Efficient Pruning-Split LSTM Machine Learning Algorithm for Terrestrial-Satellite Edge Network
2022 IEEE INTERNATIONAL CONFERENCE ON COMMUNICATIONS WORKSHOPS (ICC WORKSHOPS)(2022)
摘要
The recent advances in low earth orbit (LEO) satellite-borne edge cloud (SEC) enable resource-limited users to access edge servers via a terrestrial station terminal (TST) for rapid task processing capability. However, the dynamic variation in the TST transmit power challenges the served users to develop optimal computing task processing decisions. In this paper, we propose an efficient pruning-split long short-term memory (LSTM) learning algorithm to address this challenge. First, we present an LSTM algorithm for TST transmit power prediction. The proposed algorithm is then pruned and split to decrease the computing workload and the communication resource consumption considering the limited computing resource of TSTs and served users' quality of service (QoS). Finally, an algorithm split layer selection method is introduced based on the real-time situation of the TST. The simulation results are shown to verify the effectiveness of the proposed pruning-split LSTM algorithm.
更多查看译文
关键词
efficient pruning-split LSTM machine learning algorithm,pruning-split long short-term memory learning algorithm,terrestrial-satellite edge network,pruning-split LSTM algorithm,algorithm split layer selection method,computing resource,communication resource consumption,computing workload,TST transmit power prediction,optimal computing task processing decisions,served users,rapid task processing capability,terrestrial station terminal,edge servers,resource-limited users,low earth orbit satellite-borne edge cloud
AI 理解论文
溯源树
样例
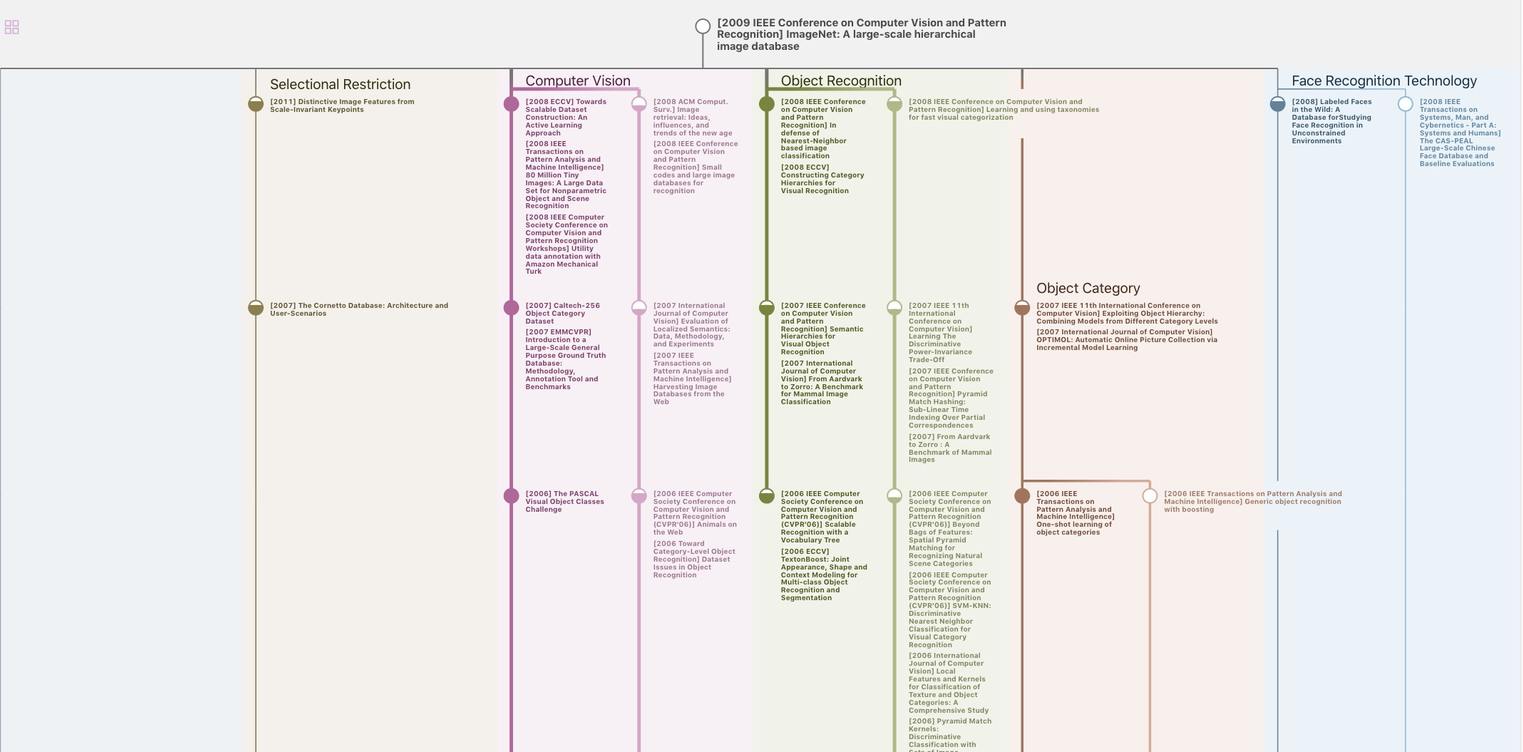
生成溯源树,研究论文发展脉络
Chat Paper
正在生成论文摘要