Explainable Boosting Machine for Choosing Radiation Dose-Volume Constraints on Cardio-Pulmonary Substructures Associated with Overall Survival in the NRG Oncology RTOG 0617 Clinical Trial
International Journal of Radiation Oncology*Biology*Physics(2022)
Abstract
Purpose/Objective(s)
Black box (BB) machine learning models are frequently used for decision making in healthcare. Although posthoc model-agnostic explanations can improve the BB model's interpretability, they are often unreliable. Explainable boosting machine (EBM) is a glass box model that allows for model-specific interpretation of each feature's contribution to outcome prediction. We used EBM for choosing radiation dose-volume constraints (DVCs) on cardio-pulmonary substructures (CPSs) associated with overall survival (OS) in locally advanced non-small cell lung cancer (NSCLC) radiotherapy.Materials/Methods
A total of 379 NSCLC patients enrolled in RTOG 0617 were randomly split into training/validation subsets (n=230/149). A deep learning autosegmentation model was used for segmenting 12 CPSs: heart, atria, ventricles, aorta, left ventricle/atrium (LV/LA), right ventricle/atrium (RV/RA), inferior/superior vena cava, pulmonary artery, and pericardium. Contours of peri (=pericardium-heart), rest [=heart-(LV|RV|LA|RA)], clinical target volume and lungs were also obtained. From each structure, dose-volume histogram (DVH) features including min/mean dose to the hottest x% volume (Dx%[Gy]/MOHx%[Gy]; x was 5-100 in 5% increments) and min/mean/max dose as well as percent volume receiving at least xGy (VxGy[%]; x was 5-75 in 5Gy increments) were extracted. Unsupervised multivariate multiblock regression was used to select DVH features in each structure that were canonically correlated with 38 clinical/dosimetric/outcome variables taken from the NTCN/NCORP data archive for RTOG 0617. Additional feature selection for OS analysis was performed using stability selection supervised principal component analysis and Boruta. Algorithm for survival stacking was implemented for training EBM by casting survival analysis as a classification problem. Discrimination performance of EBM for OS in the validation set was evaluated by Harrell's c-index and compared to that of Cox proportional hazards (CPH) and 3 BB models: random survival forest (RSF), deep neural network (DeepSurv) and extreme gradient boosting (XGB). Shapley additive explanations (SHAP) were used to explain their predictions.Results
Selected features included RA_V55Gy[%], aorta_V15Gy[%], LV_D5%[Gy] and pericardium_D20%[Gy]. EBM and all 3 BB models ranked LV_D5%[Gy] as the most important feature, while CPH model ranked second. EBM achieved the best performance for predicting OS, followed by DeepSurv, XGB, RSF and CPH (c-index=0.657, 0.646, 0.643, 0.632 and 0.630). Global explanations of EBM suggested that LV_D5%[Gy]<17.2Gy, pericardium_D20%[Gy]<52.6Gy, RA_V55Gy[%]<12.9% and aorta_V15Gy[%]<49.4% for improved OS. Although SHAP values of CPH and all 3 BB models showed similar trends, they were unable to assist in the precise selection of DVCs.Conclusion
EBM can be used for modeling OS while also providing guidance on CPS DVC selection for optimal cardiac toxicity management in lung cancer radiotherapy.Translated text
Key words
Predictive Modeling,Tumor Staging
AI Read Science
Must-Reading Tree
Example
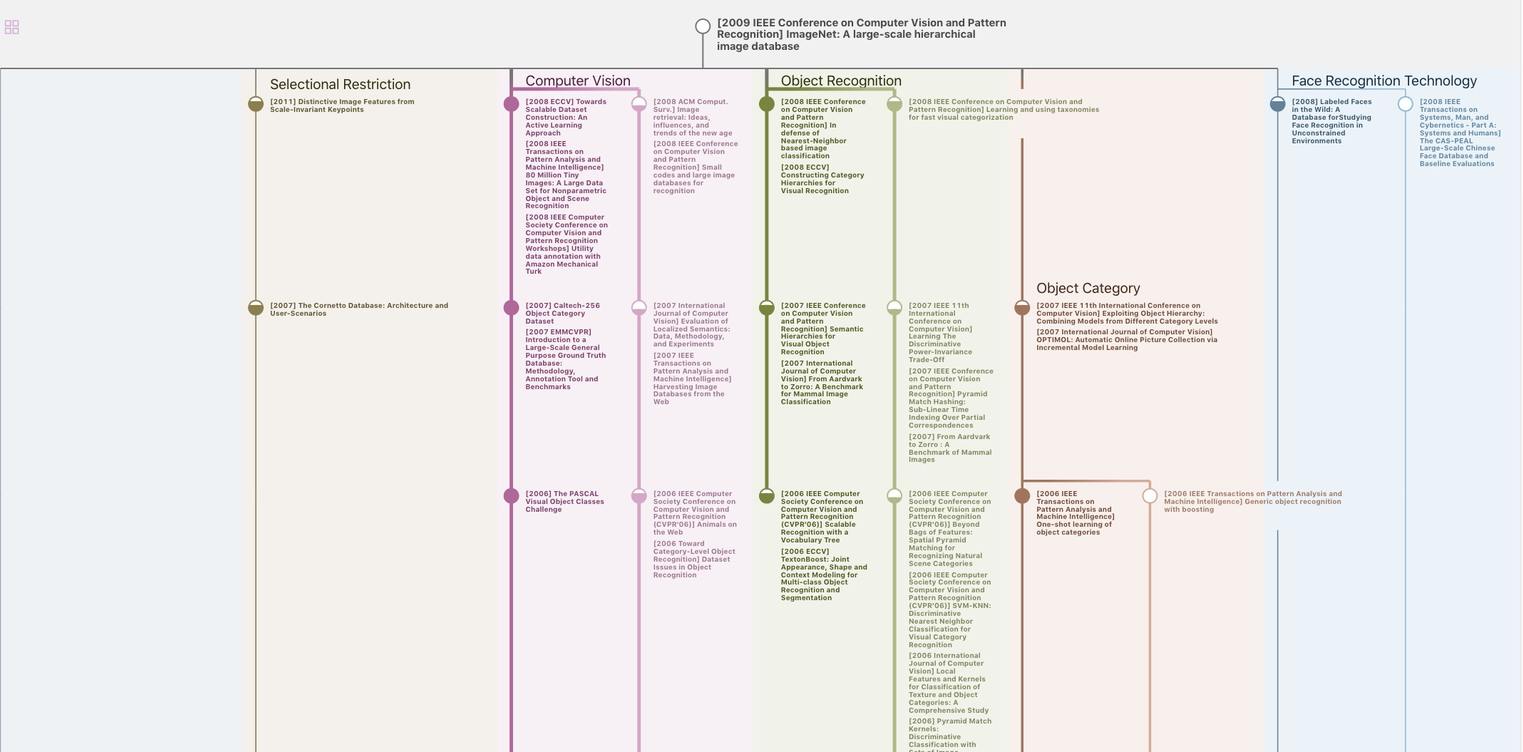
Generate MRT to find the research sequence of this paper
Chat Paper
Summary is being generated by the instructions you defined