Extraction of the plastic properties of metallic materials from scratch tests using deep learning
Mechanics of Materials(2022)
Abstract
Powered by machine learning and computer technology, neural networks have opened new paths for solving engineering problems. In this paper, the plastic parameters, i.e., the yield stress and strain hardening index, of metallic materials are extracted from scratch tests using deep learning methods. Using a dataset generated by finite element simulations, three network models, i.e., the classical multi-output multi-layer perceptron (MLP), a single-target approach (ST-MLP) and the parameter sharing-based deep network (DMTR), are adopted to determine the relationship between scratch responses and plastic parameters. According to the test dataset re-sults, the DMTR performs better than the MLP and ST-MLP. The trained DMTR is verified by comparing the plastic parameters of 18CrNiMo7-6 alloy steel, 304 stainless steel, and brass obtained from scratch tests with those under tension. This work is expected to provide an alternative method for determining the plastic pa-rameters of metallic materials.
MoreTranslated text
Key words
Plastic properties,Scratch test,Deep learning,Finite element simulation,Multi -target regression,Neural networks
AI Read Science
Must-Reading Tree
Example
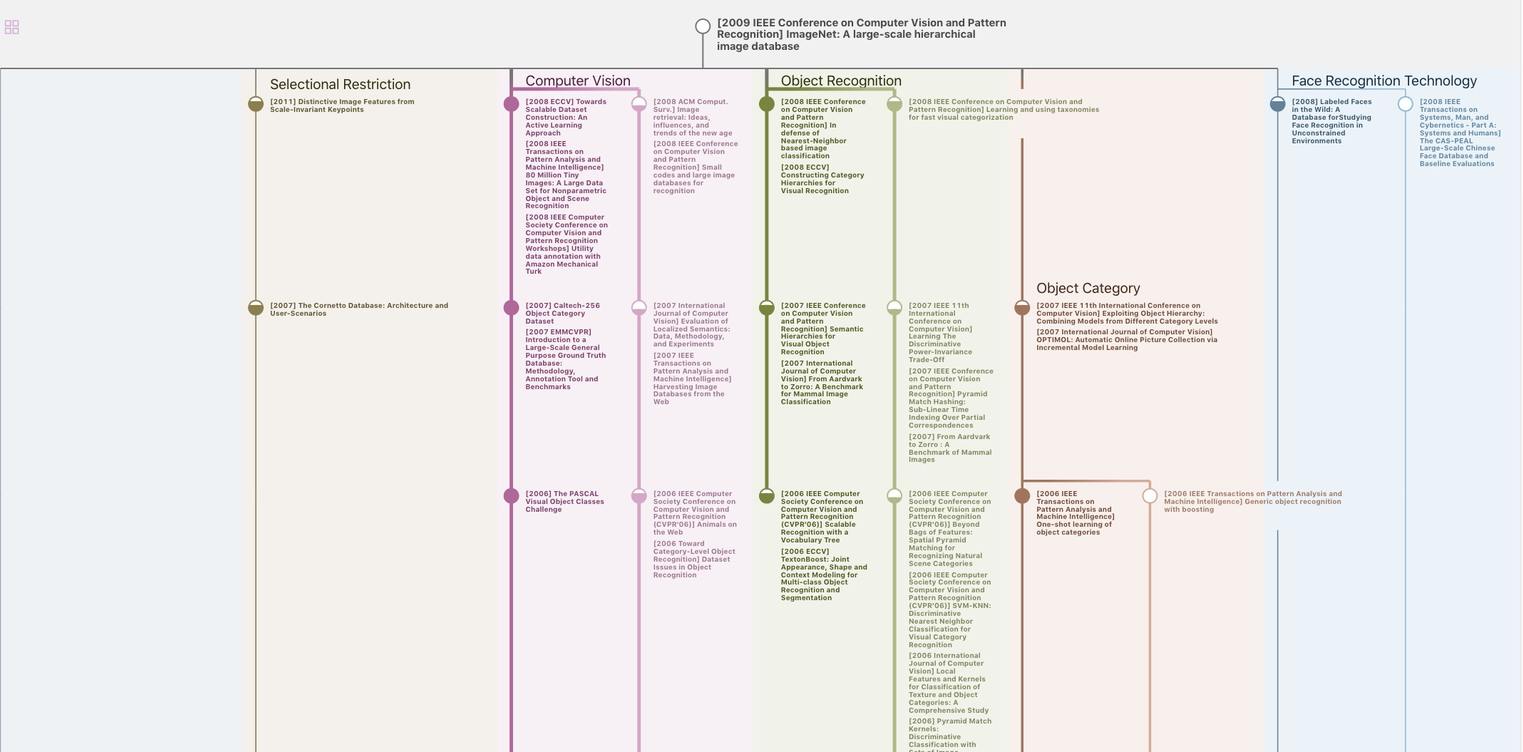
Generate MRT to find the research sequence of this paper
Chat Paper
Summary is being generated by the instructions you defined