Lightweight and Highly Robust Memristor-based Hybrid Neural Networks for EEG Signal Processing
Chinese Physics B(2022)
摘要
Abstract Memristor-based neuromorphic computing shows great potential for high speed and high throughput signal processing applications, such as electroencephalogram (EEG) signal processing. Nonetheless, the size of 1T1R memristors arrays is limited by the non-ideality of the devices, which prevents the hardware implementation of large and complex networks. In this work, we propose the Depthwise Separable Convolution and Bidirectional Gate Recurrent Unit (DSC-BiGRU) Network, a lightweight and highly robust hybrid neural network based on 1T1R arrays, which enables efficient processing of EEG signals in the temporal, frequency and spatial domains by hybridizing DSC and BiGRU blocks. The network size is reduced and network robustness is improved while ensuring network classification accuracy. In the simulation, the measured non-idealities of the 1T1R array are brought into the network through statistical analysis. Compared to traditional convolutional networks, the network parameters are reduced by 95% and the network classification accuracy is improved by 21% at 95% array yield rate and 5% tolerable error. This work demonstrates that lightweight and highly robust networks based on memristor arrays hold great promise for applications that rely on low consumption and high efficiency.
更多查看译文
AI 理解论文
溯源树
样例
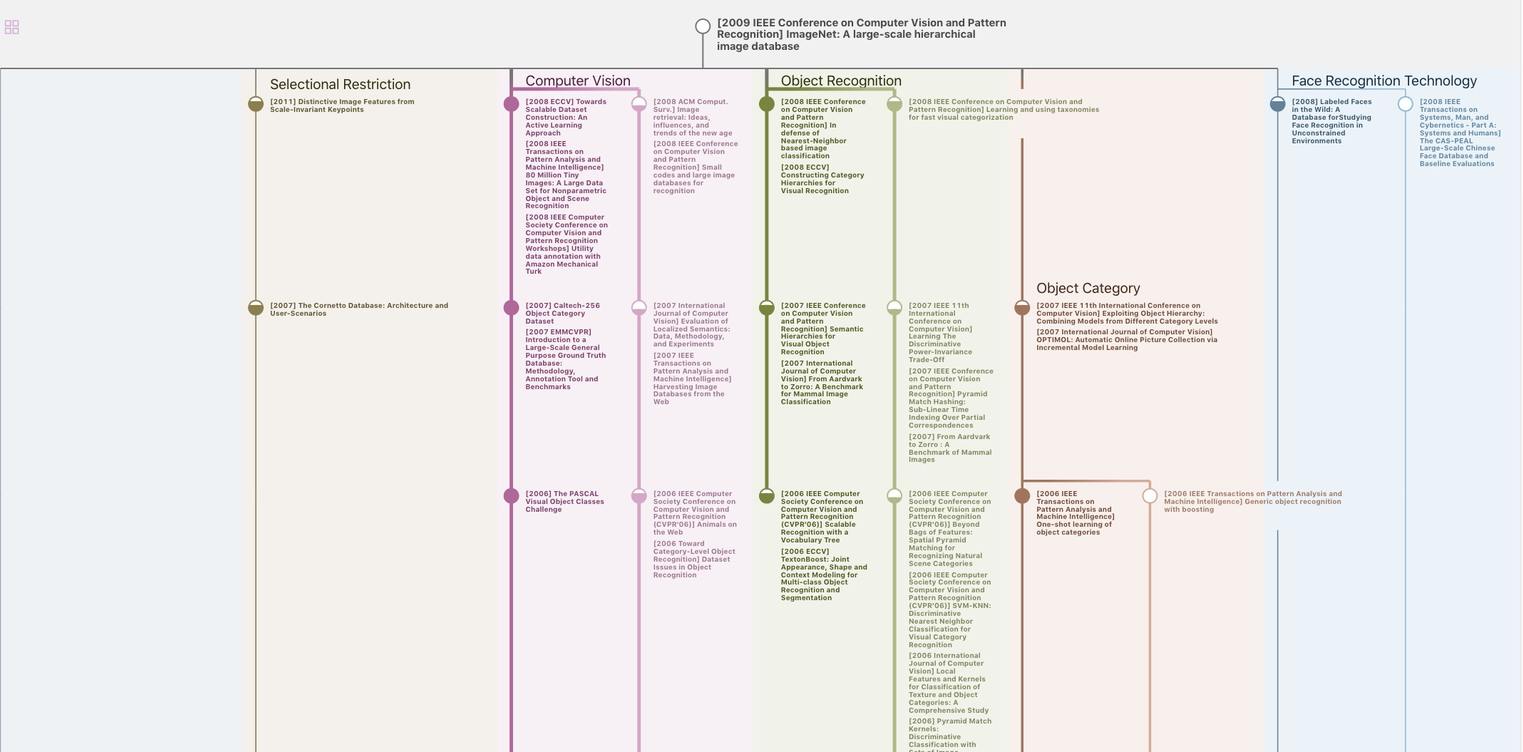
生成溯源树,研究论文发展脉络
Chat Paper
正在生成论文摘要