Wrapped Particle Filtering for Angular Data
IEEE ACCESS(2022)
摘要
Particle filtering is probably the most widely accepted methodology for general nonlinear filtering applications. The performance of a particle filter critically depends on the choice of proposal distribution. In this paper, we propose using a wrapped normal distribution as a proposal distribution for angular data, i.e. data within finite range (-pi, pi] . We then use the same method to derive the proposal density for a particle filter, in place of a standard assumed Gaussian density filter such as the unscented Kalman filter. The numerical integrals with respect to wrapped normal distribution are evaluated using Rogers-Szego quadrature. Compared to using the unscented filter and similar approximate Gaussian filters to produce proposal densities, we show through examples that wrapped normal distribution gives a far better filtering performance when working with angular data. In addition, we demonstrate the trade-off involved in particle filters with local sampling and global sampling (i.e. by running a bank of approximate Gaussian filters vs running a single approximate Gaussian filter) with the former yielding a better filtering performance than the latter at the cost of increased computational load.
更多查看译文
关键词
Nonlinear dynamical systems, angular data, particle filtering, wrapped normal distribution, Rogers-Szego quadrature rule
AI 理解论文
溯源树
样例
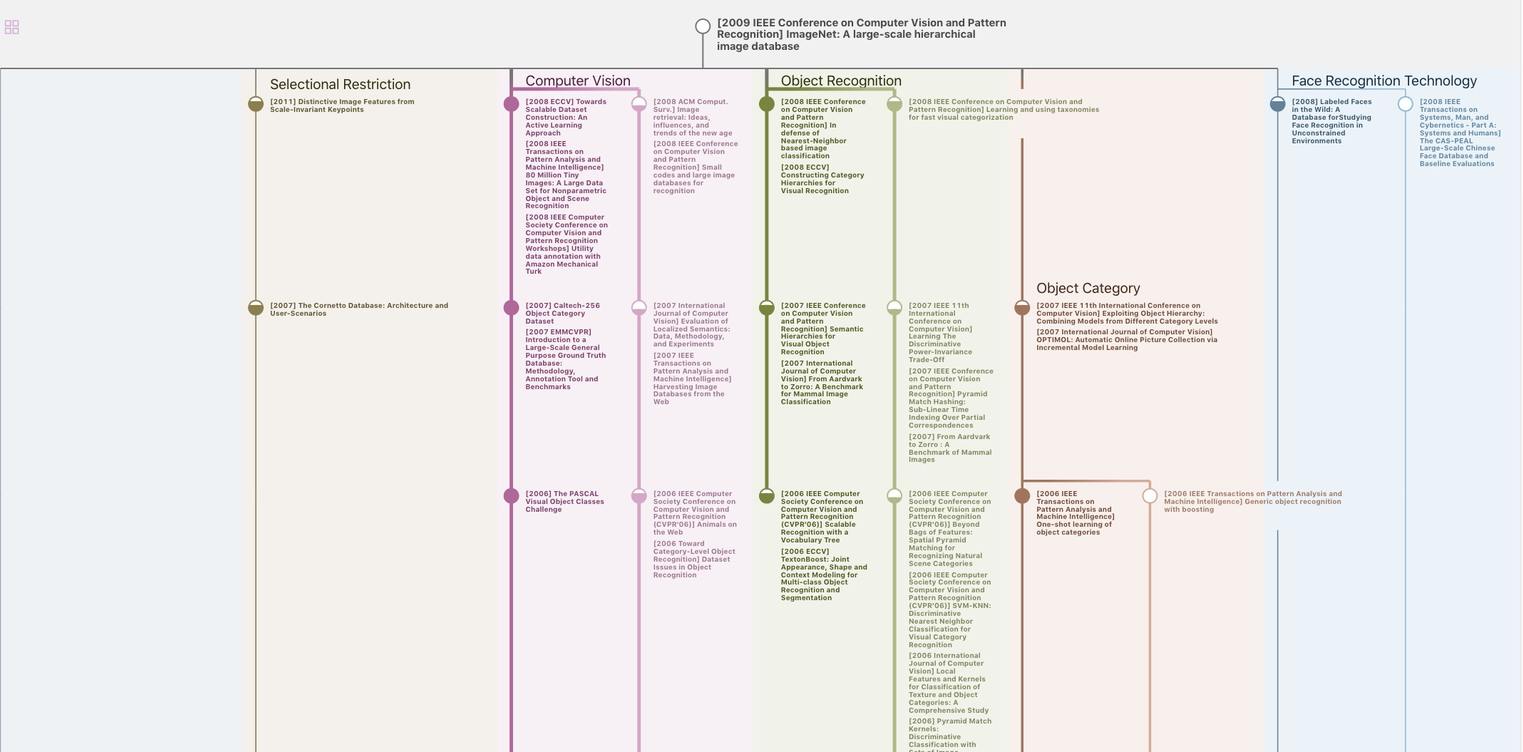
生成溯源树,研究论文发展脉络
Chat Paper
正在生成论文摘要