Input-to-state Stabilization of Delayed Semi-Markovian Jump Neural Networks Via Sampled-Data Control
NEURAL PROCESSING LETTERS(2022)
Abstract
This paper discusses the sampled-data input-to-state stabilization for delayed semi-Markovian jump neural networks subject to external disturbance. First, a hybrid closed-loop system is formulated, which contains continuous-time state signal, disturbance input signal, discrete-time control signal, and jumping parameters of the semi-Markovian process. Then, two time-dependent and mode-dependent Lyapunov functionals are constructed corresponding to different assumptions about the activation functions. Subsequently, two sufficient conditions concerning the sampled-data controller design are derived to ensure the mean-square input-to-state stability for the hybrid closed-loop system by utilizing the proposed Lyapunov functionals, a few inequalities, as well as some stochastic analysis techniques. It is worth remarking that the present conditions are capable of ensuring mean-square exponential stability of the closed-loop system in the absence of external disturbances. Lastly, a numerical example is employed to verify the validity of the proposed input-to-state stabilization methods.
MoreTranslated text
Key words
Input-to-state stability, Stabilization, Semi-Markovian process, Neural network, Sampled-data control
AI Read Science
Must-Reading Tree
Example
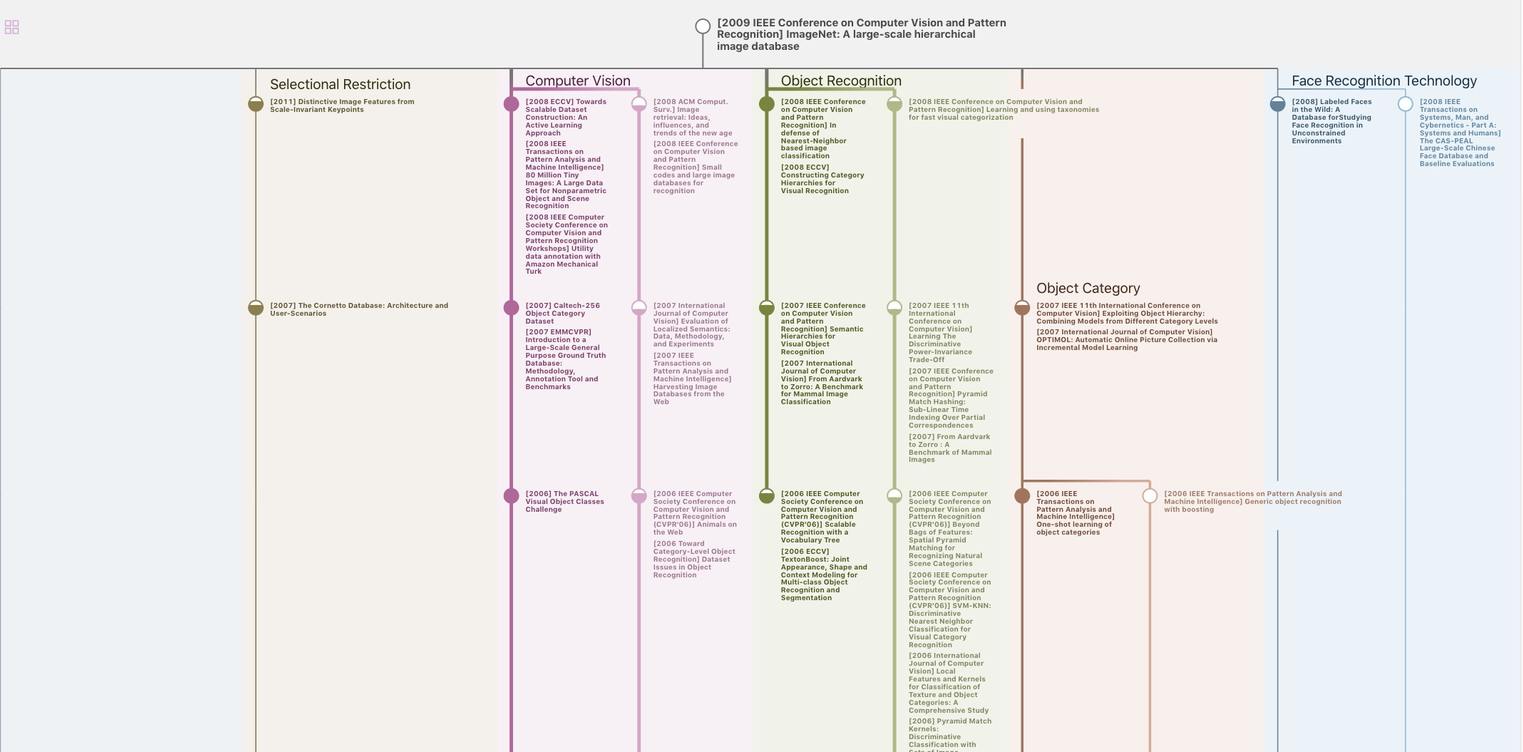
Generate MRT to find the research sequence of this paper
Chat Paper
Summary is being generated by the instructions you defined