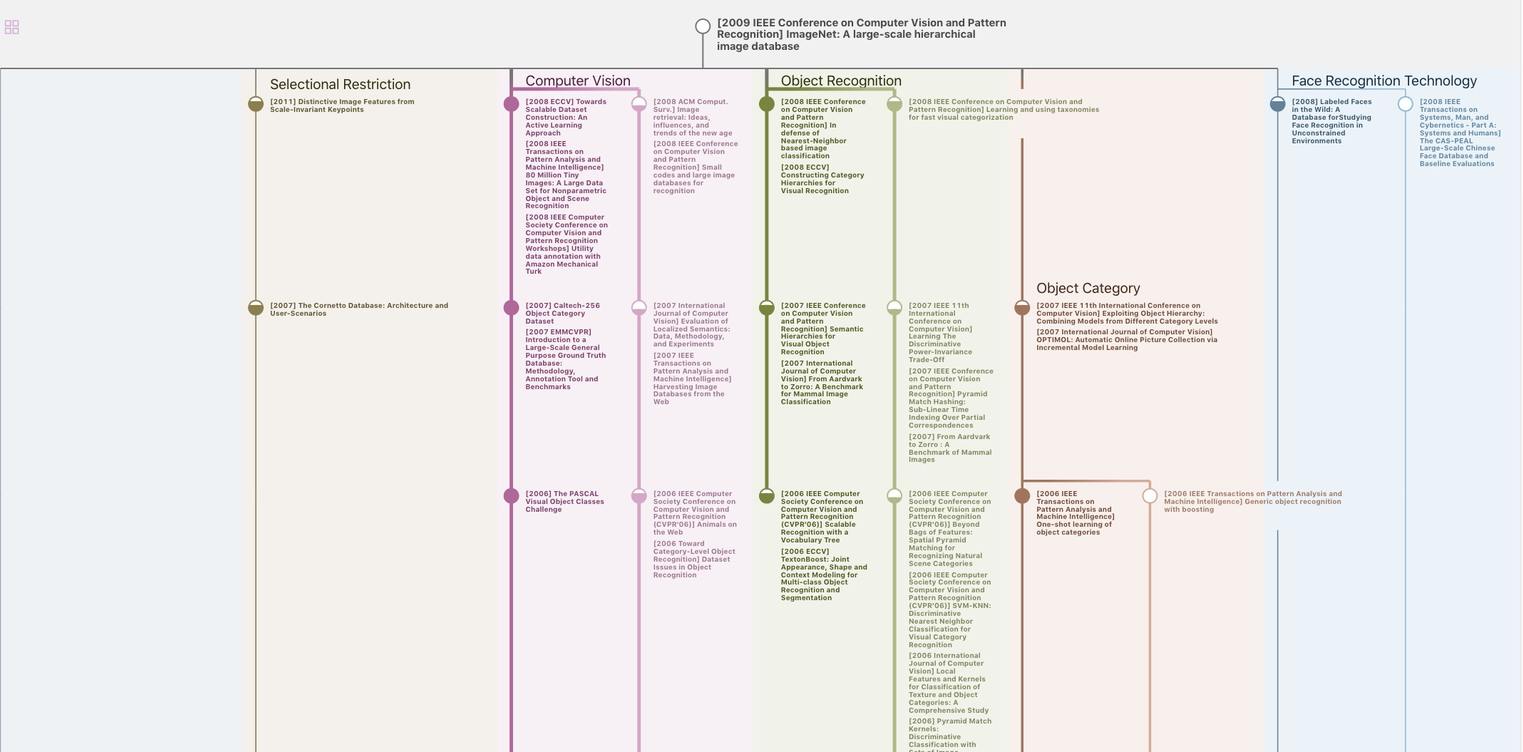
生成溯源树,研究论文发展脉络
Chat Paper
正在生成论文摘要