Study of the energy deposition of helicon plasmas driven by machine learning algorithms
Contributions to Plasma Physics(2023)
摘要
To find a fast and reliable way predicting the energy deposition of helicon plasmas, this work focuses on machine learning algorithms. Data generation model and the distribution property of the source data are studied, then the classical algorithms and deep neural network (DNN) are built, and these algorithms are studied to test the performance on the energy deposition datasets. Both decision tree classifier (DTC) and support vector machine (SVM) find the electron temperature is the noise feature, and when it is included in the feature vector, the performance will degrade. Therefore feature selection needs be done to obtain high accuracy. For DNN, by directly changing the numbers of the hidden layers and units, test accuracy exceeds 0.95 when hidden layer is greater than three. The feature selection is automatically performed and the learning process is simpler in DNN. When the splitting ratio is varied, the generalization performance of DTC and SVM fluctuates, whereas DNN exhibits no evident change. Compared with the classical algorithms, DNN shows better stability when the source data are changed. The calculations suggest machine learning technique is a promising choice to predict the energy deposition of helicon plasmas.
更多查看译文
关键词
classical algorithms,deep neutral network,energy deposition,helicon plasmas,machine learning
AI 理解论文
溯源树
样例
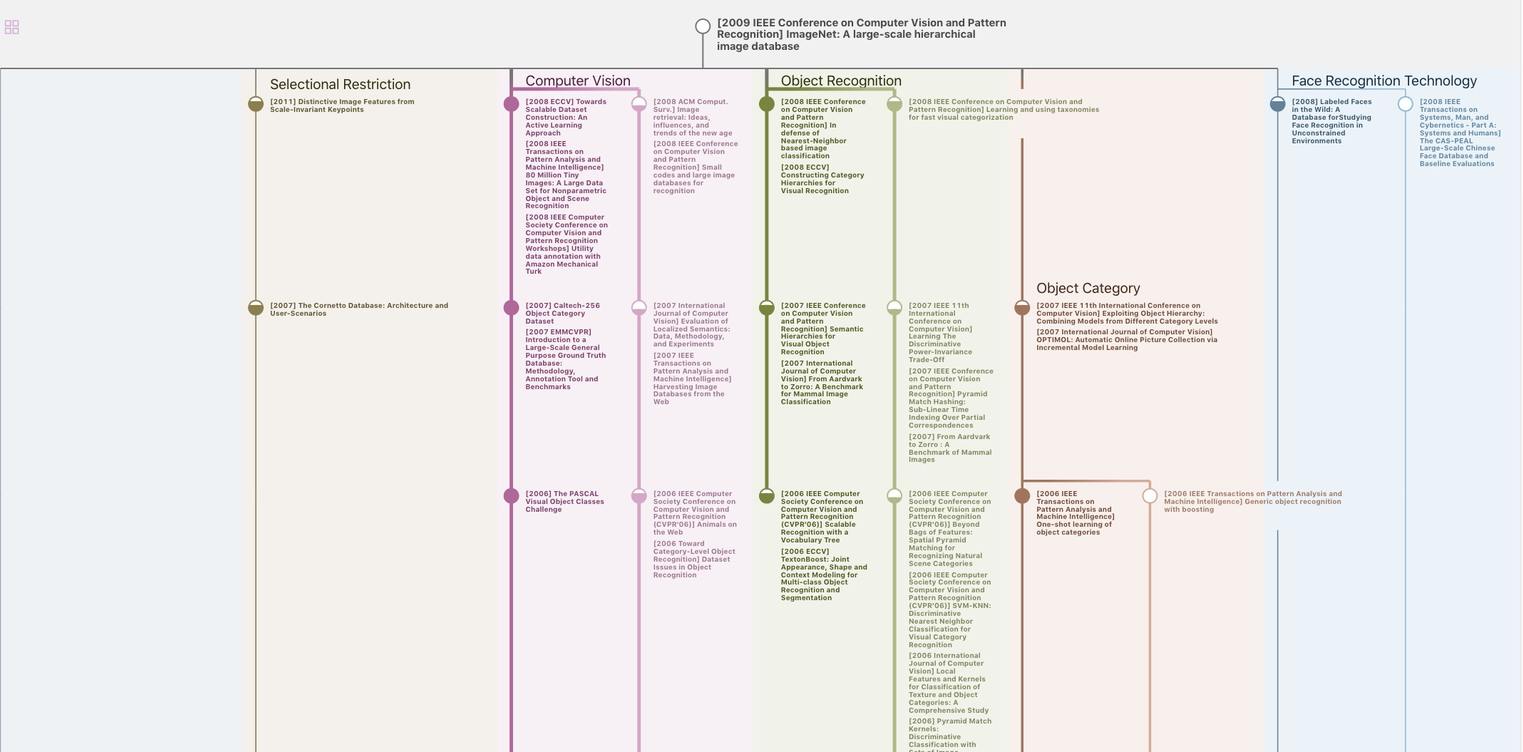
生成溯源树,研究论文发展脉络
Chat Paper
正在生成论文摘要