Parameter-Free Similarity-Aware Attention Module for Medical Image Classification and Segmentation
IEEE TRANSACTIONS ON EMERGING TOPICS IN COMPUTATIONAL INTELLIGENCE(2023)
摘要
Automatic classification and segmentation of medical images play essential roles in computer-aided diagnosis. Deep convolutional neural networks (DCNNs) have shown their advantages on image classification and segmentation. However, they have not achieved the same success on medical images as they have done on natural images. In this paper, two challenges are exploited for DCNNs on medical images, including 1) lack of feature diversity; 2) neglect of small lesions. These two issues heavily influence the classification and segmentation performances. To improve the performance of DCNN on medical images, similarity-aware attention (simi-attention) module is proposed, including a Feature-similarity-aware Channel Attention (FCA) and a Region-similarity-aware Spatial Attention (RSA). Our simi-attention provides three advantages: 1) higher accuracy can be achieved since it extracts both diverse and discriminant features from medical images via our FCA and RSA; 2) the lesions can be exactly focused and located by it even for the data with low intensity contrast and small lesions; 3) it does not increase the complexity of backbone models due to NO trainable parameters in its module. The experimental results are conducted on both classification and segmentation tasks under four public medical classification datasets and two public medical segmentation datasets. The visualization results show that our simi-attention can accurately focuses on the lesions for classification and generate fine segmentation results even for small objects. The overall performances show that our simi-attention can significantly improve the performances of backbone models and outperforms compared attention models on most of datasets for both classification and segmentation.
更多查看译文
关键词
Feature extraction, Lesions, Image segmentation, Medical diagnostic imaging, Convolution, Task analysis, Representation learning, Medical image classification, medical image segmentation, parameter-free, similarity-aware attention module
AI 理解论文
溯源树
样例
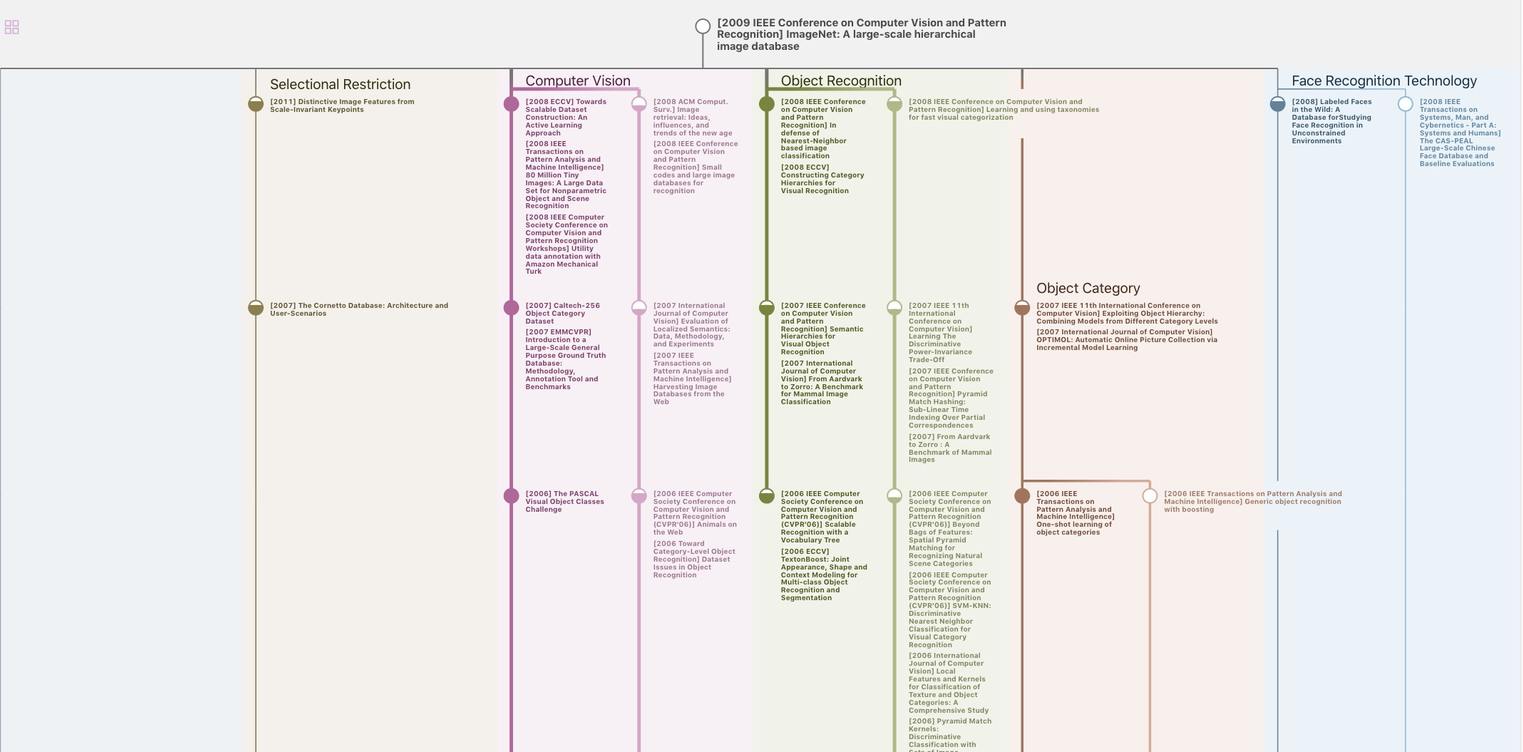
生成溯源树,研究论文发展脉络
Chat Paper
正在生成论文摘要