Long-Term Adaptation of Closed-Loop Glucose Regulation Via Reinforcement Learning Tools
IFAC PAPERSONLINE(2022)
摘要
In recent years, closed-loop controllers for glucose regulation, also called Artificial Pancreas (AP) systems, have become an emblematic problem in the field of automatic control. Several closed-loop systems are in development around the world, such is the case of the Automatic Regulation of Glucose (ARG) algorithm developed in Argentina that has already been tested in the first AP clinical trials for all Latin America. Due to the complexity of the problem at hand, the design and evaluation of controllers for glucose regulation is mostly centered around short-term performance, especially focusing on postprandial periods. However, as everybody, people with diabetes undergo changes in their routines or physiology that can result in an inadequate performance if the controller is not adapted correctly. In this work, the potential of Reinforcement Learning (RL) tools for long-term adaptation of the ARG controller is evaluated through a discrete Q-learning agent. The proposed strategy is evaluated in-silico using the UVA simulator, modifying only one parameter of the controller: its Insulin-On-Board maximum limit. Results show that RL successfully adapts the controller avoiding hypoglycemia when the subjects physiology changes through time, and that the trained agent outperforms a rule-based decision making scheme for the majority of the adult population. Copyright (C) 2022 The Authors.
更多查看译文
关键词
Diabetes Mellitus, Closed-Loop, Reinforcement Learning
AI 理解论文
溯源树
样例
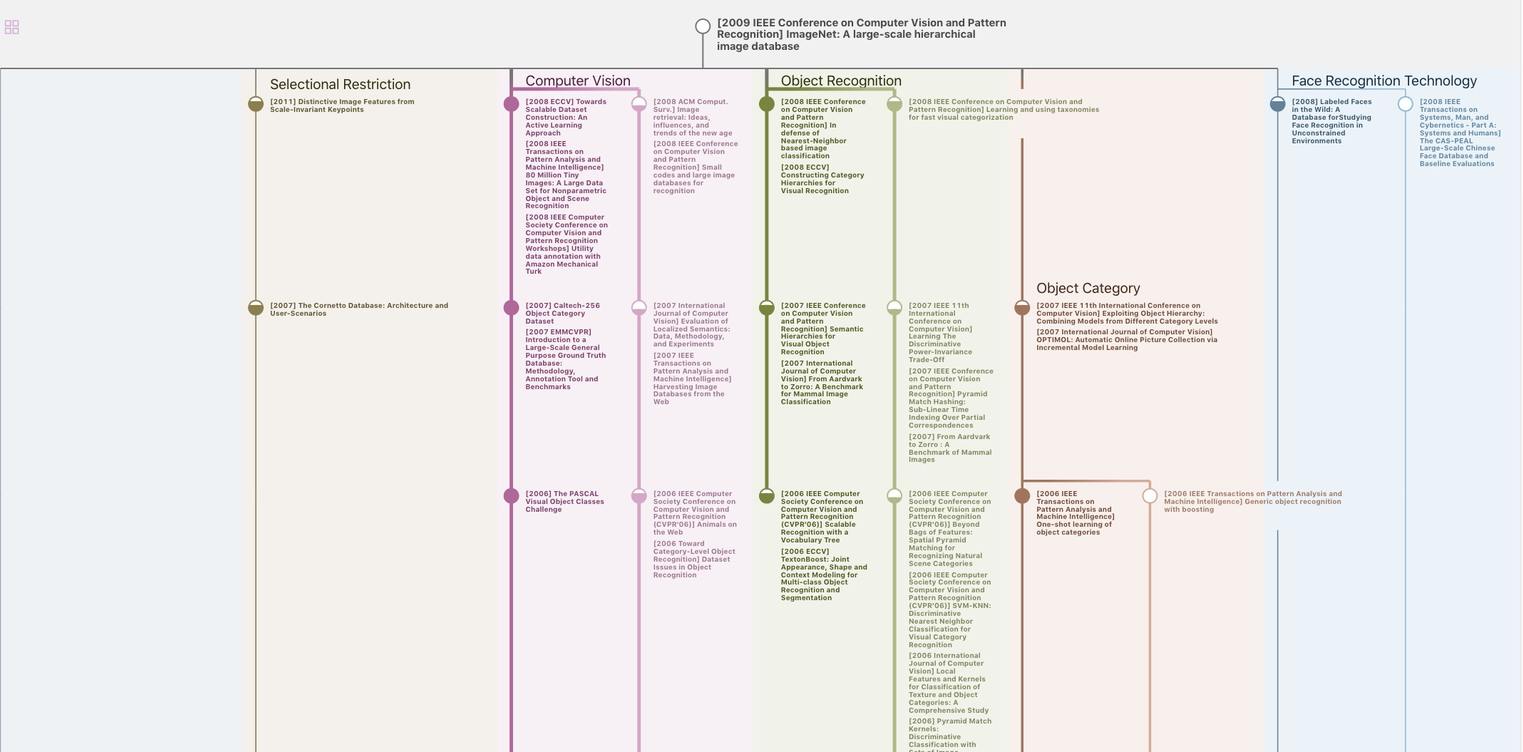
生成溯源树,研究论文发展脉络
Chat Paper
正在生成论文摘要