A Novel Data-Driven Method for Medium-Term Power Consumption Forecasting Based on Transformer-LightGBM
MOBILE INFORMATION SYSTEMS(2022)
摘要
With the widespread use of new energy sources and Internet of things, the power market landscape has become complex. In particular, new energy is more stochastic and volatile; it is prone to the problems of inaccurate forecasting on longer time scales, affecting electricity trading. This study proposes a new method for predicting medium-term load series data based on the transformer-lightGBM. The method first preprocesses electricity market data, including missing value processing, outlier processing, overall analysis, and correlation analysis, to extract features with a strong correlation to medium-term electricity consumption forecasts. Then, a transformer neural network is used to learn the complex patterns and dynamic time scales of the load series data to predict the day-ahead market series. Finally, lightGBM is used to combine power characteristics and time characteristics to forecast power consumption. The effectiveness of the proposed method is proved using the ISO-NE dataset. Experimental results indicate that the present method verified more accurate prediction than LSTM-based methods.
更多查看译文
关键词
forecasting,data-driven,medium-term,transformer-lightgbm
AI 理解论文
溯源树
样例
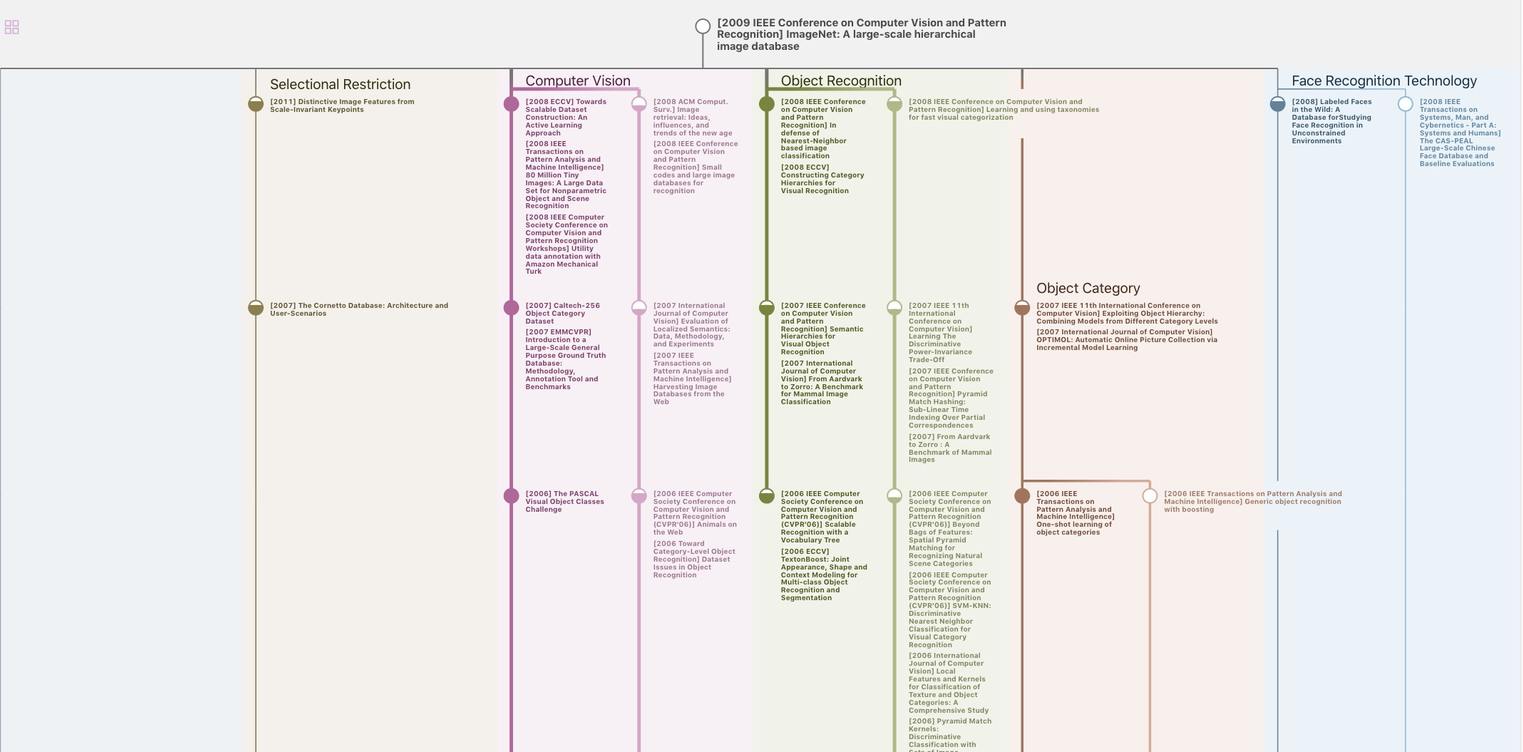
生成溯源树,研究论文发展脉络
Chat Paper
正在生成论文摘要